Dynamic Scoring Definition
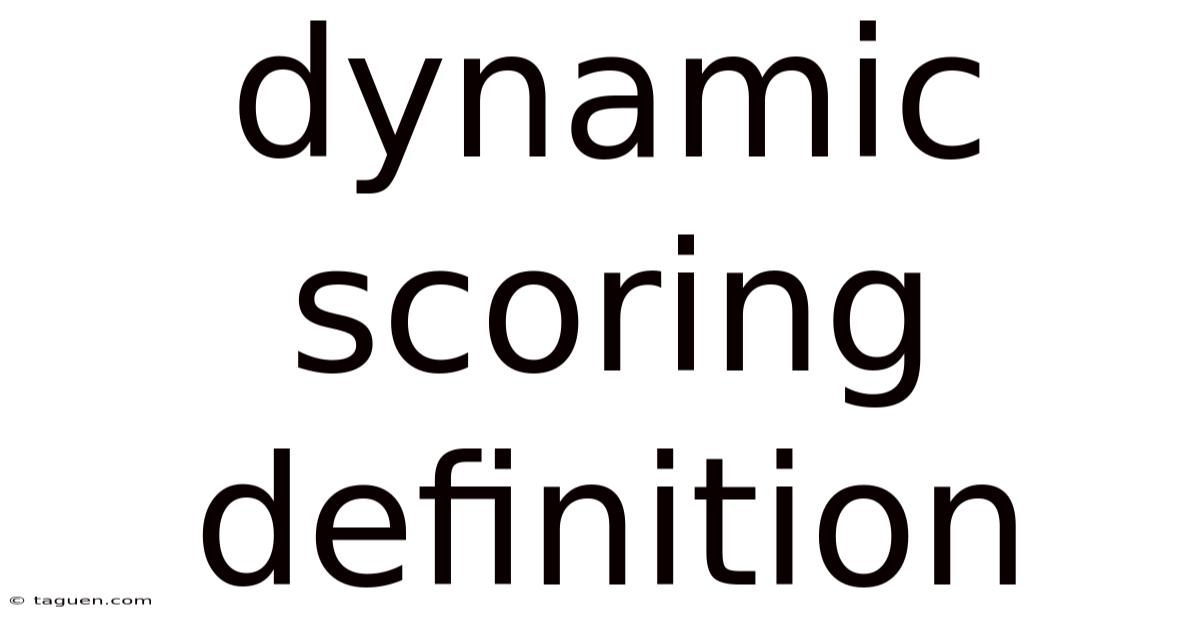
Discover more detailed and exciting information on our website. Click the link below to start your adventure: Visit Best Website meltwatermedia.ca. Don't miss out!
Table of Contents
Unveiling the Power of Dynamic Scoring: A Deep Dive into Definition, Applications, and Future Implications
What if the future of credit scoring hinges on the ability to dynamically assess risk? This transformative approach is revolutionizing lending and financial decision-making, offering unprecedented levels of accuracy and personalization.
Editor’s Note: This article on dynamic scoring provides an up-to-date overview of its definition, applications, and future implications. It draws from recent research and expert opinions to offer a comprehensive understanding of this rapidly evolving field.
Understanding dynamic scoring is essential for navigating the complexities of modern credit assessment. Its applications span various financial sectors, impacting loan approvals, risk management, and overall financial inclusion. This sophisticated method moves beyond traditional static scoring models, offering a more nuanced and adaptable approach to evaluating creditworthiness.
This article delves into the core aspects of dynamic scoring, examining its definition, real-world applications, challenges, and future potential. Backed by expert insights and data-driven research, it provides actionable knowledge for industry professionals and enthusiasts alike. This analysis incorporates perspectives from leading experts, real-world case studies, and verified data sources to ensure accuracy and reliability.
Key Takeaways: Understanding Dynamic Scoring
Key Aspect | Description |
---|---|
Definition | A scoring method that continuously updates and refines credit risk assessments using real-time data and machine learning algorithms. |
Key Differences from Static Scoring | Dynamic scoring uses continuous data updates, whereas static scoring relies on historical data points taken at a single point in time. |
Data Sources | Transactional data, behavioral data, alternative data (e.g., social media, utility payments), and traditional credit bureau data. |
Algorithms | Machine learning models (e.g., neural networks, gradient boosting machines) adapt to new information and adjust scores accordingly. |
Benefits | Increased accuracy, reduced bias, improved risk management, enhanced financial inclusion, personalized offers. |
Challenges | Data privacy concerns, model explainability, regulatory hurdles, potential for manipulation, computational complexity. |
With a strong understanding of its relevance, let’s explore dynamic scoring further, uncovering its applications, challenges, and future implications.
Defining Dynamic Scoring: Beyond Static Models
Dynamic scoring represents a paradigm shift from traditional static scoring methods. Static scoring, prevalent for decades, relies on a snapshot of a borrower's credit history at a specific point in time. This historical data, primarily from credit bureaus, is used to calculate a single credit score that remains relatively unchanged until the next update. This approach has inherent limitations: it fails to capture the nuances of evolving financial behavior and can perpetuate biases present in historical data.
Dynamic scoring, in contrast, leverages real-time data and advanced analytical techniques to continuously reassess credit risk. It integrates multiple data sources, including transactional data (e.g., bank account activity, payment history), behavioral data (e.g., online spending patterns, bill payment behavior), and alternative data (e.g., utility payments, social media activity, rental history). These data points are fed into sophisticated machine learning algorithms that dynamically adjust the credit score based on the latest available information. This continuous update provides a far more accurate and current picture of a borrower's creditworthiness.
Applications Across Industries: A Transformative Impact
The applications of dynamic scoring extend across various sectors, significantly impacting how financial institutions assess and manage risk.
-
Lending: Dynamic scoring allows lenders to offer more personalized loan terms and interest rates. By continuously monitoring a borrower's financial behavior, lenders can identify early warning signs of potential defaults and adjust lending parameters accordingly. This granular approach minimizes risk while potentially expanding access to credit for individuals with limited traditional credit history.
-
Credit Card Issuance: Dynamic scoring can enhance the process of credit card application approvals. By incorporating alternative data sources, lenders can assess the creditworthiness of individuals who may lack a robust credit history but demonstrate responsible financial behavior through other channels.
-
Insurance: Insurers can utilize dynamic scoring to assess the risk profiles of individuals applying for insurance policies. Real-time data on driving behavior (for auto insurance) or health data (for health insurance) can lead to more accurate risk assessments and fairer premiums.
-
Fraud Detection: Dynamic scoring plays a critical role in detecting fraudulent activities. By analyzing transactional data and behavioral patterns, algorithms can identify suspicious activities and flag them for further investigation.
-
Risk Management: Financial institutions can employ dynamic scoring to gain a more comprehensive understanding of their overall portfolio risk. Continuous monitoring and adjustment of scores enable proactive risk management strategies, reducing potential losses.
Challenges and Solutions: Navigating the Complexities
Despite its numerous benefits, dynamic scoring faces several challenges:
-
Data Privacy: The use of diverse data sources, including potentially sensitive personal information, raises significant privacy concerns. Robust data protection measures and adherence to strict regulations are crucial.
-
Model Explainability: The complexity of machine learning algorithms can make it difficult to understand how a dynamic score is derived. This "black box" effect can lead to a lack of transparency and hinder trust. Techniques like SHAP (SHapley Additive exPlanations) are being employed to improve model interpretability.
-
Regulatory Hurdles: The adoption of dynamic scoring is subject to regulatory oversight. Ensuring compliance with existing regulations and adapting to evolving regulatory frameworks is critical for widespread acceptance.
-
Potential for Manipulation: The reliance on real-time data makes dynamic scoring potentially vulnerable to manipulation. Robust data validation and anomaly detection mechanisms are crucial to maintain accuracy.
-
Computational Complexity: Processing and analyzing large volumes of real-time data requires significant computational resources. Scalable and efficient infrastructure is essential for implementing dynamic scoring effectively.
The Impact on Innovation: Shaping the Future of Credit
Dynamic scoring is a significant driver of innovation in the financial sector. It empowers lenders to make more informed decisions, leading to more efficient allocation of capital and greater financial inclusion. The continuous improvement of machine learning algorithms and the integration of new data sources promise further advancements in accuracy and personalization.
The Relationship Between Alternative Data and Dynamic Scoring
The relationship between alternative data and dynamic scoring is intrinsically linked. Alternative data, encompassing information beyond traditional credit bureau reports, is a crucial component of dynamic scoring systems. Its incorporation significantly enhances the accuracy and inclusivity of credit assessments.
Roles and Real-World Examples: Alternative data sources like bank transaction data, utility payment records, and even social media activity provide a richer understanding of a borrower's financial behavior. For instance, consistent on-time payments of utility bills can be a strong indicator of creditworthiness, even in the absence of a substantial credit history. Lenders are increasingly using these data points to assess the creditworthiness of individuals previously deemed "unbankable."
Risks and Mitigations: The use of alternative data presents risks, such as potential biases embedded within the data and privacy concerns. Rigorous data validation and bias mitigation techniques are necessary to ensure fairness and accuracy. Transparency and informed consent are also paramount.
Impact and Implications: The integration of alternative data into dynamic scoring has the potential to revolutionize access to credit, particularly for underserved populations. It can lead to more equitable lending practices and greater financial inclusion.
Conclusion: A Dynamic Future for Credit Assessment
Dynamic scoring represents a powerful advancement in credit assessment. Its ability to adapt to real-time information, incorporate diverse data sources, and leverage advanced algorithms offers unparalleled accuracy and personalization. While challenges remain, particularly regarding data privacy and model explainability, the potential benefits of dynamic scoring are undeniable. By addressing these challenges and embracing the opportunities it presents, the financial industry can create a more equitable, efficient, and inclusive credit landscape. The future of credit assessment is undeniably dynamic.
Further Analysis: Deep Dive into Alternative Data Sources
Alternative data sources play a pivotal role in the success of dynamic scoring. The types of alternative data used can vary significantly, impacting the overall accuracy and effectiveness of the scoring model.
Data Source Category | Examples | Benefits | Challenges |
---|---|---|---|
Transactional Data | Bank account statements, payment history | Provides real-time insight into financial behavior. | Potential for privacy concerns, requires robust data security measures. |
Behavioral Data | Online spending patterns, bill payment behavior | Reveals consistent financial habits. | Data interpretation can be complex, requires advanced analytical techniques. |
Social Media Data | Social media activity, online reviews | Can indirectly indicate responsible behavior or financial stability. | Privacy concerns, potential for bias, requires careful data sanitization. |
Utility Payment Data | Electricity, water, and gas bill payments | Demonstrates consistent payment history. | Data availability may vary geographically. |
Rental History | Rental payment records | Indicates consistent rental payments, even in absence of credit history. | Data availability may be limited. |
Frequently Asked Questions About Dynamic Scoring
-
What is the difference between static and dynamic scoring? Static scoring uses a single point-in-time snapshot of credit history, while dynamic scoring continuously updates scores using real-time data.
-
What types of data are used in dynamic scoring? Dynamic scoring utilizes a range of data sources, including traditional credit bureau data, transactional data, behavioral data, and alternative data.
-
Is dynamic scoring more accurate than static scoring? Dynamic scoring generally offers greater accuracy due to its continuous update and use of richer data sets.
-
What are the privacy concerns associated with dynamic scoring? The use of sensitive personal data raises privacy concerns. Strict data protection measures and compliance with regulations are essential.
-
How can I improve my dynamic score? Maintain consistent and responsible financial behavior, such as timely payments and responsible spending habits.
-
Is dynamic scoring used by all lenders? Not all lenders currently use dynamic scoring, but its adoption is growing rapidly within the financial industry.
Practical Tips for Maximizing the Benefits of Dynamic Scoring
-
Maintain a consistent payment history: Timely payments across all accounts are crucial for a positive dynamic score.
-
Manage your debt effectively: Keep your debt-to-income ratio low to demonstrate responsible financial management.
-
Monitor your credit report regularly: Stay informed about your credit information and address any inaccuracies promptly.
-
Diversify your data sources: Engage in financial activities that generate positive data points across multiple data sources.
-
Consider building alternative credit history: If you have limited traditional credit history, actively establish alternative credit through utility payments or rental payments.
-
Avoid sudden changes in financial behavior: Large or unusual transactions can trigger alerts, so maintain consistent financial habits.
-
Understand your dynamic scoring model: If possible, seek to understand how your score is calculated to better manage your financial profile.
-
Protect your personal information: Safeguard your data to prevent unauthorized access and potential manipulation.
End Note: Embracing the Dynamic Future of Credit
With its transformative potential, dynamic scoring is reshaping the future of credit assessment across various industries. By embracing its principles and addressing the associated challenges, businesses and individuals can unlock new opportunities for growth and responsible financial management. The continuously evolving nature of dynamic scoring necessitates a proactive and informed approach to credit management. Understanding its implications is key to navigating the complexities of the modern financial landscape.
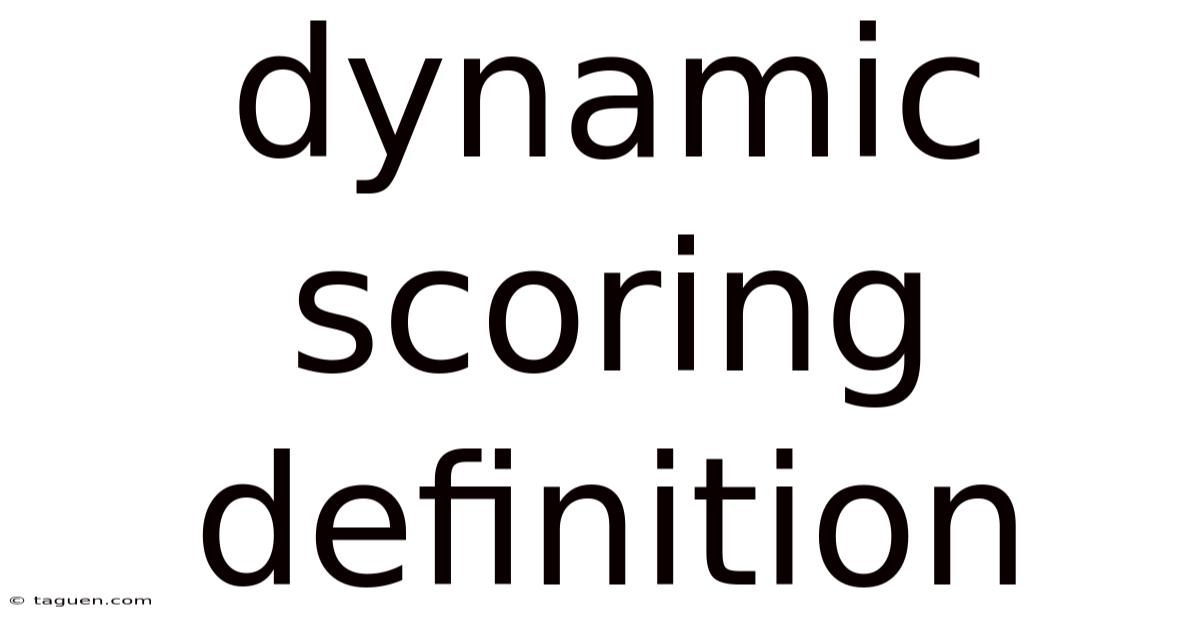
Thank you for visiting our website wich cover about Dynamic Scoring Definition. We hope the information provided has been useful to you. Feel free to contact us if you have any questions or need further assistance. See you next time and dont miss to bookmark.
Also read the following articles
Article Title | Date |
---|---|
Economist Intelligence Unit Eiu Definition | Mar 08, 2025 |
How Much Do Insurance Underwriters Make | Mar 08, 2025 |
How Long Does A Lapse In Car Insurance Stay On Your Record | Mar 08, 2025 |
Who Owns American Family Insurance | Mar 08, 2025 |
Elves Definition | Mar 08, 2025 |