Sample Selection Bias Definition Examples And How To Avoid
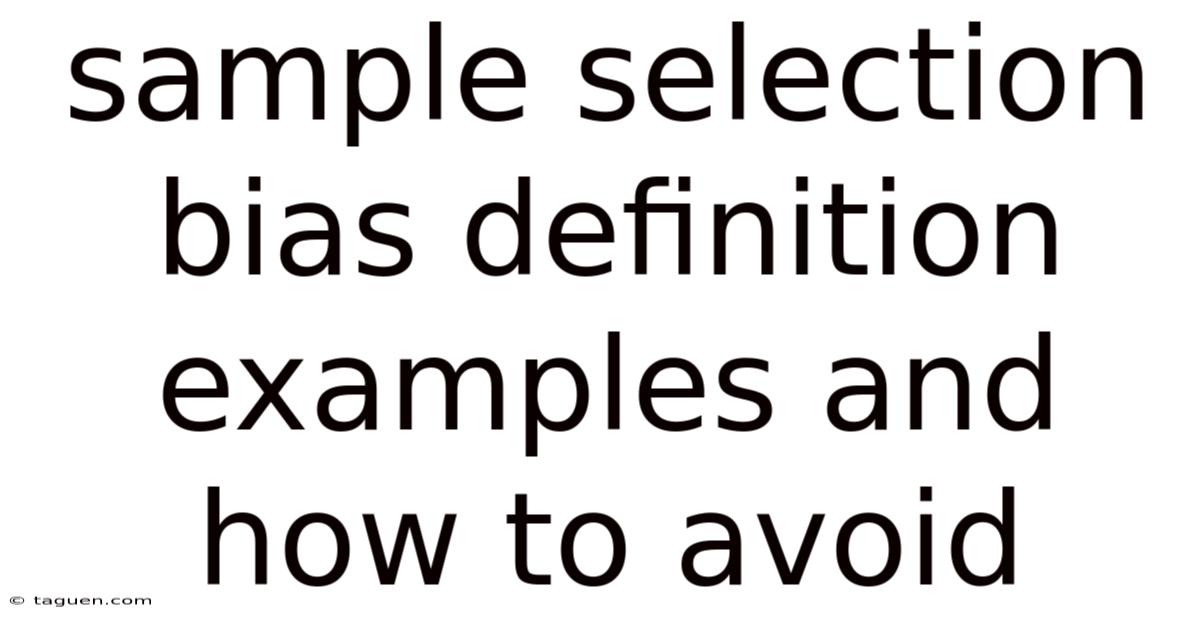
Discover more detailed and exciting information on our website. Click the link below to start your adventure: Visit Best Website meltwatermedia.ca. Don't miss out!
Table of Contents
Unveiling the Pitfalls of Sample Selection Bias: Definition, Examples, and Mitigation Strategies
What if the validity of your research hinges on the accuracy of your sample selection? Sample selection bias, a pervasive threat to research integrity, can severely distort findings and lead to inaccurate conclusions.
Editor’s Note: This article on sample selection bias provides a comprehensive overview of its definition, various examples across diverse fields, and practical strategies for its mitigation. Updated today with the latest research and best practices.
Sample selection bias, also known as selection bias, occurs when the way a sample is chosen systematically excludes or overrepresents certain parts of the population. This skewed representation leads to inaccurate generalizations about the population as a whole. The bias isn't about the size of the sample, but rather the process of selecting that sample. A large, biased sample is still a biased sample. Understanding and avoiding this bias is crucial for maintaining the rigor and reliability of research across numerous disciplines.
The Importance of Understanding Sample Selection Bias and Its Real-World Applications
Understanding sample selection bias is critical for anyone involved in research, data analysis, or decision-making processes based on data. The consequences of ignoring this bias can be substantial, leading to flawed conclusions, misallocation of resources, ineffective policies, and even harm to individuals. Its applications span various fields, including:
- Healthcare: Clinical trials susceptible to selection bias can lead to the approval of ineffective treatments or the rejection of effective ones.
- Marketing and Business: Market research using biased samples can result in inaccurate product development, ineffective marketing strategies, and missed business opportunities.
- Social Sciences: Surveys with biased samples can produce misleading conclusions about social trends, attitudes, and behaviors.
- Environmental Science: Studies on environmental impacts might misrepresent the true extent of damage if samples are not carefully chosen.
- Political Science: Polling data affected by selection bias can lead to inaccurate predictions of election outcomes and flawed policy recommendations.
This article delves into the core aspects of sample selection bias, examining its different forms, real-world manifestations, and practical strategies for prevention and mitigation. Backed by expert insights and illustrative examples, it provides actionable knowledge for researchers, analysts, and anyone working with data.
Key Takeaways:
Key Aspect | Description |
---|---|
Definition | Systematic error in sample selection leading to an unrepresentative sample of the population. |
Types | Convenience sampling, volunteer bias, healthy user bias, referral bias, attrition bias, etc. |
Consequences | Inaccurate conclusions, flawed research, misleading policy recommendations, misallocation of resources. |
Mitigation Strategies | Random sampling, stratified sampling, cluster sampling, weighting techniques, rigorous inclusion/exclusion criteria. |
Real-World Examples | Numerous examples across various fields illustrating the impact of selection bias. |
With a strong understanding of its relevance, let’s explore sample selection bias further, uncovering its various forms, challenges, and the strategies to mitigate its impact.
Defining and Understanding the Core Concepts of Sample Selection Bias
Sample selection bias arises from a systematic discrepancy between the sample and the population it intends to represent. This discrepancy isn't random; it's a consistent pattern of over- or under-representation of specific groups within the population. This systematic error compromises the generalizability of findings. A perfectly representative sample accurately reflects the proportions of different characteristics (age, gender, income, etc.) present in the larger population. A biased sample does not.
Types of Sample Selection Bias:
Several types of selection bias exist, each with its own unique mechanism:
- Convenience Sampling: This involves selecting participants who are easily accessible, leading to a non-representative sample. For example, surveying only students in one classroom to represent the entire school's opinion.
- Volunteer Bias: Participants who volunteer for a study might differ systematically from those who don't, creating a biased sample. Volunteers might be more motivated, have more free time, or possess specific characteristics.
- Healthy User Bias: This is common in health research where healthier individuals are more likely to participate in studies, leading to an underrepresentation of those with severe health conditions.
- Referral Bias: Participants are referred by others, leading to a sample that shares characteristics with the referrers. This bias is often seen in clinical trials where doctors might refer patients with certain characteristics.
- Attrition Bias: This occurs when participants drop out of a longitudinal study, resulting in a sample that differs from the initial sample. Those who drop out might share specific characteristics, leading to biased results.
- Length-Time Bias: In studies involving diseases with long latency periods, cases diagnosed earlier might be overrepresented, leading to a skewed understanding of the disease's incidence and progression.
Applications of Sample Selection Bias Across Industries
The pervasiveness of sample selection bias is evident across various sectors:
- Clinical Trials: A drug trial that only includes patients from a specific demographic (e.g., young, healthy males) might show positive results that don't generalize to the wider population.
- Market Research: A survey conducted solely in affluent neighborhoods to gauge consumer preferences for a new product will likely not accurately reflect the preferences of the overall market.
- Political Polling: Calling only landlines to conduct a political poll will likely exclude younger demographics who predominantly use cell phones, resulting in a biased representation of voter preferences.
- Educational Research: Evaluating a new teaching method only in a high-performing school will not reveal its effectiveness in diverse learning environments.
Challenges and Solutions to Mitigate Sample Selection Bias
Addressing sample selection bias requires careful planning and execution of the research design. Key strategies include:
- Random Sampling: This ensures every member of the population has an equal chance of being selected, minimizing systematic biases.
- Stratified Sampling: This involves dividing the population into subgroups (strata) and then randomly sampling from each stratum, ensuring representation from all groups.
- Cluster Sampling: This technique involves randomly selecting clusters (e.g., schools, hospitals) and then sampling all individuals within the selected clusters.
- Weighting Techniques: This statistical approach adjusts the relative influence of different groups in the sample to better reflect their proportions in the population.
- Rigorous Inclusion/Exclusion Criteria: Clearly defining the criteria for participant selection helps to minimize bias by ensuring a more homogenous sample.
- Blinding: In studies involving subjective assessments, blinding participants and researchers to group assignments can reduce bias.
The Impact of Sample Selection Bias on Innovation
Sample selection bias can severely hinder innovation. Flawed research due to bias can lead to:
- Ineffective products or services: Companies might develop products based on inaccurate market research, leading to failure.
- Misguided policy decisions: Government policies based on biased data may not achieve their intended outcomes.
- Slowed scientific progress: Inaccurate research findings can hinder the advancement of scientific understanding.
Reinforcing Key Themes: A Concise Closing Summary
Sample selection bias is a significant threat to the validity and reliability of research findings. By carefully choosing sampling methods and implementing strategies to mitigate bias, researchers can ensure that their studies generate accurate and generalizable results. Ignoring this bias can lead to costly mistakes, ineffective interventions, and a hampered understanding of complex phenomena. Rigorous methodology is essential to overcome this challenge.
Exploring the Relationship Between Randomization and Sample Selection Bias
The cornerstone of avoiding sample selection bias is randomization. Random sampling ensures that each member of the population has an equal probability of selection. This, in principle, eliminates systematic biases, as the selection process is purely chance-driven. However, even with random sampling, practical challenges can arise:
- Non-response bias: Even with a randomly selected sample, some individuals may refuse to participate, leading to a non-representative sample. This non-response bias can introduce its own form of selection bias.
- Sampling frame error: The sampling frame (the list from which the sample is drawn) might not accurately represent the entire population. For example, a phone directory might exclude individuals without landlines.
Roles and Real-World Examples:
- Clinical Trials: Randomized controlled trials (RCTs) are considered the gold standard for minimizing selection bias in clinical research. However, challenges remain in ensuring diverse representation and addressing attrition bias.
- Social Surveys: Random digit dialing is a method to mitigate selection bias in telephone surveys, ensuring that both landlines and cell phones are included.
Risks and Mitigations:
The risk of selection bias is high when convenience sampling or other non-random methods are employed. Mitigating this risk involves implementing rigorous sampling strategies, careful consideration of the sampling frame, and addressing non-response bias through strategies such as follow-up calls or incentives.
Impact and Implications:
The implications of selection bias can be substantial, ranging from misallocation of resources to inaccurate policy decisions and hindered scientific advancement. The use of robust statistical methods, careful analysis, and transparency in reporting are critical for minimizing the impact of this bias.
Further Analysis: Deep Dive into Randomization
Randomization is a powerful tool for mitigating selection bias. Its effectiveness stems from its ability to minimize the influence of confounding variables – factors that might be associated with both the outcome of interest and the exposure or treatment under study. Random assignment to treatment and control groups ensures that these confounding variables are distributed equally across groups, thereby isolating the effect of the treatment.
Advantages of Randomization:
- Minimizes confounding: Ensures that confounding variables are evenly distributed across groups.
- Reduces selection bias: Removes systematic differences between groups in terms of characteristics that may influence the outcome.
- Increases the generalizability of results: Makes it more likely that the findings can be applied to the broader population.
Limitations of Randomization:
- Practical challenges: Randomization can be difficult to implement in certain settings, such as in observational studies.
- Chance imbalances: Even with randomization, chance imbalances in characteristics can occur between groups, although these are usually less severe than systematic biases.
Frequently Asked Questions About Sample Selection Bias
-
What is the difference between sample selection bias and sampling error? Sampling error is the natural variation between the sample and the population due to chance, while selection bias is a systematic error due to the way the sample was selected.
-
How can I tell if my study has sample selection bias? Look for evidence of systematic differences between your sample and the population it represents. Compare demographic characteristics, and examine whether specific groups are under- or overrepresented.
-
What is the best way to avoid sample selection bias? The most effective method is random sampling, supplemented by strategies to minimize non-response bias and address potential limitations in the sampling frame.
-
Can I correct for sample selection bias after the data has been collected? Statistical adjustments (e.g., weighting) can help to mitigate bias, but they cannot fully eliminate it. Preventing bias during the sampling stage is crucial.
-
Is sample selection bias always a problem? Yes, any systematic error in sample selection can affect the validity of research. Even small biases can have substantial consequences, especially in large-scale studies.
-
How important is sample size in relation to sample selection bias? Sample size is important for statistical power and precision, but it does not mitigate selection bias. A large biased sample still produces biased results.
Practical Tips for Maximizing the Benefits of Avoiding Sample Selection Bias
- Define your target population: Clearly specify the population you are studying.
- Develop a comprehensive sampling plan: Choose an appropriate sampling method (random sampling is ideal) and address potential challenges.
- Develop clear inclusion and exclusion criteria: Define criteria to select participants in a way that avoids bias.
- Pilot test your methods: Conduct a small-scale pilot study to identify and address any potential biases before the main study begins.
- Monitor response rates and address non-response bias: Employ strategies to maximize response rates and account for possible differences between responders and non-responders.
- Analyze your data carefully: Use appropriate statistical methods to analyze your data and consider potential biases during interpretation.
- Report your methods transparently: Clearly document your sampling methods and any limitations in your study.
- Consider using multiple data sources: Triangulating your findings with data from multiple sources can help reduce the impact of biases in any single data source.
Conclusion: Embracing Rigor to Unlock Accurate Insights
Sample selection bias poses a significant threat to the accuracy and reliability of research. By understanding its various forms, employing rigorous sampling techniques, and carefully considering potential biases throughout the research process, we can ensure that our studies produce valid and generalizable findings. The pursuit of rigorous methodology is not merely a technical exercise; it's a commitment to the integrity of knowledge and the effective use of research in informing decisions across all fields. By prioritizing rigorous approaches, we can unlock accurate insights that lead to impactful advancements in science, policy, and practice.
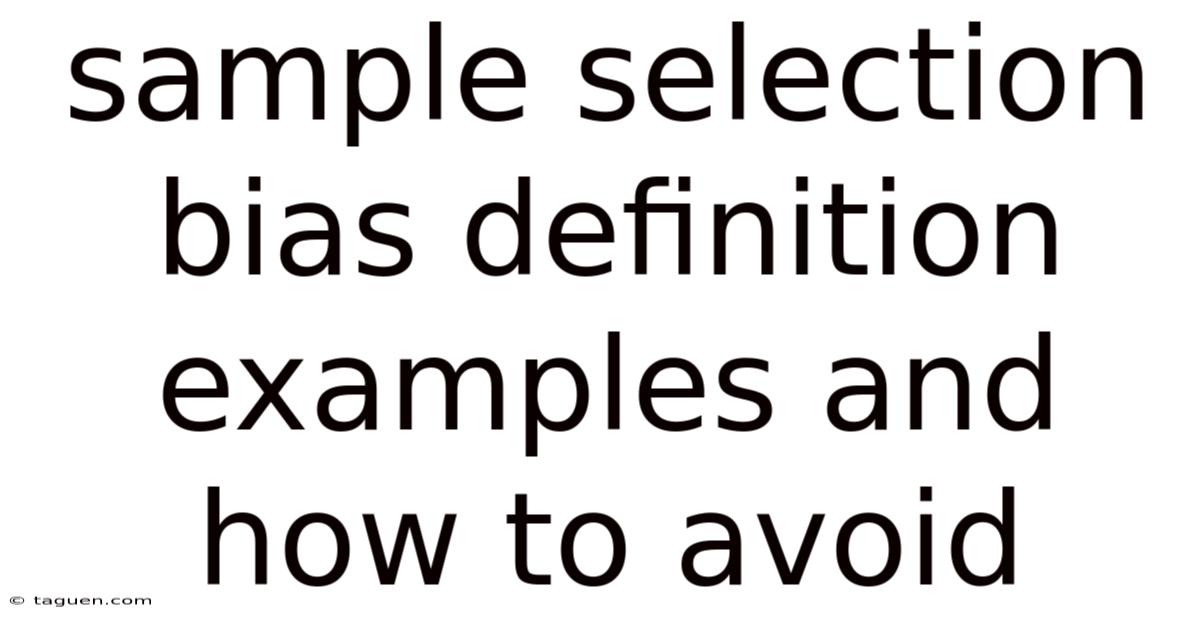
Thank you for visiting our website wich cover about Sample Selection Bias Definition Examples And How To Avoid. We hope the information provided has been useful to you. Feel free to contact us if you have any questions or need further assistance. See you next time and dont miss to bookmark.
Also read the following articles
Article Title | Date |
---|---|
Rural Housing Service Rhs Definition | Apr 24, 2025 |
How Do State Public Pension Funds Amortize Portfolio Losses | Apr 24, 2025 |
When Does The Ex Spouse Collect Pension Funds | Apr 24, 2025 |
Roth Ordering Rules Definition | Apr 24, 2025 |
Why Are Life Insurance Companies Pension Funds And Mutual Funds Considered Financial Institution | Apr 24, 2025 |