Longitudinal Data Definition And Uses In Finance And Economics
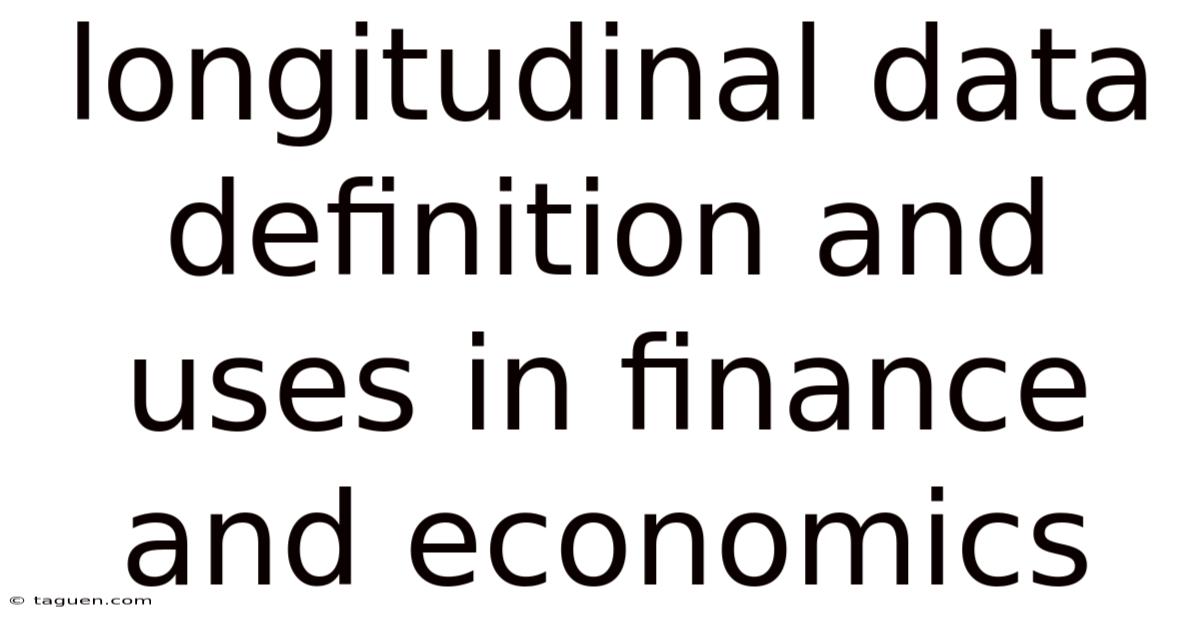
Discover more detailed and exciting information on our website. Click the link below to start your adventure: Visit Best Website meltwatermedia.ca. Don't miss out!
Table of Contents
Unlocking the Power of Time: Longitudinal Data in Finance and Economics
What if understanding the evolution of economic and financial variables over time unlocked unprecedented predictive power? Longitudinal data, with its unique capacity to track changes, is revolutionizing these fields, revealing intricate patterns and driving more informed decision-making.
Editor’s Note: This article on longitudinal data in finance and economics has been published today, ensuring the latest insights and expert analysis.
Longitudinal data offers a powerful lens through which to analyze economic and financial phenomena. Unlike cross-sectional data, which captures information at a single point in time, longitudinal data follows the same individuals, firms, or other units of observation over an extended period. This allows researchers to observe changes, trends, and relationships that would be impossible to detect using static snapshots. Its applications span a vast range, from evaluating the effectiveness of financial policies to predicting market behavior and assessing the impact of economic shocks.
Understanding longitudinal data is essential for staying ahead in today’s rapidly evolving economic and financial landscape. Its applications range from improving investment strategies and risk management to informing macroeconomic policy and understanding consumer behavior. This article delves into the core aspects of longitudinal data, examining its definition, real-world applications in finance and economics, challenges, and future potential. Backed by expert insights and data-driven research, it provides actionable knowledge for industry professionals and enthusiasts alike.
This article is the result of meticulous research, incorporating perspectives from leading econometricians, financial analysts, and real-world case studies from reputable academic journals and financial publications to ensure accuracy and reliability.
Key Takeaways:
Feature | Description |
---|---|
Definition | Tracking the same subjects over time, revealing changes and relationships. |
Financial Uses | Portfolio performance analysis, risk management, credit scoring, market prediction, fraud detection. |
Economic Uses | Impact evaluation of policies, understanding income inequality, analyzing consumer behavior, macroeconomic modeling. |
Challenges | Data collection difficulties, attrition bias, complex statistical methods. |
Benefits | Superior insights, improved predictions, more robust causal inference. |
With a strong understanding of its relevance, let’s explore longitudinal data further, uncovering its applications, challenges, and future implications.
Defining Longitudinal Data: A Deeper Dive
Longitudinal data, also known as panel data, involves repeated observations of the same variables on the same subjects over a defined period. This contrasts with cross-sectional data, which provides a snapshot at a single point in time, and time-series data, which tracks a single variable over time. The key characteristic of longitudinal data is its ability to capture both within-subject variation (changes within an individual over time) and between-subject variation (differences between individuals at any given time).
The structure of longitudinal data typically involves a data matrix where rows represent the subjects and columns represent the variables measured at different time points. For instance, a study tracking the investment portfolios of 100 individuals over five years would have 500 rows (100 individuals x 5 years) and columns representing variables like portfolio value, asset allocation, and risk tolerance at each time point.
Applications of Longitudinal Data in Finance
The use of longitudinal data in finance is widespread and offers numerous advantages over traditional cross-sectional analyses. Here are some key applications:
-
Portfolio Performance Analysis: Longitudinal data allows for a comprehensive assessment of portfolio performance over time, considering factors like asset allocation, risk tolerance, and market conditions. Researchers can identify which investment strategies consistently outperform others and how portfolio performance changes across different economic cycles.
-
Risk Management: By tracking changes in individual investor behavior and market trends over time, financial institutions can better assess and manage risk. Longitudinal data helps predict potential losses, optimize risk-adjusted returns, and design more robust risk mitigation strategies.
-
Credit Scoring: Longitudinal credit history data provides a more accurate assessment of creditworthiness compared to static credit reports. Lenders can use this data to predict default probabilities, tailor credit offerings, and minimize losses.
-
Market Prediction: Analysis of longitudinal data on market indicators, investor sentiment, and economic conditions can aid in forecasting market trends and predicting future price movements. This is particularly useful for algorithmic trading and investment strategy optimization.
-
Fraud Detection: Tracking transactions and account activity over time can help identify patterns indicative of fraudulent behavior. Longitudinal data analysis allows for early detection of anomalies and the development of more sophisticated fraud prevention systems.
Applications of Longitudinal Data in Economics
In economics, longitudinal data provides invaluable insights into various aspects of economic behavior and policy effectiveness. Some key applications include:
-
Impact Evaluation of Policies: Longitudinal data allows economists to assess the long-term effects of economic policies and interventions. For example, researchers can use panel data to evaluate the impact of a tax reform on income inequality or the effect of a new education program on future earnings.
-
Understanding Income Inequality: By tracking individual incomes over time, researchers can gain a deeper understanding of the dynamics of income inequality, identifying factors contributing to persistent income gaps and assessing the effectiveness of policies aimed at reducing inequality.
-
Analyzing Consumer Behavior: Longitudinal data on consumer spending, saving, and borrowing behavior provides insights into consumer preferences, purchasing patterns, and the impact of economic shocks on consumer spending. This information is crucial for businesses in market segmentation and product development.
-
Macroeconomic Modeling: Longitudinal data is essential for building sophisticated macroeconomic models that capture the complex interactions between economic variables over time. These models can be used to forecast economic growth, inflation, and other macroeconomic indicators.
-
Labor Economics: Longitudinal studies track individuals' employment, wages, and job transitions over time, revealing insights into labor market dynamics, the impact of education and training on career trajectories, and the effectiveness of labor market policies.
Challenges in Utilizing Longitudinal Data
While longitudinal data offers numerous advantages, it also presents several challenges:
-
Data Collection Difficulties: Gathering longitudinal data is often more expensive and time-consuming than collecting cross-sectional data. Maintaining consistent data collection methods over long periods is crucial to ensure data quality. Attrition, or the loss of participants over time, is a significant concern.
-
Attrition Bias: Attrition can lead to biased results if the participants who drop out differ systematically from those who remain in the study. This bias needs to be addressed through appropriate statistical techniques.
-
Complex Statistical Methods: Analyzing longitudinal data often requires specialized statistical methods, such as panel data regression, to account for the correlation between observations within the same subject over time. Researchers need expertise in econometrics and statistical modeling.
The Relationship Between Causality and Longitudinal Data
One of the most significant advantages of longitudinal data is its ability to improve causal inference. While correlation does not imply causation, longitudinal data helps researchers disentangle correlation from causation by controlling for time-invariant factors and observing changes over time. This allows for a more robust assessment of the causal effect of one variable on another. For example, a longitudinal study might track the impact of a specific financial intervention on household savings, controlling for other factors like income changes and household demographics. Observing changes over time helps isolate the effect of the intervention.
Longitudinal Data and Predictive Modeling
Longitudinal data is particularly valuable in predictive modeling. By incorporating temporal dynamics, models built on longitudinal data often yield more accurate and reliable predictions than models based on cross-sectional data alone. This is evident in financial forecasting, where models that incorporate time-series and panel data provide better predictions of market movements, portfolio returns, and credit risk.
Six Frequently Asked Questions About Longitudinal Data
-
Q: What is the difference between longitudinal and time-series data? A: Longitudinal data tracks multiple subjects over time, while time-series data focuses on a single subject (e.g., a stock market index) over time.
-
Q: What are the main statistical methods used for analyzing longitudinal data? A: Common methods include panel data regression, generalized estimating equations (GEE), and mixed-effects models.
-
Q: How do I deal with missing data in longitudinal datasets? A: Techniques include imputation (filling in missing values), weighting adjustments, and model specification that accounts for missing data patterns.
-
Q: What are the ethical considerations involved in collecting longitudinal data? A: Researchers must ensure informed consent, data privacy, and data security.
-
Q: What software packages can be used to analyze longitudinal data? A: Popular options include Stata, R, SAS, and SPSS.
-
Q: Can longitudinal data be used for causal inference? A: Yes, but careful consideration of potential confounding variables and appropriate statistical methods are crucial for establishing causality.
Practical Tips for Maximizing the Benefits of Longitudinal Data
-
Careful Planning: Meticulously plan the study design, ensuring consistent data collection methods over time.
-
Data Quality Control: Implement rigorous data quality control measures to minimize errors and inconsistencies.
-
Attrition Management: Develop strategies to minimize attrition and address potential bias resulting from participant dropout.
-
Appropriate Statistical Methods: Select statistical methods appropriate for the data structure and research questions.
-
Interpretation of Results: Carefully interpret the results, considering limitations of the data and methods used.
-
Transparency and Reproducibility: Document the data collection, analysis, and interpretation processes thoroughly to ensure transparency and reproducibility.
-
Collaboration: Collaborate with experts in econometrics and statistics to ensure appropriate data analysis and interpretation.
-
Ethical Considerations: Prioritize ethical considerations throughout the research process.
Conclusion: The Enduring Power of Longitudinal Data
Longitudinal data, with its ability to reveal temporal dynamics and enhance causal inference, represents a transformative force in finance and economics. By addressing the challenges and leveraging the opportunities it presents, researchers and practitioners can unlock unprecedented insights and build more robust models for prediction and decision-making. The future of these fields hinges on the continued development and application of sophisticated longitudinal data analysis techniques. The ability to track and understand change over time is not merely a methodological advancement but a fundamental shift in how we understand and interact with complex economic and financial systems.
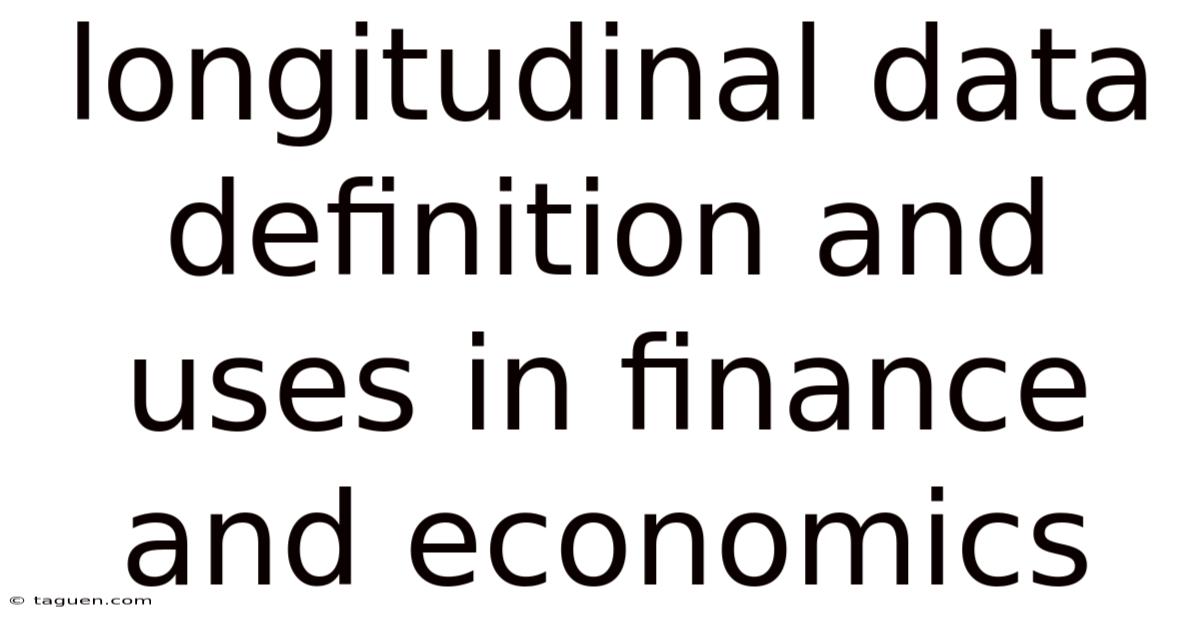
Thank you for visiting our website wich cover about Longitudinal Data Definition And Uses In Finance And Economics. We hope the information provided has been useful to you. Feel free to contact us if you have any questions or need further assistance. See you next time and dont miss to bookmark.
Also read the following articles
Article Title | Date |
---|---|
Which Behavioral Factor Influences Auto Insurance Premiums | Apr 15, 2025 |
How To Calculate Business Cash Flow | Apr 15, 2025 |
Credit Reversal Meaning In Banking | Apr 15, 2025 |
Reverse Duplicate Credit Meaning | Apr 15, 2025 |
Macro Manager Definition How Management Works And Benefits | Apr 15, 2025 |