Model Risk Definition Management And Examples
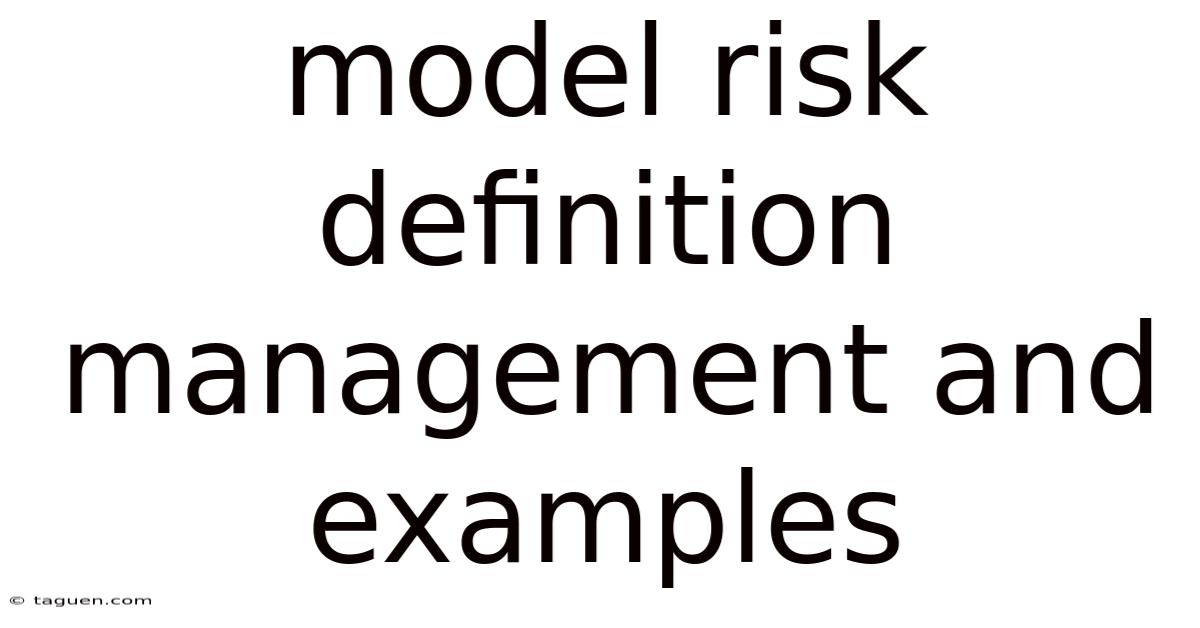
Discover more detailed and exciting information on our website. Click the link below to start your adventure: Visit Best Website meltwatermedia.ca. Don't miss out!
Table of Contents
Unveiling Model Risk: Definition, Management, and Real-World Examples
What if the stability of your entire financial institution hinges on the accuracy of complex mathematical models? This isn't a hypothetical scenario; it's the critical reality of model risk, a pervasive challenge demanding robust management strategies.
Editor’s Note: This article on model risk definition, management, and examples has been published today, ensuring the latest insights and expert analysis. We've incorporated real-world case studies and best practices to provide a comprehensive understanding of this crucial topic.
Model risk, in its simplest form, is the potential for loss resulting from the use of inaccurate, incomplete, or improperly applied models. These models, often sophisticated mathematical constructs, are employed across numerous industries, particularly in finance, to make critical decisions affecting everything from pricing and risk assessment to regulatory compliance and strategic planning. Understanding model risk and implementing effective management strategies is paramount for maintaining financial stability and operational integrity.
This article delves into the core aspects of model risk, examining its definition, real-world applications, challenges, mitigation strategies, and the crucial interplay between model risk and other forms of operational risk. Backed by expert insights and data-driven research, it provides actionable knowledge for risk managers, financial professionals, and anyone involved in the development or application of quantitative models.
Key Takeaways: This article will explore the following key areas:
Key Area | Description |
---|---|
Defining Model Risk | A precise definition and breakdown of the different facets of model risk. |
Model Risk Types & Sources | Categorization of model risk based on origin and impact, including model specification, implementation, and use errors. |
Real-World Examples | Case studies illustrating the devastating consequences of inadequate model risk management. |
Model Risk Management Framework | A detailed examination of a robust model risk management (MRM) framework, encompassing governance, development, validation, and monitoring. |
Model Risk and Operational Risk | Exploring the close relationship between model risk and broader operational risks. |
Mitigation Strategies | Practical strategies for identifying, assessing, mitigating, and monitoring model risk. |
The Future of Model Risk Management | Emerging challenges and trends shaping the future of MRM in an increasingly complex and data-driven world. |
With a strong understanding of its relevance, let’s explore model risk further, uncovering its applications, challenges, and future implications.
Defining Model Risk and its Core Concepts
Model risk encompasses the uncertainty associated with the use of models. This uncertainty arises from various sources, including:
-
Model Specification Error: This occurs when the underlying assumptions of the model do not accurately reflect the real-world phenomenon it aims to represent. For instance, a credit scoring model that fails to account for changes in macroeconomic conditions can lead to inaccurate risk assessments.
-
Model Implementation Error: This refers to errors that occur during the process of translating the model's theoretical framework into a working computer program. Coding errors, data input errors, or incorrect parameterization can all contribute to implementation error.
-
Model Use Error: This involves misapplication of the model, such as using it outside its intended scope or relying on its outputs without sufficient understanding of its limitations. For example, using a model calibrated on historical data to predict future events under significantly different conditions can be a major source of error.
-
Data Error: Inaccurate or incomplete data used to build or calibrate the model can severely undermine its reliability and lead to biased or misleading results.
-
External Factors: Changes in market conditions, regulatory environments, or other external factors not considered during model development can also invalidate a model's outputs.
Applications Across Industries
While prevalent in finance, model risk extends beyond the financial services sector. Industries utilizing quantitative models for decision-making, such as insurance, healthcare, energy, and even retail, are equally susceptible. Examples include:
-
Financial Services: Credit scoring, fraud detection, risk management (market, credit, operational), pricing derivatives, portfolio optimization, and algorithmic trading.
-
Insurance: Actuarial modeling, claims processing, fraud detection, pricing insurance products.
-
Healthcare: Predictive modeling for disease outbreaks, personalized medicine, treatment optimization.
-
Energy: Forecasting energy demand, optimizing energy grids, risk assessment for renewable energy projects.
-
Retail: Customer segmentation, personalized recommendations, inventory management, fraud detection.
Challenges and Solutions in Model Risk Management
Effective model risk management faces several key challenges:
-
Model Complexity: Advanced models can be extremely complex, making it difficult to understand their internal workings and identify potential weaknesses.
-
Data Limitations: The quality, quantity, and availability of data can be significant constraints. Lack of sufficient historical data, biases in data collection, or changes in data characteristics can undermine model accuracy.
-
Evolving Regulatory Landscape: The regulatory environment surrounding model risk is constantly evolving, requiring continuous adaptation and compliance efforts.
-
Lack of Expertise: A shortage of skilled professionals with the necessary expertise in both quantitative modeling and risk management is a common challenge.
-
Communication and Collaboration: Effective communication and collaboration among model developers, validators, users, and risk managers are crucial for successful model risk management.
To address these challenges, robust model risk management frameworks are essential. These frameworks typically include:
-
Model Inventory: A comprehensive catalog of all models used within an organization.
-
Model Governance: Clear responsibilities, oversight, and approval processes for model development, validation, and use.
-
Model Development: Rigorous methodologies for model design, calibration, and testing.
-
Model Validation: Independent assessment of model accuracy, reliability, and suitability for its intended use.
-
Model Monitoring: Ongoing surveillance of model performance and identification of potential issues.
-
Incident Management: Processes for handling model-related incidents and investigating their root causes.
Model Risk and Operational Risk
Model risk is intrinsically linked to operational risk. Operational risk encompasses the risk of losses resulting from inadequate or failed internal processes, people, and systems or from external events. Model risk is a significant subset of operational risk, as model failures can directly lead to operational losses.
Real-World Examples of Model Risk Failures
Several high-profile incidents highlight the devastating consequences of inadequate model risk management:
-
The 2008 Financial Crisis: The widespread use of complex financial models, particularly in the securitization of mortgages, played a significant role in the crisis. These models often failed to accurately capture the risks associated with subprime lending, leading to widespread losses.
-
The London Whale Trading Losses: JPMorgan Chase's "London Whale" trading losses in 2012 resulted partly from flawed models used to assess the risks associated with complex derivatives trades.
-
Algorithmic Trading Errors: Errors in algorithmic trading systems, often stemming from model failures, can lead to significant financial losses in a short period.
Mitigation Strategies for Model Risk
Several key strategies can be implemented to mitigate model risk:
-
Independent Model Validation: This involves the independent review and assessment of models by a separate team to verify their accuracy, reliability, and suitability.
-
Robust Data Management: Implementing robust data management procedures, including data quality checks, data cleansing, and data governance, is crucial.
-
Stress Testing and Scenario Analysis: Testing models under various stress scenarios to assess their resilience and identify potential vulnerabilities.
-
Regular Model Monitoring: Continuous monitoring of model performance and identification of any deviations from expected behavior.
-
Comprehensive Documentation: Maintaining detailed documentation of the model's development, assumptions, limitations, and validation results.
-
Model Version Control: Tracking changes made to models and maintaining versions to facilitate auditing and analysis.
-
Training and Education: Providing training and education to model developers, validators, and users to enhance their understanding of model risk.
The Future of Model Risk Management
The future of model risk management will likely be shaped by several key trends:
-
Increased Regulation: Expect stricter regulatory requirements and greater scrutiny of model risk management practices.
-
Advancements in Artificial Intelligence (AI) and Machine Learning (ML): The increasing use of AI and ML in model development will present new challenges and opportunities in model risk management.
-
Explainable AI (XAI): A growing focus on developing models that are more transparent and easier to understand will improve model risk management.
-
Big Data Analytics: Leveraging big data analytics to improve data quality and enhance model accuracy.
-
Cloud Computing: The use of cloud computing for model development and deployment will require new approaches to model risk management.
Further Analysis: Deep Dive into Model Validation
Model validation is a critical component of effective model risk management. A robust validation process should encompass:
-
Review of Model Documentation: A thorough examination of the model's specifications, assumptions, and limitations.
-
Data Analysis: Assessment of data quality, completeness, and relevance.
-
Testing: Verification of model accuracy, stability, and performance using various testing methods, including backtesting, out-of-sample testing, and stress testing.
-
Sensitivity Analysis: Analyzing the impact of changes in model inputs on model outputs.
-
Benchmarking: Comparing model performance against alternative models or industry benchmarks.
Frequently Asked Questions (FAQs) About Model Risk
Q1: What is the difference between model risk and operational risk? Model risk is a subset of operational risk, specifically the risk of losses associated with the use of inaccurate, incomplete, or improperly applied models.
Q2: Who is responsible for model risk management? Model risk management typically involves a collaborative effort among model developers, validators, users, and senior management.
Q3: How can I identify potential model risks? Potential model risks can be identified through model documentation review, data analysis, testing, stress testing, and ongoing monitoring.
Q4: What are the consequences of inadequate model risk management? Inadequate model risk management can lead to financial losses, regulatory penalties, reputational damage, and even legal action.
Q5: What is the role of independent model validation? Independent model validation provides an objective assessment of model accuracy, reliability, and suitability for its intended use.
Q6: How often should models be validated? The frequency of model validation depends on several factors, including the complexity of the model, the volatility of the data, and regulatory requirements. Regular reviews, often annual, are common, but high-risk models may require more frequent validation.
Practical Tips for Maximizing the Benefits of Effective Model Risk Management
-
Establish a robust model governance framework: Define clear roles, responsibilities, and approval processes for model development, validation, and use.
-
Implement rigorous model development methodologies: Employ structured approaches to model development, including detailed documentation and testing procedures.
-
Conduct thorough model validation: Ensure independent validation of all models to verify their accuracy, reliability, and suitability.
-
Establish a comprehensive model inventory: Maintain a detailed inventory of all models used within the organization.
-
Develop effective model monitoring procedures: Implement procedures to monitor model performance and identify potential issues.
-
Invest in training and education: Provide training to model developers, validators, and users to enhance their understanding of model risk.
-
Embrace technology: Utilize advanced technologies, such as AI and big data analytics, to enhance model development, validation, and monitoring.
-
Foster a culture of risk awareness: Encourage open communication and collaboration among all stakeholders to promote a culture of risk awareness.
Conclusion: Harnessing the Power of Models While Mitigating Risk
Model risk is an inherent aspect of using quantitative models for decision-making. However, by implementing a robust model risk management framework, organizations can harness the power of models while mitigating the potential for significant losses. Understanding model risk, fostering a culture of risk awareness, and embracing technological advancements are key to navigating the complexities of a data-driven world and ensuring long-term financial stability. The future of model risk management will be marked by increased sophistication, regulatory scrutiny, and a continued emphasis on transparency and explainability. By proactively addressing these challenges, organizations can unlock the immense potential of quantitative models while mitigating the risks inherent in their use.
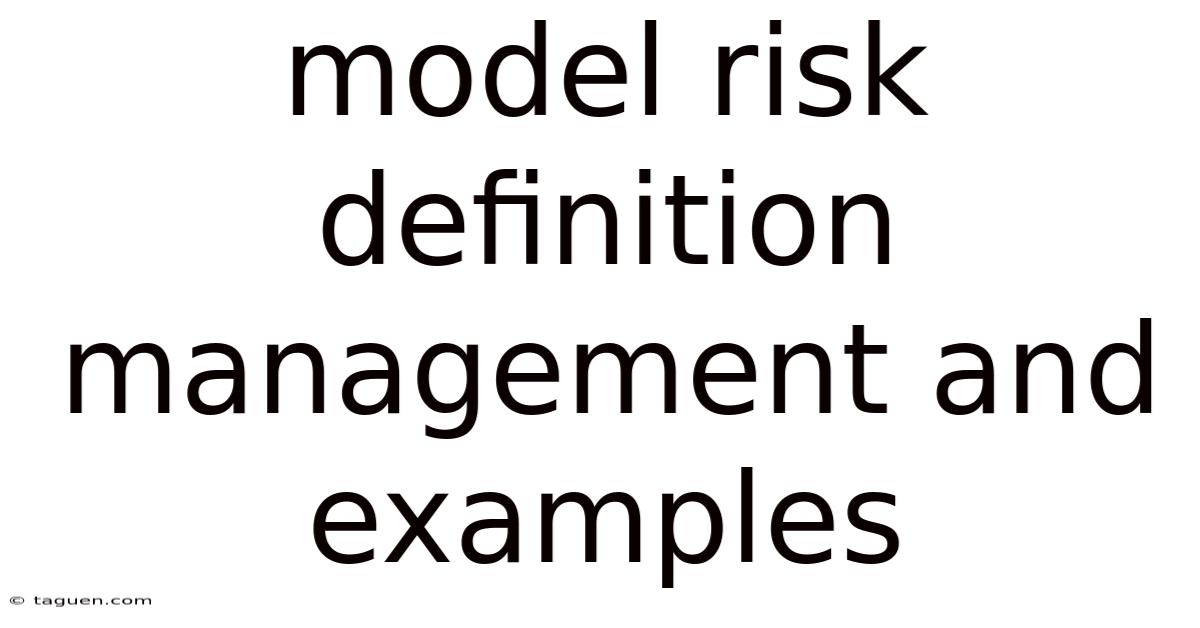
Thank you for visiting our website wich cover about Model Risk Definition Management And Examples. We hope the information provided has been useful to you. Feel free to contact us if you have any questions or need further assistance. See you next time and dont miss to bookmark.
Also read the following articles
Article Title | Date |
---|---|
Mississippi Company Definition | Apr 17, 2025 |
What Terms Commonly Used Under Ifrs Are Synonymous With Common Stock On The Balance Sheet | Apr 17, 2025 |
Foreign Bank Branch Definition Example Vs Subsidiary | Apr 17, 2025 |
Money Market Yield Definition Calculation And Example | Apr 17, 2025 |
National Market System Plan Nmsp Definition | Apr 17, 2025 |