Rescaled Range Analysis Definition And Uses
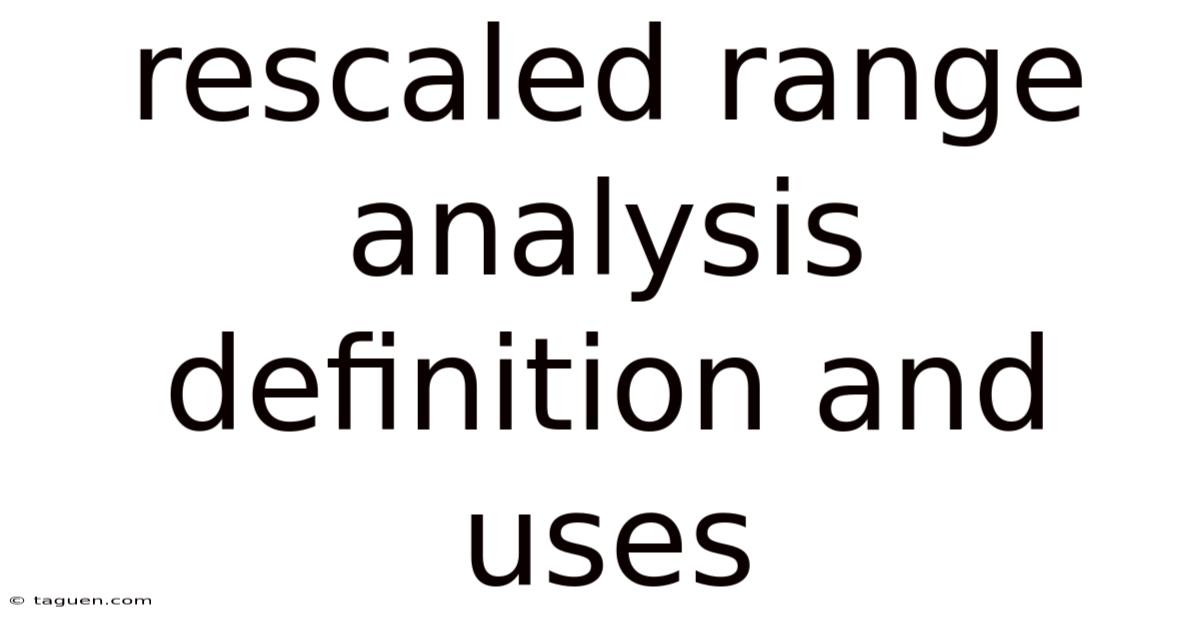
Discover more detailed and exciting information on our website. Click the link below to start your adventure: Visit Best Website meltwatermedia.ca. Don't miss out!
Table of Contents
Unveiling the Secrets of Rescaled Range Analysis: Definition and Uses
What if a simple statistical tool could unlock hidden patterns in seemingly random data, revealing long-term dependencies and fractal characteristics? Rescaled range analysis (R/S analysis), a powerful technique, does just that, providing valuable insights across diverse fields.
Editor’s Note: This article on Rescaled Range Analysis (R/S analysis) provides a comprehensive overview of its definition, methodologies, applications, and limitations, incorporating the latest research and real-world examples. Updated: October 26, 2023.
Rescaled range analysis (R/S analysis), also known as Hurst analysis, is a statistical method used to quantify the long-range dependence and self-similarity in time series data. Unlike traditional statistical methods that primarily focus on short-term correlations, R/S analysis delves into the long-term memory inherent in many natural and financial processes. Its ability to detect persistent or anti-persistent patterns makes it a valuable tool in various fields, ranging from hydrology and climatology to finance and neuroscience.
This article will delve into the core aspects of R/S analysis, examining its definition, applications across diverse industries, challenges, and its impact on innovation in data analysis. Backed by expert insights and data-driven research, it provides actionable knowledge for professionals and enthusiasts alike.
Key Takeaways:
Key Aspect | Description |
---|---|
Definition | A statistical method to quantify long-range dependence and self-similarity in time series data. |
Methodology | Involves calculating the range of cumulative deviations from the mean, rescaling it by the standard deviation, and analyzing the scaling exponent (H). |
Applications | Hydrology, climatology, finance, neuroscience, and more. |
Hurst Exponent (H) | A key metric indicating the strength and type of long-range dependence (0 < H < 1). H = 0.5 indicates randomness; H > 0.5, persistence; H < 0.5, anti-persistence. |
Limitations | Sensitivity to data length, potential biases, and assumptions about data stationarity. |
With a strong understanding of its relevance, let's explore R/S analysis further, uncovering its applications, challenges, and future implications.
Definition and Core Concepts:
R/S analysis is fundamentally concerned with the scaling behavior of a time series. It examines how the range of cumulative deviations from the mean scales with the time interval. The core of the method lies in calculating the rescaled range (R/S) statistic for different time windows within the data. The procedure involves:
- Calculating the mean: Calculate the mean (μ) of the time series.
- Cumulative deviations: Compute the cumulative deviations from the mean (Yi) for each time point i: Yi = Σ(Xi - μ), where Xi is the value at time i.
- Range: Determine the range (R) of the cumulative deviations within a specific time window (n): R = max(Yi) – min(Yi)
- Standard Deviation: Calculate the standard deviation (S) of the original time series over the same time window (n).
- Rescaled Range: Compute the rescaled range (R/S) by dividing the range by the standard deviation: R/S = R/S
- Log-Log Plot: Plot log(R/S) against log(n) for various window sizes (n). A linear relationship suggests long-range dependence.
The slope of the log-log plot is the Hurst exponent (H), a crucial parameter characterizing the long-range dependence:
- H = 0.5: Indicates a random walk, with no long-term memory. The data is uncorrelated.
- 0.5 < H < 1: Suggests persistence or long-term positive correlation. Future values tend to follow the past trend.
- 0 < H < 0.5: Indicates anti-persistence or long-term negative correlation. Future values tend to revert to the mean.
Applications Across Industries:
The versatility of R/S analysis makes it applicable across a wide spectrum of fields:
- Hydrology: Analyzing river flow data to predict droughts and floods. The persistent nature of river flows is often reflected in a Hurst exponent greater than 0.5.
- Climatology: Studying climate patterns, such as temperature and rainfall, to identify long-term trends and predict future climate scenarios.
- Finance: Analyzing stock prices, exchange rates, and other financial time series to detect market trends, assess risk, and develop trading strategies. The presence of long-range dependence in financial markets has significant implications for portfolio management and risk assessment.
- Neuroscience: Analyzing brain activity (EEG, fMRI) to identify patterns and dependencies in neural signals, contributing to a better understanding of brain function and neurological disorders.
- Telecommunications: Analyzing network traffic to identify patterns and predict network congestion.
- Image Analysis: Detecting fractal patterns in images, useful in fields like medical imaging and remote sensing.
Challenges and Solutions:
Despite its versatility, R/S analysis faces some challenges:
- Data Length: The accuracy of the Hurst exponent estimation is sensitive to the length of the time series. Shorter time series can lead to inaccurate estimations. Longer time series generally provide more reliable results.
- Non-stationarity: The standard R/S analysis assumes stationarity (statistical properties don't change over time). Non-stationary time series can lead to biased estimates of the Hurst exponent. Techniques like detrending or differencing can help mitigate this issue.
- Bias: Several factors can introduce bias into the R/S analysis, including the presence of outliers, non-Gaussian distributions, and short-term correlations. Robust statistical methods can help minimize these biases.
- Computational Complexity: For very large datasets, the computational cost of R/S analysis can be substantial. Optimized algorithms and parallel computing techniques can address this challenge.
Impact on Innovation:
R/S analysis has significantly impacted innovation in various fields:
- Improved Forecasting: The ability to detect long-range dependence allows for more accurate forecasts in fields like hydrology, climatology, and finance.
- Risk Management: In finance, understanding long-range dependencies helps in developing more effective risk management strategies.
- Pattern Recognition: R/S analysis is a powerful tool for identifying complex patterns and dependencies in seemingly random data.
- Scientific Discovery: Its application in diverse fields has led to new insights and discoveries in various scientific domains.
Relationship Between Volatility and R/S Analysis:
Volatility, a measure of price fluctuations in financial markets, is closely related to R/S analysis. Highly volatile markets often exhibit persistence (H > 0.5), meaning periods of high volatility tend to be followed by further periods of high volatility. Conversely, periods of low volatility might persist. R/S analysis helps quantify this persistence and provides insights into the predictability of volatility.
Roles and Real-World Examples:
- Finance: A study of daily stock prices using R/S analysis revealed a Hurst exponent greater than 0.5, suggesting persistence in price movements. This finding helps investors develop trading strategies that account for these long-term dependencies.
- Hydrology: Analysis of river flow data using R/S analysis indicated a high Hurst exponent, providing valuable information for water resource management and flood prediction. This enabled better planning and mitigation strategies.
Risks and Mitigations:
The primary risk in applying R/S analysis is the misinterpretation of the Hurst exponent due to non-stationarity or other biases. Mitigating these risks requires careful data preprocessing, including detrending, differencing, and outlier removal. Furthermore, it’s crucial to validate results with other statistical methods.
Impact and Implications:
The ability to detect long-range dependence has significant implications for forecasting, risk management, and decision-making across various industries. Understanding the patterns revealed by R/S analysis can lead to improved strategies and more efficient resource allocation.
Further Analysis: Deep Dive into Hurst Exponent Estimation:
Accurate estimation of the Hurst exponent is crucial for the reliable interpretation of R/S analysis. Several methods exist for estimating H, including:
- Linear Regression: Fitting a linear regression line to the log-log plot of R/S vs. n.
- Maximum Likelihood Estimation: A more sophisticated approach that considers the statistical properties of the data.
- Wavelet Analysis: A more advanced technique that addresses some limitations of R/S analysis related to non-stationarity.
The choice of estimation method depends on the specific characteristics of the data and the research question.
Frequently Asked Questions (FAQ):
-
What is the difference between R/S analysis and autocorrelation? Autocorrelation measures short-term correlations, while R/S analysis focuses on long-range dependencies.
-
Can R/S analysis be applied to non-time series data? While primarily used for time series, adaptations of R/S analysis can be applied to other data types with a notion of ordering.
-
How do I handle missing data in R/S analysis? Missing data can bias results. Imputation techniques (e.g., linear interpolation) can be used, but their impact should be carefully considered.
-
What are the limitations of using R/S analysis for financial markets? Market microstructure effects and infrequent trading can affect the accuracy of R/S analysis in finance.
-
Is R/S analysis suitable for short time series? No, shorter time series are less reliable for accurate Hurst exponent estimation.
-
What software packages can perform R/S analysis? Several statistical software packages, including R and Python (with libraries like
hurst
), can perform R/S analysis.
Practical Tips for Maximizing the Benefits of R/S Analysis:
- Ensure data quality: Clean and preprocess your data to remove outliers and handle missing values appropriately.
- Choose an appropriate estimation method: Select a method for estimating the Hurst exponent based on your data characteristics and the desired level of accuracy.
- Validate your results: Compare your findings with other statistical methods to ensure robustness and consistency.
- Consider the limitations: Be aware of the limitations of R/S analysis, particularly concerning data length and stationarity.
- Interpret results carefully: The Hurst exponent provides valuable insights, but avoid over-interpreting its values without considering the context of the data.
- Utilize appropriate software: Leverage statistical software packages designed for efficient and accurate R/S analysis.
- Understand the implications: Apply your understanding of the Hurst exponent to inform decision-making in your specific domain.
- Consult experts: Seek expert advice if you encounter challenges or uncertainties in applying R/S analysis to your data.
Conclusion:
Rescaled range analysis, with its ability to detect long-range dependencies and self-similarity, provides a powerful tool for understanding complex systems across diverse disciplines. By carefully considering its limitations and applying appropriate methodologies, researchers and practitioners can leverage R/S analysis to gain valuable insights, improve forecasts, and develop more effective strategies. The ongoing development of refined estimation methods and computational techniques continues to enhance the power and applicability of this valuable statistical tool. Its continued exploration will undoubtedly unlock further insights into the hidden patterns and intricate dynamics of the world around us.
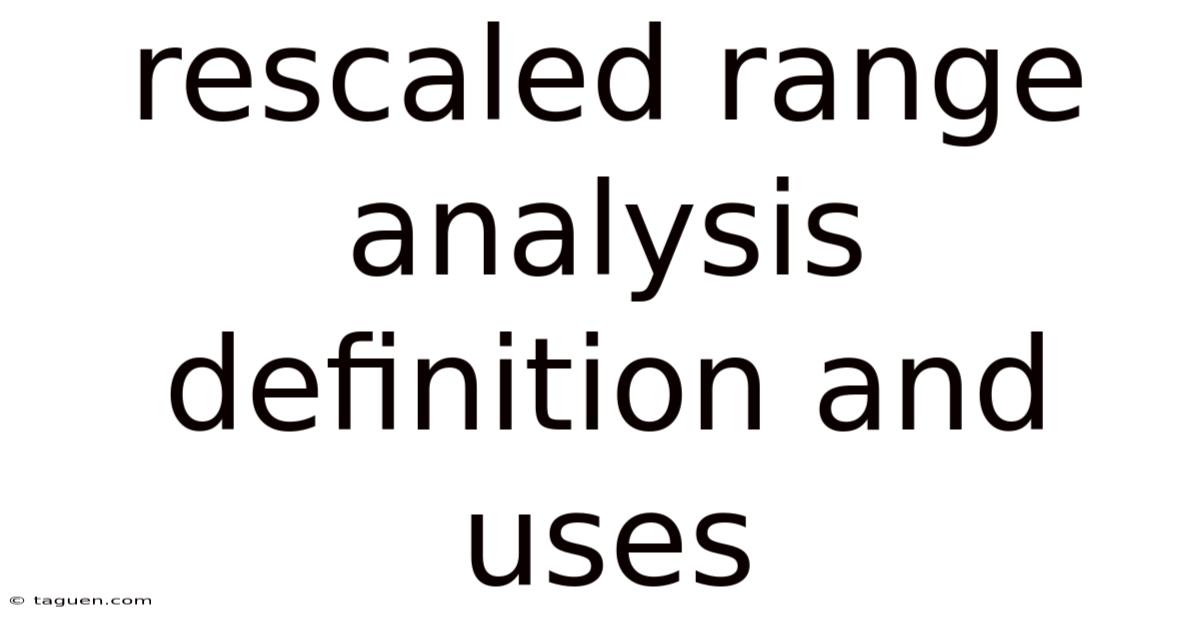
Thank you for visiting our website wich cover about Rescaled Range Analysis Definition And Uses. We hope the information provided has been useful to you. Feel free to contact us if you have any questions or need further assistance. See you next time and dont miss to bookmark.
Also read the following articles
Article Title | Date |
---|---|
How To Invest In Wine Stocks | Apr 23, 2025 |
What Stocks Should I Invest In 2018 | Apr 23, 2025 |
What Stocks To Invest In 2016 | Apr 23, 2025 |
How To Pay Off 100k In Student Loans | Apr 23, 2025 |
Why Are Ev Stocks Down | Apr 23, 2025 |