Multi Factor Model Definition And Formula For Comparing Factors
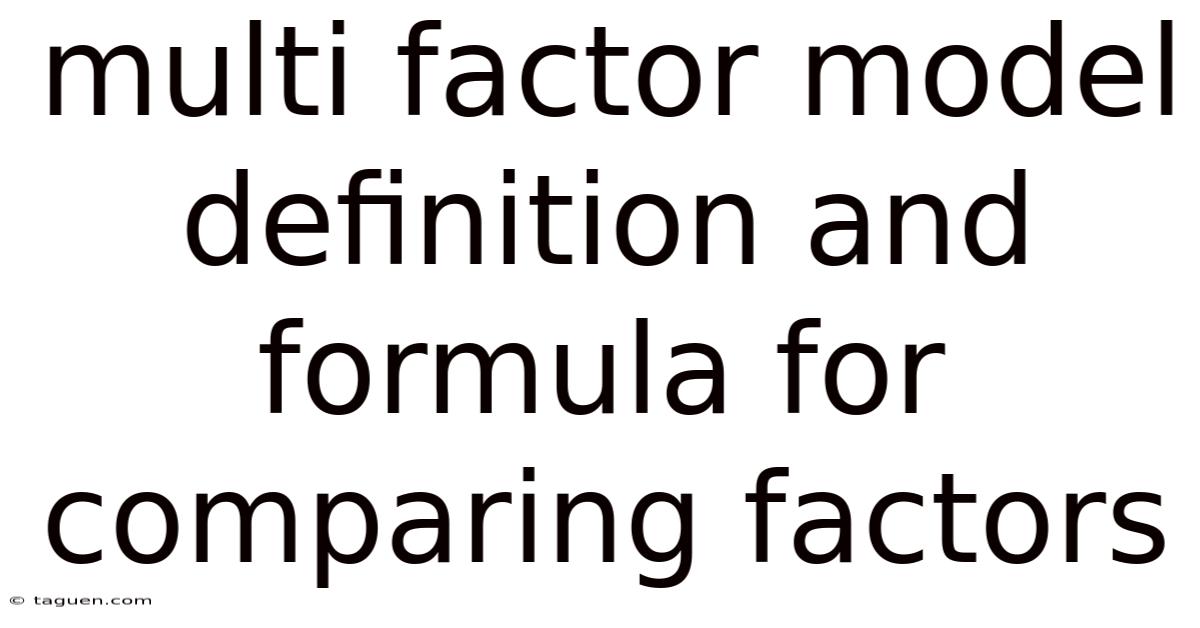
Discover more detailed and exciting information on our website. Click the link below to start your adventure: Visit Best Website meltwatermedia.ca. Don't miss out!
Table of Contents
Unveiling the Multi-Factor Model: A Deep Dive into Definition and Factor Comparison
What if a more comprehensive approach to investment analysis could unlock superior returns? Multi-factor models offer precisely that, moving beyond simplistic single-factor assessments to capture the nuanced interplay of market forces.
Editor’s Note: This article on multi-factor models provides a comprehensive overview of their definition, formulaic underpinnings, and methods for comparing different factors. It incorporates the latest research and real-world applications, ensuring readers gain a practical understanding of this crucial investment strategy.
Understanding multi-factor models is essential for navigating today's complex investment landscape. These models transcend the limitations of single-factor approaches (like the Capital Asset Pricing Model, or CAPM), offering a more nuanced and potentially more profitable perspective on asset pricing. Their applications span diverse investment strategies, from portfolio construction and risk management to performance attribution and alpha generation. This article will delve into the core concepts, practical applications, and challenges associated with multi-factor models, equipping readers with the knowledge to leverage their power effectively.
This article will explore the core aspects of multi-factor models, examining their definition, the formulas used for comparing factors, their real-world applications, and the challenges involved in their implementation. Backed by expert insights and data-driven research, it provides actionable knowledge for industry professionals and enthusiasts alike.
Defining Multi-Factor Models
A multi-factor model is a financial model that uses multiple macroeconomic and microeconomic variables to explain the returns of an asset or portfolio. Unlike single-factor models like the CAPM, which rely solely on market risk (beta), multi-factor models incorporate several factors believed to influence returns. These factors can be quantitative (e.g., value, size, momentum) or qualitative (e.g., management quality, industry trends). The core principle is that asset returns are driven by exposures to multiple systematic risk factors, not just market risk.
The general formula for a multi-factor model can be represented as:
R<sub>i</sub> = α<sub>i</sub> + β<sub>i1</sub>F<sub>1</sub> + β<sub>i2</sub>F<sub>2</sub> + ... + β<sub>in</sub>F<sub>n</sub> + ε<sub>i</sub>
Where:
- R<sub>i</sub> represents the return of asset i.
- α<sub>i</sub> is the asset's alpha, representing the portion of return not explained by the factors. A positive alpha suggests outperformance.
- β<sub>ij</sub> represents the sensitivity (beta) of asset i to factor j. This shows how much the asset's return is expected to change for a one-unit change in the factor.
- F<sub>j</sub> represents the return of factor j.
- ε<sub>i</sub> represents the asset's specific or idiosyncratic risk – the portion of return unexplained by the model.
Popular Multi-Factor Models and Their Factors
Several prominent multi-factor models exist, each emphasizing different factors:
-
Fama-French Three-Factor Model: This model expands on the CAPM by adding size (SMB – Small Minus Big) and value (HML – High Minus Low) factors. SMB captures the tendency of smaller companies to outperform larger ones, while HML reflects the outperformance of value stocks (high book-to-market ratio) over growth stocks (low book-to-market ratio).
-
Carhart Four-Factor Model: Builds upon the Fama-French model by adding a momentum factor (UMD – Up Minus Down). Momentum captures the tendency of stocks that have performed well recently to continue performing well.
-
Barra Model: A proprietary model used extensively in portfolio management, it incorporates numerous factors including size, value, volatility, liquidity, and industry effects.
-
Fundamental Law of Active Management: This model highlights the importance of information ratio in active portfolio management. It's not a specific model with fixed factors but provides a framework for evaluating the effectiveness of factor selection and portfolio construction.
Comparing Factors: Methods and Metrics
Comparing different factors within a multi-factor model requires careful consideration of several metrics:
-
Factor Return: This is the average return of a portfolio constructed to have high exposure to a specific factor. A higher factor return suggests a stronger factor.
-
Factor Risk: Measured by the standard deviation of the factor return, this indicates the volatility associated with exposure to the factor. Higher risk doesn’t automatically imply higher returns.
-
Information Ratio (IR): This crucial metric measures the risk-adjusted return of a factor. It's calculated as the average factor return divided by the factor's standard deviation. A higher IR implies a better risk-adjusted return.
-
Sharpe Ratio: Similar to the information ratio, it measures risk-adjusted return but uses the risk-free rate as a benchmark. It's calculated as (factor return - risk-free rate) / factor standard deviation.
-
Factor Correlation: Examining the correlation between different factors is crucial to understand diversification effects. High correlation suggests redundancy, while low correlation indicates diversification benefits.
-
Factor Timing and Selection: Sophisticated multi-factor models incorporate dynamic factor weighting, adjusting factor exposures based on market conditions and predicted future performance. Successful factor timing enhances performance.
Formula for Comparing Factor Returns:
A straightforward comparison of factor returns can be done using simple arithmetic averages over a specific period. For example, to compare the performance of the Size (SMB) and Value (HML) factors over the past five years, one would calculate the average annual return for each factor over that period.
Example: Let's say SMB averaged 5% annual return and HML averaged 7% over five years. This suggests HML had a superior return. However, risk must be incorporated for a complete comparison (using IR or Sharpe Ratio).
Formula for Comparing Factor Risk:
Factor risk, typically measured by standard deviation, reflects the volatility associated with each factor. A higher standard deviation indicates greater risk.
Example: If SMB had a standard deviation of 10% and HML had a standard deviation of 8%, HML exhibits lower risk.
Formulas for Information Ratio (IR) and Sharpe Ratio:
-
Information Ratio (IR): IR = (Average Factor Return) / (Standard Deviation of Factor Return)
-
Sharpe Ratio: Sharpe Ratio = (Average Factor Return - Risk-Free Rate) / (Standard Deviation of Factor Return)
Applications of Multi-Factor Models
Multi-factor models have wide-ranging applications in finance:
-
Portfolio Construction: These models help construct diversified portfolios tailored to specific risk and return objectives. By identifying factors with attractive risk-adjusted returns and low correlations, investors can build efficient portfolios.
-
Risk Management: Multi-factor models allow for a more precise assessment of portfolio risk by breaking it down into specific factor exposures. This facilitates better risk mitigation strategies.
-
Performance Attribution: Understanding the contribution of each factor to a portfolio's performance provides valuable insights into investment strategies. This helps identify successful and underperforming factors.
-
Alpha Generation: Identifying factors that are not fully priced by the market (i.e., offering a positive alpha) is a key goal of many quantitative investment strategies. Multi-factor models facilitate the discovery of such opportunities.
-
Security Selection: By analyzing a security's exposure to different factors, investors can make more informed decisions about its potential return and risk.
Challenges and Limitations
Despite their advantages, multi-factor models present some challenges:
-
Factor Selection: Choosing the right factors is crucial, and there is no universally agreed-upon set of factors. The optimal factor set may vary across markets and time periods.
-
Data Requirements: Implementing multi-factor models requires extensive historical data on asset returns and factor returns, which may not always be readily available or reliable.
-
Overfitting: Overfitting occurs when a model fits the historical data too closely, leading to poor out-of-sample performance. Careful model validation is necessary to mitigate this risk.
-
Factor Persistence: The persistence of factor premiums (excess returns) over time is not guaranteed. Factors that have performed well historically may not continue to do so in the future.
-
Transaction Costs: Implementing dynamic factor weighting strategies can lead to significant transaction costs, potentially offsetting potential gains.
Relationship Between Factor Risk and Return
The relationship between factor risk and return is not always linear or predictable. While higher risk often implies higher potential returns, this is not a guaranteed outcome. Some factors may offer attractive risk-adjusted returns (high IR) even with moderate risk. Understanding this relationship requires a thorough analysis of historical data and market dynamics. It’s crucial to consider both return and risk simultaneously, using metrics like Information Ratio or Sharpe Ratio, to evaluate factor performance effectively.
Conclusion
Multi-factor models offer a powerful and comprehensive approach to investment analysis, moving beyond the limitations of single-factor models. By incorporating multiple factors that influence asset returns, these models provide a more nuanced understanding of risk and return. While challenges exist regarding factor selection, data requirements, and model validation, the potential benefits for portfolio construction, risk management, and alpha generation make them an invaluable tool for sophisticated investors. Careful selection and monitoring of factors, combined with rigorous model testing, are essential for successful implementation. The continuous evolution of multi-factor models and the emergence of new factors underscores their ongoing relevance in the dynamic world of finance.
Further Analysis: Deep Dive into Factor Risk
Factor risk is a critical component of multi-factor models. It represents the volatility associated with exposure to a specific factor. Understanding factor risk is essential for building well-diversified portfolios and managing risk effectively. High factor risk doesn't automatically imply high returns, and some low-risk factors might offer attractive risk-adjusted returns.
We can analyze factor risk through several methods:
-
Standard Deviation: The most common measure of factor risk, representing the dispersion of factor returns around their average. A higher standard deviation indicates greater volatility and higher risk.
-
Value at Risk (VaR): Estimates the maximum possible loss in a portfolio's value over a specific period with a given confidence level. This provides a more risk-averse measure compared to standard deviation.
-
Conditional Value at Risk (CVaR or Expected Shortfall): Measures the expected loss in the tail of the distribution, providing a more comprehensive risk assessment than VaR.
-
Stress Testing: Simulating various adverse market scenarios to assess the impact on the factor’s return, providing a more qualitative view of the factor risk.
A table illustrating different factors and their risk characteristics would be very beneficial here, but the data would be hypothetical since it requires specific market data and would change rapidly.
Frequently Asked Questions (FAQs)
1. What is the difference between a single-factor and a multi-factor model?
A single-factor model, like the CAPM, considers only one factor (market risk) to explain asset returns. Multi-factor models incorporate multiple factors, providing a more comprehensive analysis.
2. How do I choose the right factors for my multi-factor model?
Factor selection depends on your investment objectives, market conditions, and risk tolerance. Consider factors with historical evidence of providing excess returns and low correlation with other factors.
3. What are the limitations of using multi-factor models?
Limitations include data requirements, overfitting risk, factor persistence, and transaction costs.
4. How can I mitigate the risk of overfitting in my multi-factor model?
Use robust statistical methods, cross-validation techniques, and out-of-sample testing to prevent overfitting.
5. Are multi-factor models suitable for all types of investors?
Multi-factor models require a certain level of financial expertise and access to data. They may not be suitable for all investors.
6. How frequently should I rebalance my multi-factor portfolio?
Rebalancing frequency depends on your investment strategy and market conditions. Regular rebalancing can help maintain desired risk and return characteristics.
Practical Tips for Maximizing the Benefits of Multi-Factor Models
- Thoroughly research and select relevant factors: Base your selection on academic research, market trends, and your investment goals.
- Use robust statistical methods for factor analysis: Employ techniques that account for non-normality and potential biases in the data.
- Validate your model rigorously: Test your model on out-of-sample data to ensure it generalizes well beyond the historical period.
- Consider transaction costs: Factor in trading expenses when implementing dynamic factor weighting strategies.
- Monitor factor performance regularly: Track the performance of your chosen factors and adjust your strategy as needed.
- Diversify your factor exposures: Don't rely on a single factor; diversify across multiple factors with low correlation.
- Stay updated on research and market developments: New factors and insights are constantly emerging in the field of multi-factor investing.
- Use professional tools and software: Specialized software can significantly aid in the construction and analysis of multi-factor models.
End Note: The Future of Multi-Factor Investing
Multi-factor models are not a static concept. Ongoing research continues to refine factor identification, weighting strategies, and risk management techniques. The integration of alternative data sources, machine learning, and artificial intelligence promises to further enhance the power and effectiveness of multi-factor investing. By embracing innovation and adaptation, investors can harness the full potential of these advanced models to achieve superior risk-adjusted returns in the ever-evolving financial markets.
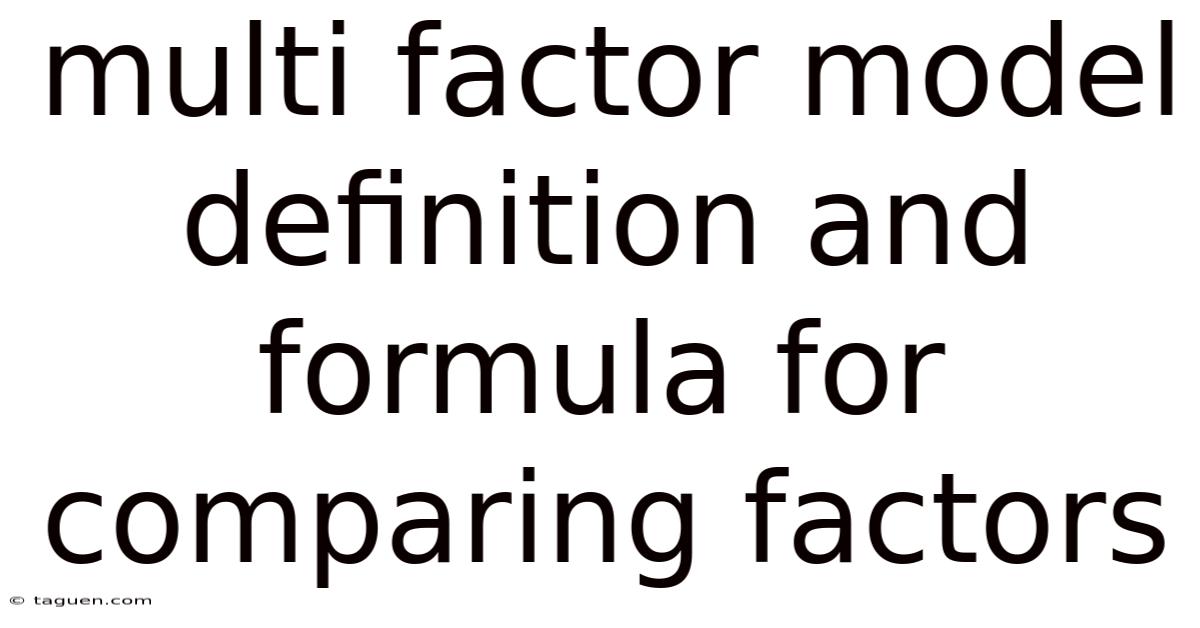
Thank you for visiting our website wich cover about Multi Factor Model Definition And Formula For Comparing Factors. We hope the information provided has been useful to you. Feel free to contact us if you have any questions or need further assistance. See you next time and dont miss to bookmark.
Also read the following articles
Article Title | Date |
---|---|
Mutual Exclusion Doctrine Definition | Apr 17, 2025 |
What Is Momentum Definition In Trading Tools And Risks | Apr 17, 2025 |
Monetary Base Definition What It Includes Example | Apr 17, 2025 |
What Is Another Name For Balance Sheet | Apr 17, 2025 |
What Is A Balance Sheet Loan | Apr 17, 2025 |