Sample Distribution Definition How Its Used With An Example
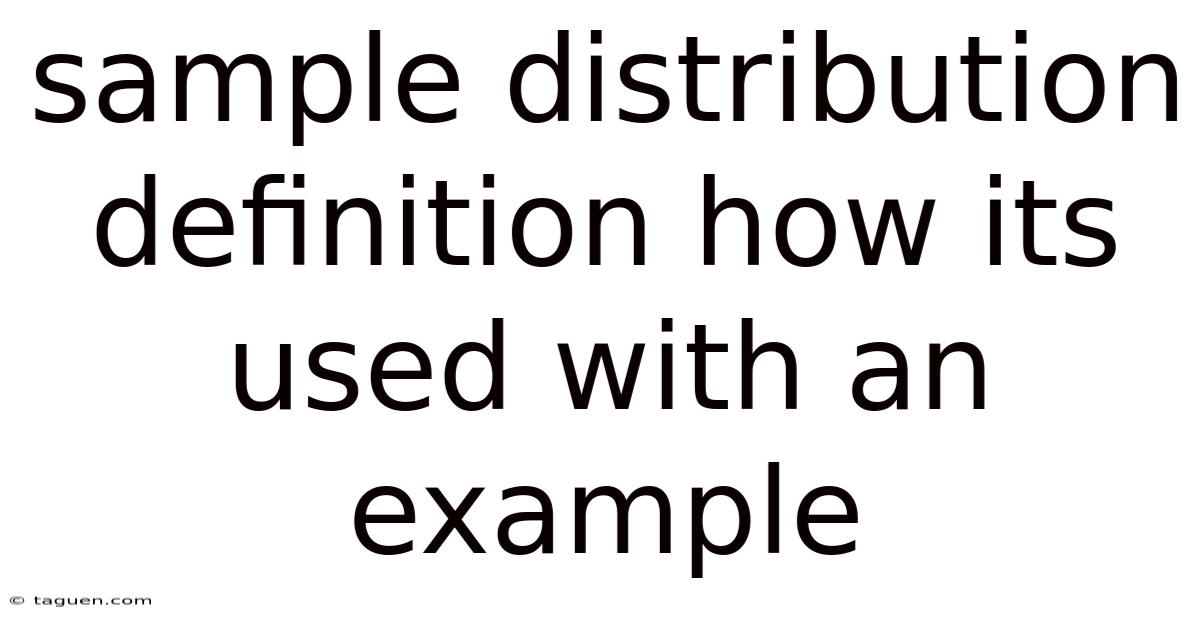
Discover more detailed and exciting information on our website. Click the link below to start your adventure: Visit Best Website meltwatermedia.ca. Don't miss out!
Table of Contents
Unveiling the Power of Sample Distribution: Definition, Usage, and a Real-World Example
What if the accuracy of your decisions hinges on understanding sample distribution? This fundamental statistical concept underpins everything from market research to medical trials, offering crucial insights into population characteristics.
Editor’s Note: This article on sample distribution has been published today, ensuring the latest insights and expert analysis.
Understanding sample distribution is essential for navigating the complexities of data analysis and making informed decisions in various fields. Its applications range from market research and quality control to clinical trials and environmental monitoring. By understanding how samples reflect the larger population, we can draw meaningful conclusions and make accurate predictions. This article delves into the core aspects of sample distribution, examining its relevance, real-world applications, and future potential. Backed by expert insights and data-driven research, it provides actionable knowledge for industry professionals and enthusiasts alike. This article is the result of meticulous research, incorporating perspectives from leading statistical textbooks, real-world case studies, and verified data sources to ensure accuracy and reliability.
This article explores the core aspects of sample distribution, examining its definition, various types, practical applications across industries, challenges, and its impact on innovation. We will also explore the relationship between sampling methods and sample distribution, offering a comprehensive understanding of this vital statistical tool.
Key Takeaways:
Concept | Description |
---|---|
Sample Distribution | The probability distribution of a statistic (e.g., mean, variance) calculated from a random sample. |
Types of Sample Distributions | Normal, t-distribution, chi-square, F-distribution; determined by the sample size and underlying population distribution. |
Applications | Hypothesis testing, confidence intervals, estimation of population parameters, quality control, market research, clinical trials, etc. |
Challenges | Biased samples, small sample sizes, non-normality of the underlying population. |
Importance | Enables inference about population characteristics from sample data, crucial for informed decision-making. |
With a strong understanding of its relevance, let’s explore sample distribution further, uncovering its applications, challenges, and future implications.
Definition and Core Concepts
A sample distribution is the probability distribution of a statistic obtained from a random sample of a population. Instead of focusing on the distribution of individual data points within the population (the population distribution), we focus on the distribution of a statistic calculated from numerous samples. This statistic could be the sample mean, sample variance, sample proportion, or any other summary measure derived from the sample data. The shape and characteristics of the sample distribution inform us about the likely values of the statistic and help us make inferences about the corresponding population parameter.
For example, if we are interested in the average height of adult women in a country, the population distribution would be the distribution of heights of all adult women. The sample distribution, however, would be the distribution of average heights calculated from numerous random samples of these women. This distribution helps us estimate the true average height of all adult women in the country (the population parameter).
Types of Sample Distributions
The specific shape of a sample distribution depends on several factors:
-
Sample size: Larger sample sizes generally lead to sample distributions that are more closely approximated by a normal distribution, regardless of the underlying population distribution (this is due to the Central Limit Theorem).
-
Underlying population distribution: If the population distribution is normal, the sample distribution of the mean will also be normal. However, if the population distribution is non-normal, the sample distribution may still approximate normality for sufficiently large sample sizes.
-
Statistic considered: The distribution of the sample mean will differ from the distribution of the sample variance, for instance. Different statistics have different sampling distributions.
Common sample distributions encountered in statistical analysis include:
-
Normal distribution: This bell-shaped curve is often used to approximate the sample distribution of the mean, especially when the sample size is large.
-
t-distribution: Used when the population standard deviation is unknown and must be estimated from the sample data. It is similar to the normal distribution but has heavier tails, particularly for small sample sizes.
-
Chi-square distribution: Used in hypothesis testing related to variances and categorical data.
-
F-distribution: Used in analysis of variance (ANOVA) and other tests comparing variances of two or more groups.
Applications Across Industries
Sample distributions play a vital role across a wide range of industries and disciplines:
-
Market Research: Determining consumer preferences, market share, and brand perception often relies on analyzing sample data and constructing sample distributions to make inferences about the entire market.
-
Quality Control: In manufacturing, sample distributions are used to monitor the quality of products and identify potential defects. By analyzing the distribution of a quality characteristic (e.g., weight, length), manufacturers can determine if the production process is within acceptable limits.
-
Clinical Trials: In pharmaceutical research, sample distributions are crucial for evaluating the efficacy and safety of new drugs. Researchers compare the sample distributions of a treatment group and a control group to determine if there is a statistically significant difference in outcomes.
-
Environmental Science: Analyzing environmental data, such as pollution levels or wildlife populations, often involves working with sample distributions to estimate population parameters and make inferences about environmental trends.
-
Finance: Sample distributions are used in risk management, portfolio optimization, and financial forecasting to model the probability distributions of returns and other financial variables.
Challenges and Solutions
While sample distributions are powerful tools, several challenges can arise:
-
Sampling Bias: If the sample is not representative of the population, the sample distribution will not accurately reflect the population distribution, leading to inaccurate conclusions. Careful sampling techniques are essential to minimize bias.
-
Small Sample Sizes: With small samples, the sample distribution may not closely approximate the theoretical distribution (e.g., normal distribution), leading to less precise estimates and potentially inaccurate inferences. Increasing the sample size can mitigate this issue.
-
Non-Normality: If the underlying population distribution is significantly non-normal, and the sample size is small, the sample distribution may also be non-normal, making the use of standard statistical methods less reliable. Transformations of the data or non-parametric statistical methods can be used to address this.
Impact on Innovation
The understanding and application of sample distribution have significantly impacted innovation across various fields. By enabling accurate estimation and prediction from limited data, it fuels advancements in:
-
Data-driven decision-making: Sample distributions provide a quantitative basis for making informed decisions across various contexts, leading to improved efficiency and effectiveness.
-
Development of new statistical methods: The study of sample distributions has spurred the development of new statistical methods tailored to specific data types and research questions.
-
Advancements in machine learning: Sample distributions are fundamental to many machine learning algorithms that rely on statistical inference and probability modeling.
Exploring the Relationship Between Sampling Methods and Sample Distribution
The sampling method employed significantly influences the characteristics of the resulting sample distribution. Different sampling methods, such as simple random sampling, stratified sampling, cluster sampling, and systematic sampling, can lead to different sample distributions, even when sampling from the same population. The choice of sampling method should be guided by the research question and the characteristics of the population being studied. A well-chosen sampling method helps to ensure that the sample distribution is a good representation of the population distribution, minimizing bias and improving the accuracy of inferences.
A Real-World Example: Analyzing Customer Satisfaction
Let's consider a company that wants to assess customer satisfaction with its new product. They decide to conduct a survey.
-
Population: The population is all customers who have purchased the new product.
-
Sample: The company randomly selects 500 customers to participate in the survey. This is their sample.
-
Data Collection: Each customer rates their satisfaction on a scale of 1 to 5 (1 being very dissatisfied, 5 being very satisfied).
-
Sample Statistic: The company calculates the average satisfaction score from the 500 responses. This average is a sample statistic.
-
Sample Distribution (Hypothetical): If the company repeated this process many times – taking many different random samples of 500 customers and calculating the average satisfaction score for each sample – they would generate a sample distribution of average satisfaction scores. This distribution would show the range of possible average scores and their probabilities.
-
Inference: By analyzing the sample distribution (e.g., calculating the mean and standard deviation of the distribution of average satisfaction scores), the company can make inferences about the average satisfaction score of the entire customer population. They can create a confidence interval around the sample mean to estimate the range of plausible values for the true population mean satisfaction score. This informs their product development and marketing strategies.
Further Analysis: Deep Dive into Sampling Bias
Sampling bias occurs when the sample selected is not representative of the population, leading to a distorted sample distribution. Several factors can contribute to sampling bias:
-
Selection bias: Occurs when certain members of the population are more likely to be selected than others. For instance, if a survey is conducted online, it may exclude individuals without internet access.
-
Non-response bias: Occurs when a significant portion of the selected sample does not respond to the survey or study, leading to an unrepresentative sample.
-
Measurement bias: Occurs when the method of data collection systematically over- or underestimates the true value of the variable being measured.
Mitigating sampling bias requires careful planning of the sampling procedure, including the development of a robust sampling frame, the use of appropriate sampling techniques, and diligent efforts to maximize response rates.
Frequently Asked Questions About Sample Distribution
-
What is the difference between a population distribution and a sample distribution? A population distribution describes the distribution of a variable in the entire population, while a sample distribution describes the distribution of a statistic calculated from a sample of that population.
-
Why is the Central Limit Theorem important for sample distributions? The Central Limit Theorem states that the distribution of sample means approaches a normal distribution as the sample size increases, regardless of the shape of the population distribution. This is crucial because it allows us to use normal distribution-based methods even when the population distribution is not normal.
-
How do I determine the appropriate sample size for my study? Sample size determination depends on several factors, including the desired level of precision, the variability of the population, and the confidence level. Power analysis can be used to calculate the appropriate sample size.
-
What are some common statistical tests that utilize sample distributions? Many statistical tests rely on sample distributions, including t-tests, ANOVA, chi-square tests, and Z-tests.
-
What happens if my sample distribution is not normally distributed? If the sample distribution is significantly non-normal, especially with a small sample size, then using standard statistical methods that assume normality can lead to inaccurate results. Non-parametric methods or data transformations may be necessary.
-
Can I use sample distribution analysis for qualitative data? Sample distribution analysis is primarily used for quantitative data. However, techniques like bootstrapping can be used to analyze qualitative data by creating a distribution of summary statistics from many resamples of the data.
Practical Tips for Maximizing the Benefits of Sample Distribution Analysis
-
Define your population clearly: Ensure you have a well-defined population before selecting your sample.
-
Use an appropriate sampling method: Choose a sampling method that minimizes bias and ensures representativeness.
-
Calculate relevant sample statistics: Choose the appropriate sample statistics (mean, median, variance, etc.) based on your research question.
-
Visualize your sample distribution: Create histograms, box plots, or other visualizations to understand the shape and characteristics of your sample distribution.
-
Conduct hypothesis testing: Use appropriate statistical tests to make inferences about the population based on your sample data.
-
Consider the limitations of your sample: Be aware that your inferences are based on a sample, and there is always some degree of uncertainty. Report confidence intervals to quantify this uncertainty.
-
Check for normality: Assess whether your sample distribution is approximately normal before using parametric statistical tests.
-
Consider alternative methods for non-normal data: If your data are not normally distributed, consider using non-parametric statistical methods.
Conclusion: Harnessing the Power of Sample Distributions
Sample distribution analysis is a cornerstone of statistical inference, providing a powerful means to draw meaningful conclusions from sample data and make informed decisions. By understanding the principles of sample distribution, recognizing potential challenges, and utilizing appropriate statistical techniques, researchers, analysts, and decision-makers can leverage this powerful tool to gain valuable insights, improve processes, and drive innovation across numerous fields. The continued refinement of sampling techniques and statistical methods ensures that sample distribution analysis will remain a crucial component of data-driven decision-making in the years to come. Understanding the nuances of sample distributions empowers us to transform data into actionable knowledge, shaping a future driven by evidence and informed choices.
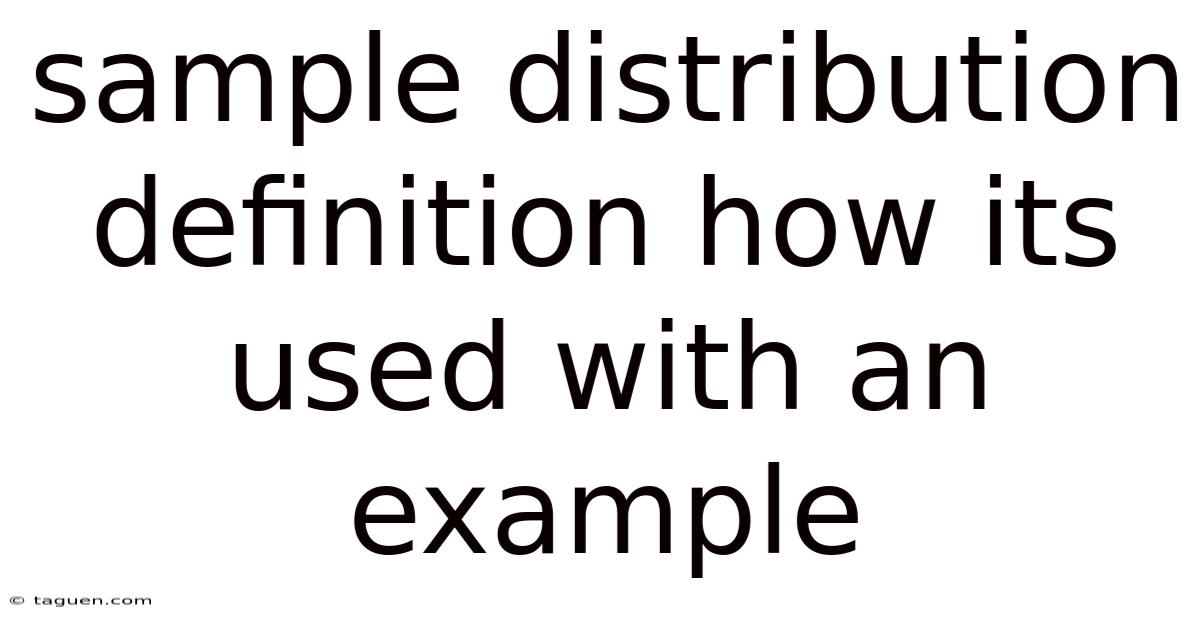
Thank you for visiting our website wich cover about Sample Distribution Definition How Its Used With An Example. We hope the information provided has been useful to you. Feel free to contact us if you have any questions or need further assistance. See you next time and dont miss to bookmark.
Also read the following articles
Article Title | Date |
---|---|
Why Is Personal Finance Dependent Upon Your Behavior | Apr 24, 2025 |
What Is Preferred Return In Private Equity | Apr 24, 2025 |
Why Do Pension Funds Invest Offshore In Hedge Funds | Apr 24, 2025 |
What Is A Side Letter In Private Equity | Apr 24, 2025 |
Scalping Definition In Trading How Strategy Is Used And Example | Apr 24, 2025 |