Multiple Discriminant Analysis Mda Definition How Its Used
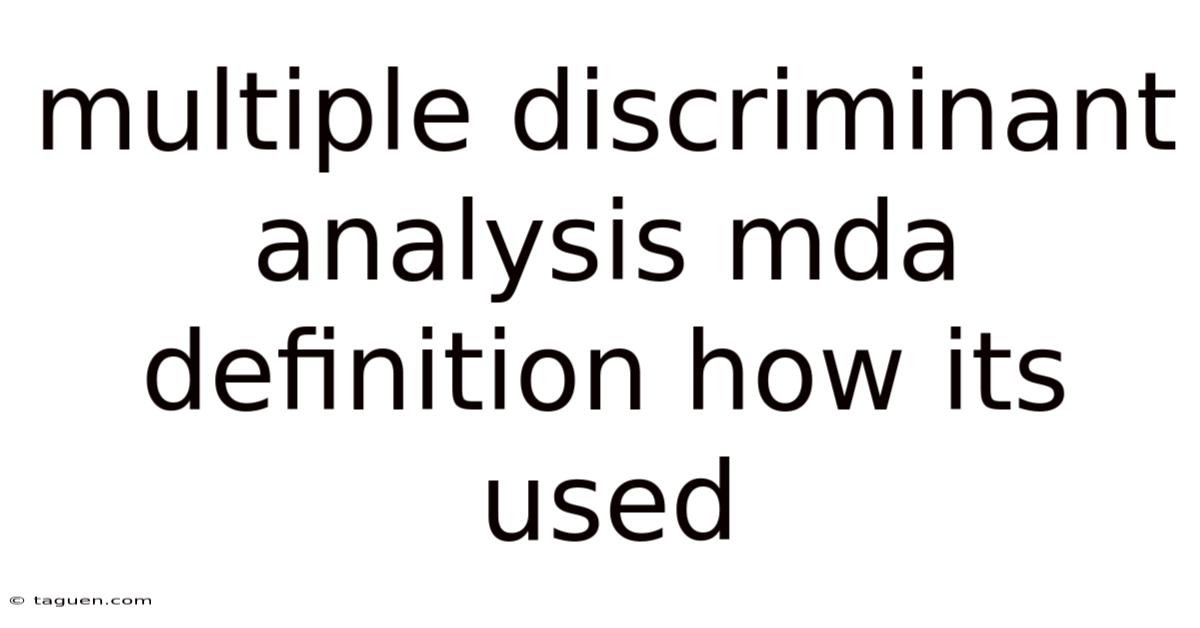
Discover more detailed and exciting information on our website. Click the link below to start your adventure: Visit Best Website meltwatermedia.ca. Don't miss out!
Table of Contents
Unlocking Insights: A Deep Dive into Multiple Discriminant Analysis (MDA)
What if the power to classify and predict complex datasets rested in the hands of a single, sophisticated statistical technique? Multiple Discriminant Analysis (MDA) offers just that, providing a robust framework for understanding and predicting group membership based on multiple predictor variables.
Editor’s Note: This article on Multiple Discriminant Analysis (MDA) provides a comprehensive overview of its definition, applications, and interpretations, drawing on the latest research and practical examples.
Multiple Discriminant Analysis (MDA), a multivariate statistical technique, is a powerful tool used to predict group membership based on several independent variables. Unlike simpler methods that focus on single predictors, MDA leverages the combined power of multiple variables to achieve more accurate and nuanced classifications. This makes it particularly valuable in situations where predicting group membership based on a single factor is unreliable or insufficient. Its applications span numerous fields, from marketing and finance to medicine and ecology, making it a crucial technique for data analysts and researchers alike.
Understanding the Core of MDA: Key Takeaways
This article will delve into the core aspects of MDA, exploring its underlying principles, its application across various industries, common challenges encountered, its role in fostering innovation, and its relationship with other statistical methods. We will provide practical examples, illustrative case studies, and a structured approach to understanding its interpretation. By the end, readers will have a solid grasp of MDA's capabilities and limitations, empowering them to effectively utilize this powerful analytical tool.
Key Takeaway | Description |
---|---|
MDA's Purpose: Prediction of Group Membership | Predicts the probability of an observation belonging to a specific group based on multiple predictor variables. |
Multivariate Nature: Multiple Predictors | Utilizes multiple independent variables simultaneously to improve predictive accuracy. |
Discriminant Functions: Dimensionality Reduction | Creates linear combinations of predictors (discriminant functions) to maximize group separation. |
Classification Accuracy: Evaluation Metric | Assesses the accuracy of the model in correctly classifying observations into their respective groups. |
Applications: Diverse Fields | Used across various disciplines, including marketing, finance, medicine, and ecology. |
Interpretation: Understanding Discriminant Functions | Interpreting the weights associated with each predictor in the discriminant functions reveals variable importance. |
From Theory to Practice: Defining and Applying MDA
MDA's foundation lies in its ability to discriminate between different groups based on a set of measured variables. Imagine you're a marketing analyst trying to segment customers into high-value, medium-value, and low-value groups based on their purchasing behavior, demographics, and website activity. MDA can help you identify the specific combinations of these variables that best differentiate these customer segments.
Definition and Core Concepts: At its core, MDA aims to find linear combinations of the predictor variables (also known as discriminant functions) that best separate the different groups. These functions are constructed to maximize the variance between groups while minimizing the variance within groups. The resulting discriminant functions can then be used to classify new observations into their most likely group. The number of discriminant functions is limited by the smaller of (number of groups - 1) and (number of predictors).
Applications Across Industries: MDA's versatility is evident in its wide range of applications:
- Marketing: Customer segmentation, market research, brand positioning.
- Finance: Credit risk assessment, fraud detection, investment portfolio management.
- Medicine: Disease diagnosis, patient classification, clinical trial analysis.
- Ecology: Species identification, habitat classification, environmental monitoring.
- Psychology: Personality assessment, psychological profiling, clinical diagnosis.
Challenges and Solutions: While MDA is a powerful technique, certain challenges need to be addressed:
- Multicollinearity: High correlation between predictor variables can affect the stability and interpretation of the discriminant functions. Techniques like principal component analysis (PCA) can help mitigate this issue.
- Violation of Assumptions: MDA assumes multivariate normality, homogeneity of variance-covariance matrices, and linearity. Violations of these assumptions can lead to inaccurate results. Transformations of variables or alternative methods might be necessary.
- Sample Size: Sufficient sample size is crucial for reliable results, particularly when dealing with multiple groups and predictors.
- Interpretability: While MDA provides accurate classifications, interpreting the discriminant functions can sometimes be challenging. Careful consideration of the variable weights and their significance is necessary.
Impact on Innovation: MDA contributes to innovation by enabling more accurate predictions and classifications, leading to improved decision-making across various domains. For instance, in medicine, MDA can help develop more accurate diagnostic tools, while in marketing, it can facilitate targeted advertising campaigns. The ability to leverage multiple variables simultaneously drives efficiency and enhances the quality of insights gained from data analysis.
Exploring the Interplay: MDA and Other Statistical Methods
The relationship between MDA and other statistical methods is crucial for a comprehensive understanding of its place within the broader landscape of data analysis.
Relationship between PCA and MDA: Principal Component Analysis (PCA) and MDA are closely related. PCA is often used as a preprocessing step for MDA, particularly when dealing with multicollinearity among predictor variables. PCA reduces the dimensionality of the data by creating uncorrelated principal components, which can then be used as input for MDA. This reduces redundancy and improves the stability of the discriminant functions.
Roles and Real-World Examples: Consider a study on predicting customer churn in a telecom company. Numerous variables (usage patterns, demographics, contract type, customer service interactions) might influence churn. PCA could be used to reduce these variables into a smaller set of uncorrelated components, which would then be used as predictors in an MDA model to classify customers as likely to churn or not.
Risks and Mitigations: Over-reliance on PCA before MDA can lead to loss of interpretability. The principal components might not have direct intuitive meaning, making it difficult to understand which original variables contributed most to the classification. Careful selection of the number of principal components to retain is crucial.
Impact and Implications: Using PCA with MDA can enhance predictive accuracy and model robustness, but it requires careful consideration of the trade-off between dimensionality reduction and interpretability. Effective communication of results becomes paramount to ensure that stakeholders understand both the model's predictions and the variables that drive them.
Frequently Asked Questions about MDA
- What are the assumptions of MDA? MDA assumes multivariate normality, homogeneity of variance-covariance matrices across groups, and linearity between predictors and group membership.
- How do I interpret the discriminant functions? Examine the standardized coefficients (weights) associated with each predictor variable in the discriminant functions. Larger absolute values indicate greater importance in separating the groups.
- What is the difference between MDA and logistic regression? While both predict group membership, logistic regression is typically used for binary or binomial outcomes, whereas MDA can handle multiple groups.
- How do I assess the accuracy of an MDA model? Use metrics like classification accuracy, sensitivity, specificity, and the confusion matrix to evaluate the model's performance.
- What if I have unequal group sizes? Unequal group sizes are common. MDA can still be applied, but the results should be interpreted cautiously.
- What software packages can perform MDA? Several statistical software packages, including SPSS, SAS, R, and Python (with libraries like scikit-learn), can perform MDA.
Practical Tips for Maximizing MDA's Benefits
- Data Preparation: Thoroughly clean and prepare your data. Handle missing values appropriately and consider transforming variables to meet MDA's assumptions.
- Variable Selection: Carefully select relevant predictor variables. Avoid including too many irrelevant variables, as this can reduce the model's accuracy and interpretability.
- Assumption Checking: Assess whether your data meets MDA's assumptions. If violations occur, explore data transformations or alternative methods.
- Model Evaluation: Rigorously evaluate your model's performance using appropriate metrics. Consider cross-validation techniques to assess its generalizability.
- Interpretation: Carefully interpret the discriminant functions and their coefficients to understand the relationship between predictors and group membership.
- Visualization: Use visualizations (e.g., scatter plots, box plots) to explore the data and understand the separation between groups.
- Iterative Process: MDA is often an iterative process. Refine your model based on evaluation results and adjust variables or transformations as needed.
- Contextual Understanding: Always interpret the results within the context of the research question and the data being analyzed.
Conclusion: Unlocking the Predictive Power of MDA
Multiple Discriminant Analysis (MDA) offers a powerful and versatile tool for classifying observations into predefined groups based on multiple predictor variables. By understanding its principles, applications, and limitations, researchers and analysts can harness its potential to improve decision-making and unlock valuable insights from complex datasets. While challenges exist, careful consideration of data preparation, assumption checking, and model evaluation ensures accurate and reliable results, ultimately driving innovation and informed decision-making across a wide range of fields. The continued development and refinement of MDA will undoubtedly solidify its position as a cornerstone technique in multivariate statistical analysis.
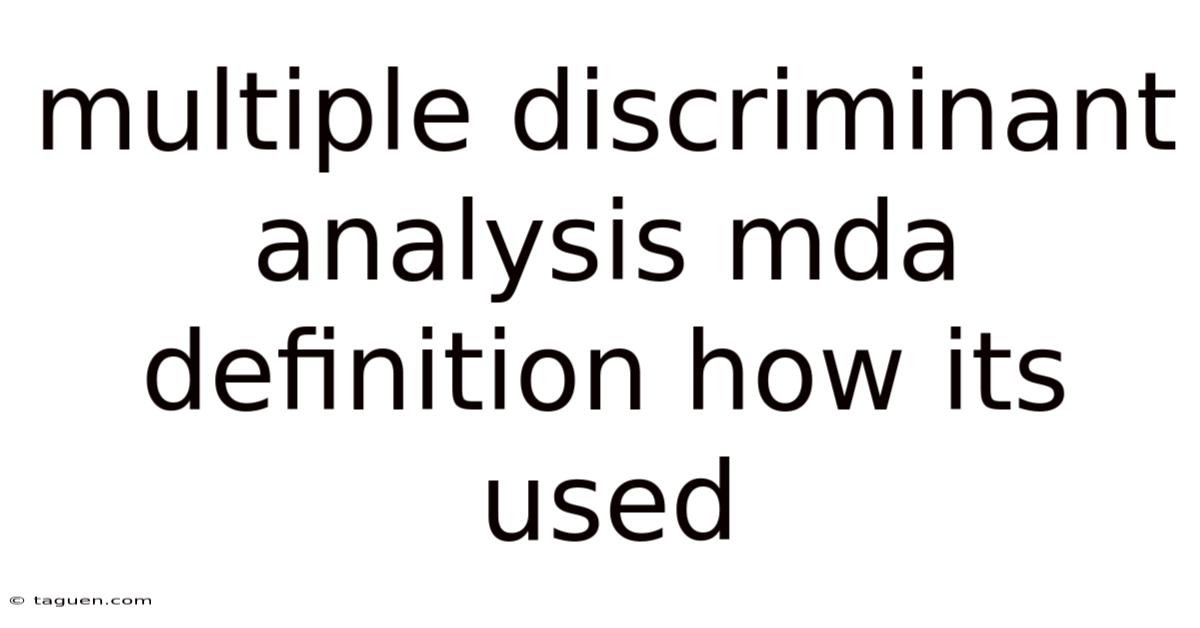
Thank you for visiting our website wich cover about Multiple Discriminant Analysis Mda Definition How Its Used. We hope the information provided has been useful to you. Feel free to contact us if you have any questions or need further assistance. See you next time and dont miss to bookmark.
Also read the following articles
Article Title | Date |
---|---|
Why Snapchat Turned Down Buyouts | Apr 17, 2025 |
Mutual Exclusion Doctrine Definition | Apr 17, 2025 |
Form 4506 Request For Copy Of Tax Return Definition And Filing | Apr 17, 2025 |
Morningstar Sustainability Rating Definition And How It Works | Apr 17, 2025 |
What Is Securities Trading | Apr 17, 2025 |