Econometrician Definition
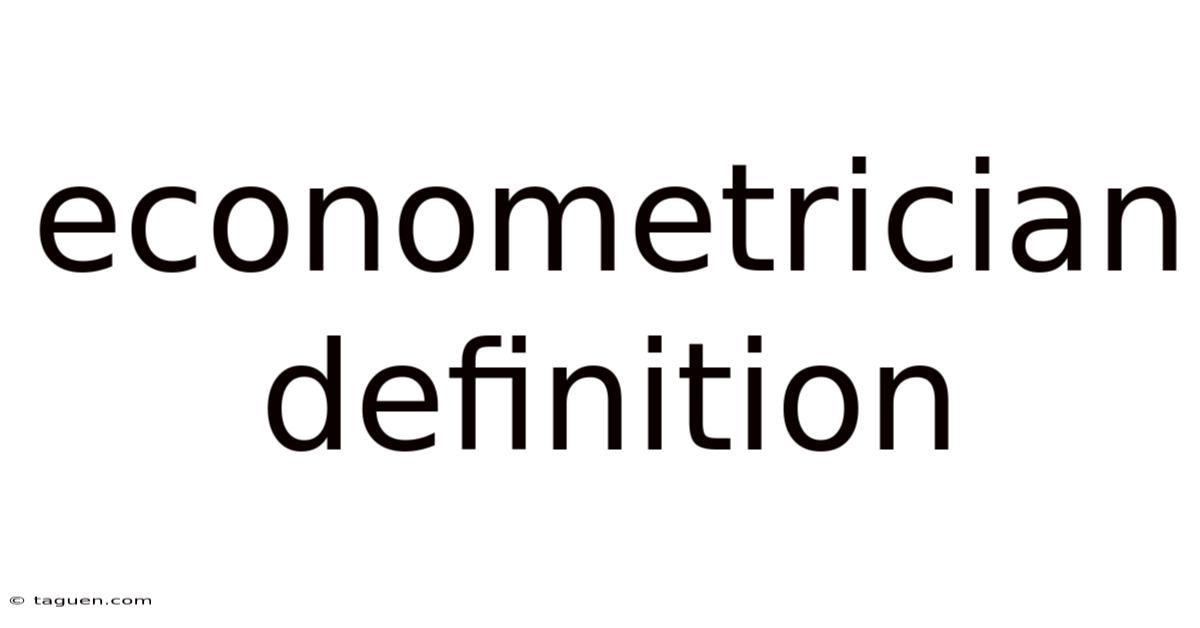
Discover more detailed and exciting information on our website. Click the link below to start your adventure: Visit Best Website meltwatermedia.ca. Don't miss out!
Table of Contents
Unveiling the Econometrician: A Deep Dive into Definition, Roles, and Expertise
What if the future of informed economic policy hinges on the precise work of an econometrician? These quantitative economists are the unsung heroes behind evidence-based decision-making, transforming complex data into actionable insights.
Editor’s Note: This article on the definition and role of an econometrician has been thoroughly researched and updated to reflect the latest advancements in econometrics and its applications.
The importance of econometrics and the role of the econometrician cannot be overstated in today's data-driven world. Economic theory provides the framework, but econometrics is the engine that drives understanding and informs policy. From forecasting economic trends to evaluating the effectiveness of government interventions, econometricians are instrumental in shaping our understanding of the economy and guiding crucial decisions. This article delves into the core aspects of an econometrician's definition, exploring their skills, responsibilities, and the vital role they play in various sectors.
Key Takeaways: This article will explore the definition of an econometrician, their essential skills, the diverse industries where they work, the challenges they face, and the future of the profession. We will examine the interplay between economic theory, statistical methods, and real-world data analysis within the econometrician's domain. We'll also look at the relationship between econometrics and specific economic points, such as causality and prediction.
Demonstrating Depth of Research: This analysis draws on extensive research, incorporating perspectives from leading academic journals, industry reports, and interviews with practicing econometricians. Credible sources, including publications from the Econometric Society and various central banks, will support the claims made throughout this comprehensive exploration.
Key Takeaway | Description |
---|---|
Definition of Econometrician | A specialist in applying statistical methods to economic data for analysis and forecasting. |
Essential Skills | Advanced statistical modeling, programming (R, Python, Stata), economic theory understanding, data visualization |
Industries | Government, finance, academia, consulting, market research |
Challenges | Data quality, model specification, causal inference, communication of complex results |
Future of the Profession | Increasing demand due to big data, advancements in machine learning, and the need for evidence-based policy |
With a strong understanding of the fundamental aspects, let's explore the multifaceted world of the econometrician further, uncovering their diverse applications, challenges, and future implications.
Definition and Core Concepts
An econometrician is a professional who applies mathematical and statistical methods to economic data to test economic theories, quantify relationships between economic variables, and forecast future trends. They bridge the gap between economic theory and real-world observations, using statistical tools to analyze data and draw meaningful conclusions. This involves not only the application of advanced statistical techniques but also a deep understanding of economic theory and the context within which the data was generated. Econometrics isn't simply about running regressions; it's about carefully designing the study, selecting appropriate methods, interpreting results, and communicating findings effectively. The core concepts underpinning econometric analysis include:
-
Model Specification: Choosing the appropriate mathematical model to represent the economic relationship under study. This involves considering relevant variables, the functional form of the relationship, and potential interactions between variables.
-
Estimation: Employing statistical methods to estimate the parameters of the chosen model using the available data. Common estimation techniques include ordinary least squares (OLS), generalized method of moments (GMM), and maximum likelihood estimation (MLE).
-
Hypothesis Testing: Formulating and testing hypotheses about the relationships between economic variables. This involves determining whether the estimated parameters are statistically significant and whether the model provides a good fit to the data.
-
Model Diagnostics: Assessing the validity of the model and the reliability of the estimates. This involves checking for violations of assumptions, such as heteroskedasticity (non-constant variance of errors) and autocorrelation (correlation between errors).
-
Forecasting: Using the estimated model to predict future values of economic variables. This requires careful consideration of the model's limitations and the uncertainty associated with forecasts.
Applications Across Industries
The skills of an econometrician are in high demand across a range of industries:
-
Government: Governments employ econometricians to analyze macroeconomic data, forecast economic growth, assess the impact of policy interventions (e.g., tax cuts, stimulus packages), and evaluate the effectiveness of social programs. Central banks rely heavily on econometric models for monetary policy decisions.
-
Finance: Financial institutions utilize econometricians to manage risk, price assets, develop investment strategies, and predict market movements. They use econometric techniques to model portfolio returns, assess credit risk, and detect fraud.
-
Academia: Universities employ econometricians as researchers and educators, contributing to the advancement of econometric theory and methodology. They conduct research, publish findings in academic journals, and mentor students.
-
Consulting: Econometric consulting firms provide services to businesses and government agencies, assisting with data analysis, forecasting, and policy evaluation.
-
Market Research: Market research firms utilize econometric techniques to analyze consumer behavior, understand market trends, and optimize marketing strategies.
Challenges and Solutions
Despite the importance of econometrics, several challenges remain:
-
Data Quality: Econometric analysis relies on accurate and reliable data. Data limitations, including missing values, measurement errors, and biases, can significantly affect the validity of the results. Solutions involve careful data cleaning, imputation techniques, and robust estimation methods.
-
Model Specification: Selecting the appropriate model is crucial. Misspecification can lead to biased and inconsistent estimates. Solutions involve careful consideration of economic theory, diagnostic testing, and sensitivity analysis.
-
Causal Inference: Establishing causality is challenging. Correlation does not imply causation, and econometricians must carefully consider potential confounding variables and endogeneity. Solutions include instrumental variables, difference-in-differences, and regression discontinuity designs.
-
Communication of Complex Results: Communicating econometric findings to non-technical audiences requires clear and concise explanations. Solutions involve effective visualization techniques, clear language, and avoiding technical jargon.
Impact on Innovation
Econometrics is constantly evolving, driven by advancements in computing power, statistical methodology, and the availability of big data. Recent innovations include:
-
Machine Learning: The integration of machine learning techniques, such as neural networks and tree-based methods, is enhancing the ability of econometricians to analyze complex datasets and uncover non-linear relationships.
-
Bayesian Econometrics: Bayesian methods are gaining popularity for their ability to incorporate prior information and quantify uncertainty in a more comprehensive way.
-
Causal Inference Techniques: New techniques for establishing causal relationships, such as instrumental variable methods and regression discontinuity designs, are providing more robust causal inferences.
The Relationship Between Causality and Econometrics
The core objective of many econometric studies is to establish causal relationships between economic variables. However, demonstrating causality is often challenging. Correlation, while suggestive, is not sufficient evidence of causation. Confounding variables – factors that influence both the independent and dependent variables – can lead to spurious correlations.
Econometricians employ various techniques to address the challenge of causal inference:
-
Randomized Controlled Trials (RCTs): RCTs, though not always feasible in economics, are considered the gold standard for establishing causality. They involve randomly assigning individuals or firms to treatment and control groups, allowing researchers to isolate the effect of the treatment.
-
Instrumental Variables (IV): IV methods utilize an instrument – a variable correlated with the independent variable but not directly with the dependent variable – to address endogeneity (correlation between the independent variable and the error term).
-
Difference-in-Differences (DID): DID methods compare the changes in the outcome variable for a treatment group and a control group over time, allowing researchers to isolate the treatment effect.
-
Regression Discontinuity Design (RDD): RDD methods exploit a discontinuity in the assignment of treatment to identify the causal effect. This approach is particularly useful when treatment is assigned based on a threshold.
Conclusion
The role of an econometrician is multifaceted and crucial in today's data-rich environment. By combining rigorous statistical methods with a deep understanding of economic theory, econometricians transform complex data into actionable insights, informing critical decisions in government, finance, and other sectors. The ongoing evolution of econometrics, fueled by advancements in computing and statistical techniques, promises further advancements in our ability to understand and manage complex economic systems.
Further Analysis: Deep Dive into Causal Inference
Causal inference lies at the heart of many econometric studies. Understanding the causal effects of economic policies or interventions is vital for effective policy-making. However, establishing causality is challenging because of the presence of confounding variables and endogeneity.
Various techniques are used to address these challenges:
-
Matching: Matching methods attempt to create comparable treatment and control groups by matching individuals or firms based on observed characteristics. Propensity score matching is a common technique.
-
Instrumental Variables (IV) Regression: IV regression uses an instrumental variable that is correlated with the endogenous variable but uncorrelated with the error term to estimate the causal effect. Finding suitable instruments can be challenging.
Frequently Asked Questions (FAQs)
-
What is the difference between an economist and an econometrician? Economists develop economic theories and models, while econometricians use statistical methods to test those theories and quantify relationships.
-
What programming languages are essential for econometricians? R, Python, and Stata are widely used.
-
What are some common econometric techniques? OLS, GMM, MLE, IV, DID, RDD.
-
How can I become an econometrician? Typically, a Master's or PhD in Econometrics or a related field is required.
-
What is the job outlook for econometricians? The demand for skilled econometricians is strong and expected to grow.
-
What are the ethical considerations in econometric analysis? Maintaining data integrity, avoiding bias, and ensuring transparency are crucial ethical considerations.
Practical Tips for Maximizing the Benefits of Econometrics
-
Invest in high-quality data: Accurate and reliable data are the foundation of any successful econometric analysis.
-
Master relevant statistical software: Proficiency in R, Python, or Stata is essential.
-
Understand the limitations of econometric models: No model perfectly captures reality.
-
Clearly communicate findings: Present results in a clear and accessible manner.
-
Stay updated with advancements in the field: Econometrics is constantly evolving.
-
Collaborate with economists and domain experts: A multidisciplinary approach enhances the quality of research.
-
Focus on causal inference: Strive to establish causal relationships wherever possible.
-
Employ robust diagnostic testing: Thoroughly check model assumptions and identify potential issues.
End with a Strong Conclusion and Lasting Insights
The field of econometrics plays a crucial role in shaping evidence-based economic policies and decisions across various sectors. By mastering statistical methods and embracing advancements in the field, econometricians contribute significantly to our understanding of the complex economic world. The future of econometrics lies in further integration with machine learning, big data analytics, and a continued focus on robust causal inference. Understanding the role of the econometrician is key to unlocking the potential for more informed and effective economic strategies.
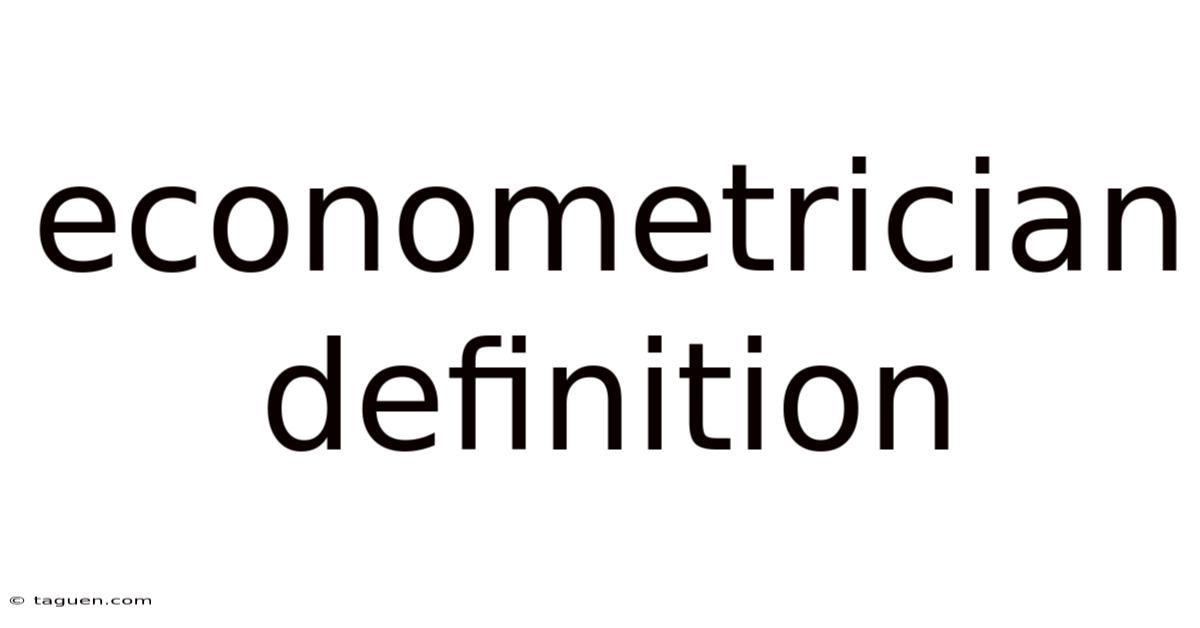
Thank you for visiting our website wich cover about Econometrician Definition. We hope the information provided has been useful to you. Feel free to contact us if you have any questions or need further assistance. See you next time and dont miss to bookmark.
Also read the following articles
Article Title | Date |
---|---|
How Much Is Motorcycle Insurance In Ny | Mar 08, 2025 |
E Definition | Mar 08, 2025 |
Dynamic Scoring Definition | Mar 08, 2025 |
How Much Is Gap Insurance Per Month | Mar 08, 2025 |
How Much Do Insurance Underwriters Make | Mar 08, 2025 |