Sampling Errors In Statistics Definition Types And Calculation
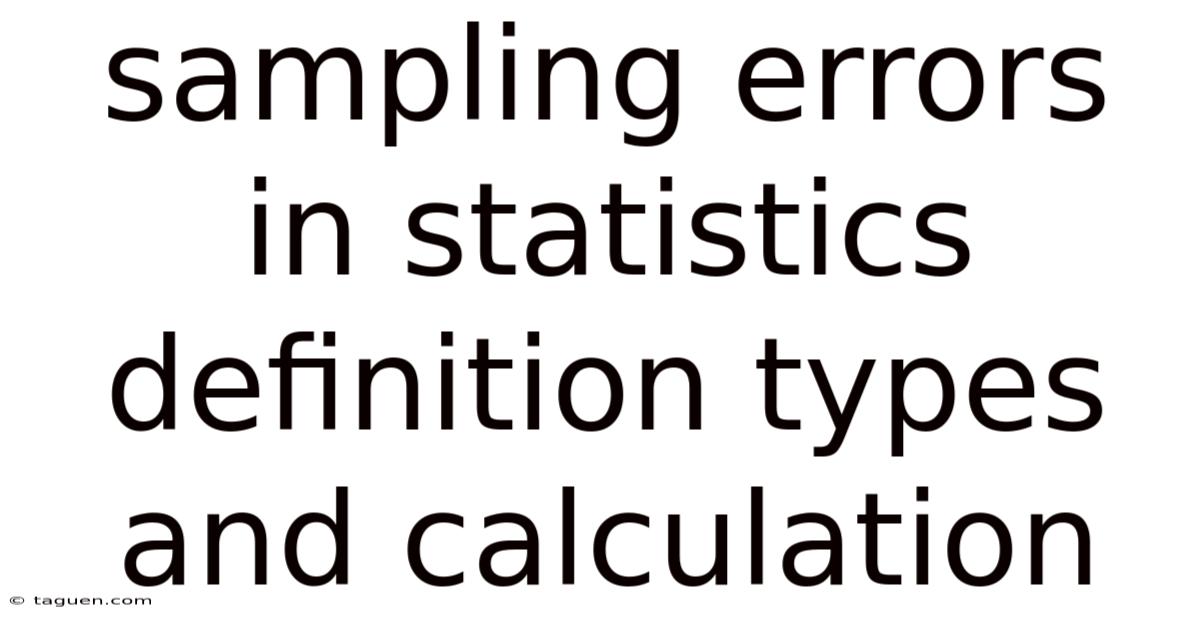
Discover more detailed and exciting information on our website. Click the link below to start your adventure: Visit Best Website meltwatermedia.ca. Don't miss out!
Table of Contents
Unmasking Sampling Errors: Definition, Types, and Calculation
What if the conclusions drawn from your research are fundamentally flawed because of how you collected your data? Understanding and mitigating sampling errors is crucial for the validity and reliability of any statistical analysis.
Editor's Note: This article on sampling errors in statistics has been meticulously researched and updated to provide the most current and accurate information available.
Sampling error, a ubiquitous challenge in statistical research, refers to the inherent difference between the characteristics of a sample and the characteristics of the population from which it's drawn. It arises because a sample, by definition, represents only a portion of the larger population. No matter how carefully a sample is selected, it's unlikely to perfectly mirror the population's attributes. Understanding the nature and extent of sampling error is paramount for drawing accurate and reliable conclusions from statistical analyses.
This article delves into the core aspects of sampling errors, examining their definition, various types, calculation methods, and strategies for minimization. Backed by practical examples and clear explanations, it provides actionable knowledge for researchers, students, and anyone working with statistical data.
Key Takeaways:
Concept | Description | Importance |
---|---|---|
Definition of Sampling Error | The difference between a sample statistic and the corresponding population parameter. | Establishes the foundation for understanding the limitations of sample-based inferences. |
Types of Sampling Errors | Random and Non-random (systematic) errors; further categorized by sampling methods used (e.g., simple random, stratified). | Understanding the source of error is crucial for selecting appropriate sampling techniques and error reduction strategies. |
Calculating Sampling Error | Involves estimating the standard error of the mean or proportion, depending on the data type and sampling method. | Quantifies the uncertainty associated with sample-based estimates. |
Minimizing Sampling Error | Utilizing larger sample sizes, employing appropriate sampling techniques (stratified, cluster), and careful data collection. | Ensures more precise and reliable estimations of population parameters. |
With a strong understanding of its relevance, let’s explore sampling errors further, uncovering their applications, challenges, and future implications.
Definition and Core Concepts
Sampling error is the discrepancy between a sample statistic (e.g., the sample mean, sample proportion) and the corresponding population parameter (e.g., the population mean, population proportion). This discrepancy arises simply because a sample is not a perfect representation of the whole population. It's a natural consequence of using a subset of data to make inferences about a larger dataset. The sample statistic provides an estimate of the population parameter, and the sampling error represents the error in that estimate.
For example, if we're trying to determine the average height of all adults in a country, we can't measure every single adult. Instead, we take a sample and calculate the average height of that sample. The difference between this sample average and the true average height of all adults in the country is the sampling error.
Types of Sampling Errors
Sampling errors broadly fall into two categories: random errors and non-random (or systematic) errors.
1. Random Errors: These errors are unpredictable and occur due to chance. They are inherent to the sampling process and are equally likely to overestimate or underestimate the population parameter. The magnitude of random error can be reduced by increasing the sample size. A larger sample is more likely to better represent the population's characteristics, minimizing the influence of random chance.
2. Non-Random (Systematic) Errors: These errors are not due to chance but are introduced by flaws in the sampling design or data collection process. These errors consistently bias the sample in a particular direction, leading to inaccurate estimates of the population parameter. Examples include:
- Selection Bias: Occurs when the sampling method systematically favors certain individuals or groups over others. For example, surveying people only at a shopping mall might exclude individuals who don't frequent shopping malls.
- Measurement Bias: Occurs when the method of collecting data systematically distorts the measurements. For instance, using a poorly calibrated scale to measure weights would introduce measurement bias.
- Non-response Bias: Occurs when a significant portion of the selected sample refuses to participate in the study or cannot be reached. This can lead to a biased sample if the non-respondents differ systematically from the respondents.
Calculating Sampling Error
The calculation of sampling error depends on the type of data and the sampling method used. The most common calculations involve the standard error.
Standard Error of the Mean (SEM): Used when estimating the population mean from a sample mean. It measures the variability of the sample means across multiple samples. The formula for SEM is:
SEM = s / √n
Where:
- s is the sample standard deviation
- n is the sample size
Standard Error of the Proportion (SEP): Used when estimating the population proportion from a sample proportion. It measures the variability of sample proportions across multiple samples. The formula for SEP is:
SEP = √[p(1-p) / n]
Where:
- p is the sample proportion
- n is the sample size
The standard error provides a measure of the precision of the sample statistic as an estimate of the population parameter. A smaller standard error indicates a more precise estimate. Confidence intervals are often constructed using the standard error to provide a range of plausible values for the population parameter.
Applications Across Industries
Understanding and minimizing sampling error is crucial across diverse fields:
- Market Research: Accurately gauging consumer preferences and market trends requires minimizing sampling bias to avoid misleading product development or marketing strategies.
- Public Health: Estimating disease prevalence or the effectiveness of interventions depends on representative samples to provide reliable public health data.
- Quality Control: Assessing product quality relies on proper sampling techniques to ensure quality standards are met across the entire production.
- Political Polling: Predicting election outcomes accurately necessitates large, representative samples to minimize sampling error and provide reliable poll results.
- Environmental Science: Monitoring environmental conditions requires careful sampling to obtain accurate data for environmental impact assessment and resource management.
Challenges and Solutions
Minimizing sampling error is a continuous challenge. Some key obstacles include:
- Accessibility to the population: Reaching every member of a target population is often difficult or impossible.
- Cost and time constraints: Larger sample sizes generally lead to lower sampling error, but they also increase the cost and time required for data collection.
- Maintaining sample representativeness: Ensuring the sample accurately reflects the characteristics of the population can be complex, especially with diverse populations.
Solutions to mitigate these challenges include:
- Employing appropriate sampling techniques: Stratified sampling, cluster sampling, and multi-stage sampling can increase the representativeness of the sample.
- Increasing the sample size: A larger sample reduces the influence of random error. Power analysis can help determine the optimal sample size for a given study.
- Careful data collection procedures: Implementing rigorous protocols to minimize measurement error and non-response bias is essential.
- Using statistical methods to adjust for bias: Techniques like weighting or regression analysis can help to correct for some types of bias.
Impact on Innovation
The accurate estimation of population parameters is essential for innovation across many fields. Sampling error, if not properly addressed, can lead to:
- Misinformed product development: Incorrect market research due to sampling error can lead to products that fail to meet consumer needs.
- Ineffective policy decisions: Biased data on social issues can lead to ineffective policy interventions.
- Failed clinical trials: Sampling error in clinical trials can lead to inaccurate conclusions about the effectiveness of new treatments.
Minimizing sampling error through improved sampling techniques and data analysis methods is therefore crucial for driving innovation and making sound decisions based on reliable data.
The Relationship Between Sample Size and Sampling Error
The relationship between sample size and sampling error is inversely proportional. As the sample size increases, the sampling error decreases. This is because a larger sample is more likely to accurately reflect the characteristics of the population. This relationship is encapsulated in the standard error formulas: as 'n' (sample size) increases, the standard error decreases. However, the rate of decrease diminishes as the sample size gets larger – the benefit of increasing sample size from 1000 to 1001 is much smaller than from 10 to 11. This underscores the importance of power analysis to determine the optimal sample size that balances precision with cost and feasibility.
Roles and Real-World Examples
- Market research: A company launching a new product uses a small, biased sample (e.g., only surveying their existing customers) to estimate market demand. This leads to an overestimation of demand, resulting in excess inventory and financial losses.
- Clinical trials: A clinical trial for a new drug uses a small sample size. The results show a statistically significant improvement, but due to the small sample size, the result is not reliable and may not reflect the true effect of the drug on a larger population.
- Political polling: A poll conducted just before an election uses a non-representative sample, overrepresenting one demographic group. The poll results inaccurately predict the election outcome.
Risks and Mitigations
The primary risk associated with large sampling errors is drawing inaccurate conclusions about the population. This can lead to wrong decisions in various fields, from business to healthcare to policy-making.
Mitigation strategies include careful planning of sampling methods, use of appropriate statistical techniques, and rigorous data quality control.
Impact and Implications
The implications of ignoring sampling error are significant. Incorrect conclusions can lead to wasted resources, ineffective policies, and flawed decision-making. It's crucial to acknowledge the inherent uncertainty associated with sampling and to use statistical methods to quantify and manage this uncertainty.
Further Analysis: Deep Dive into Non-Response Bias
Non-response bias occurs when a significant portion of the selected sample doesn't participate in the study. This can significantly skew the results if the characteristics of the non-respondents differ from those of the respondents. For example, if a survey on political opinions has a high non-response rate, and non-respondents tend to be more politically apathetic, the survey results will overrepresent the views of politically engaged individuals.
Analyzing non-response bias involves understanding the reasons for non-response and attempting to adjust for it. This might involve weighting the responses of respondents to account for the characteristics of the non-respondents, or using statistical modeling to estimate the missing data.
Frequently Asked Questions (FAQs)
Q1: How can I determine the appropriate sample size for my study?
A1: The appropriate sample size depends on several factors, including the desired level of precision, the variability of the population, and the type of analysis being conducted. Power analysis is a statistical technique used to determine the necessary sample size to detect a statistically significant effect.
Q2: What is the difference between sampling error and sampling bias?
A2: Sampling error is the natural variability inherent in using a sample to estimate a population parameter. Sampling bias, on the other hand, is a systematic error introduced by flaws in the sampling method or data collection process.
Q3: Can sampling error be completely eliminated?
A3: No, sampling error cannot be completely eliminated, as it's an inherent consequence of using a sample to make inferences about a population. However, it can be minimized through careful sampling design, larger sample sizes, and appropriate statistical techniques.
Q4: How do I know if my sampling error is too large?
A4: You can assess the magnitude of sampling error by calculating the standard error of the mean or proportion and constructing confidence intervals. If the confidence interval is too wide, it indicates that the sampling error is large and the estimate is imprecise.
Q5: What are some techniques to reduce non-response bias?
A5: Techniques to reduce non-response bias include using multiple modes of contact (phone, mail, email), offering incentives for participation, and employing callbacks to re-contact non-respondents.
Q6: What is the role of statistical software in managing sampling errors?
A6: Statistical software packages can greatly assist in managing sampling errors by facilitating power analysis (to determine sample size), calculating standard errors, constructing confidence intervals, and conducting various statistical tests to analyze data while considering potential sampling error.
Practical Tips for Maximizing the Benefits of Effective Sampling
- Clearly define your target population: Precisely identify the population you want to study to ensure your sampling methods accurately target it.
- Choose the appropriate sampling method: Select a method (simple random, stratified, cluster, etc.) that best suits your research objectives and the characteristics of your population.
- Determine an optimal sample size: Conduct a power analysis to determine the sample size needed to achieve the desired level of precision.
- Implement rigorous data collection procedures: Use standardized questionnaires and protocols to minimize measurement error and ensure consistent data quality.
- Monitor response rates and address non-response bias: Actively track response rates and, if significant non-response is observed, explore methods to account for potential bias.
- Analyze data appropriately: Use appropriate statistical methods to account for sampling error when interpreting results. Consider confidence intervals and margin of error.
- Document your methodology thoroughly: Clearly document your sampling methods, data collection procedures, and analysis techniques to enhance transparency and reproducibility.
- Seek expert advice: Consult with a statistician or other research expert to ensure your sampling strategy is sound and appropriate for your study.
Conclusion: Embracing the Reality of Sampling Error
Sampling error is an inevitable aspect of statistical inference. However, by understanding its nature, types, and methods for calculation and mitigation, researchers can significantly enhance the validity and reliability of their findings. Through careful planning, appropriate sampling techniques, and rigorous data analysis, we can minimize the impact of sampling error and draw more accurate conclusions based on sample data. Acknowledging and addressing sampling error is not a limitation; it is a crucial step towards responsible and effective data-driven decision-making. The ongoing development of sophisticated sampling techniques and statistical methods continues to improve our ability to manage and mitigate sampling error, driving progress across diverse fields.
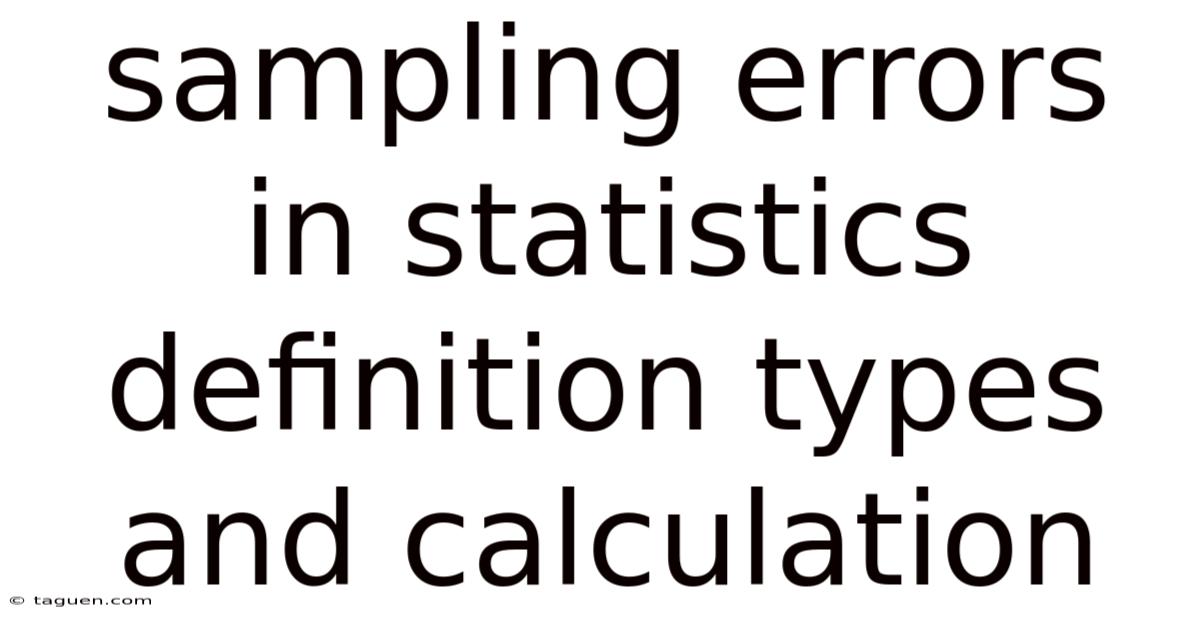
Thank you for visiting our website wich cover about Sampling Errors In Statistics Definition Types And Calculation. We hope the information provided has been useful to you. Feel free to contact us if you have any questions or need further assistance. See you next time and dont miss to bookmark.
Also read the following articles
Article Title | Date |
---|---|
Which Companies Still Offer Pensions | Apr 24, 2025 |
Which Jobs Offer Pensions | Apr 24, 2025 |
What Is A Realistic Return On Investment For Pension Funds | Apr 24, 2025 |
Sandbag Definition And Examples In Business And Finance | Apr 24, 2025 |
Rollover Mortgage Definition | Apr 24, 2025 |