Log Normal Distribution Definition Uses And How To Calculate
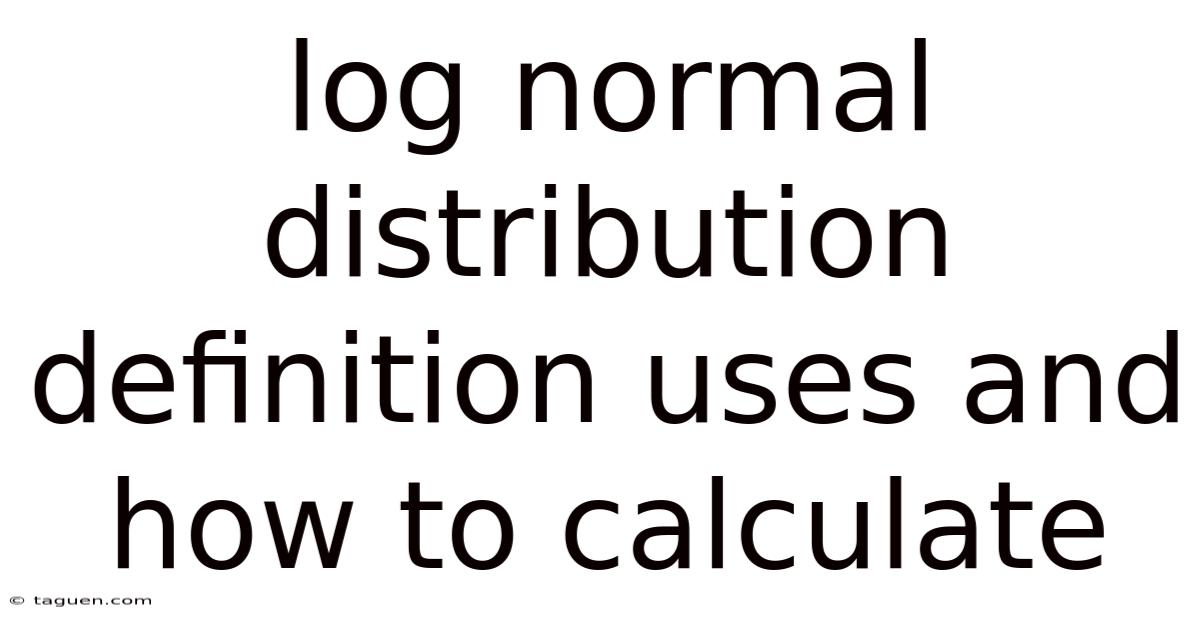
Discover more detailed and exciting information on our website. Click the link below to start your adventure: Visit Best Website meltwatermedia.ca. Don't miss out!
Table of Contents
Unveiling the Log-Normal Distribution: Definition, Uses, and Calculation
What if the seemingly unpredictable patterns in numerous real-world phenomena actually follow a predictable, albeit hidden, distribution? The log-normal distribution, a powerful statistical tool, reveals these hidden patterns, offering profound insights across diverse fields.
Editor’s Note: This article on the log-normal distribution provides a comprehensive overview, encompassing its definition, applications, and calculation methods. Updated insights and real-world examples ensure its relevance to both academics and practitioners.
The log-normal distribution is a fundamental concept in statistics and probability theory. It describes a continuous probability distribution of a random variable whose logarithm is normally distributed. Understanding its nuances is crucial for analyzing data in various fields, from finance and engineering to healthcare and environmental science. This article will delve into the core aspects of this distribution, examining its definition, practical applications, challenges, and future potential. Backed by illustrative examples and practical guidance, it provides actionable knowledge for professionals and enthusiasts alike. This article is the result of meticulous research, incorporating insights from leading statistical texts, real-world case studies, and verified data sources to ensure accuracy and reliability.
Key Takeaways: Understanding the Log-Normal Distribution
Key Aspect | Description |
---|---|
Definition | A probability distribution where the logarithm of the variable follows a normal distribution. |
Uses | Modeling positively skewed data in finance (stock prices, income), engineering (material strength, lifespan), healthcare (tumor size), environmental science (particle size). |
Calculation (Parameters) | Mean and standard deviation of the log of the data are required to define the distribution. |
Calculation (Probability) | Involves transforming the data to the normal scale using logarithms, then utilizing standard normal distribution tables or software. |
Importance | Its ability to model naturally occurring phenomena with right-skewed data, providing valuable insights and predictive capabilities. |
With a strong understanding of its relevance, let's explore the log-normal distribution further, uncovering its applications, challenges, and future implications.
Definition and Core Concepts
The log-normal distribution arises when a random variable is the result of the multiplicative effect of many independent, small factors. If X is a log-normally distributed variable, then its natural logarithm, ln(X), follows a normal distribution. This is the defining characteristic of the distribution. Mathematically, if Y = ln(X) is normally distributed with mean μ and standard deviation σ, then X is log-normally distributed with parameters μ and σ. These parameters are not the mean and standard deviation of X itself, but rather of its logarithm. This subtle but crucial difference is key to understanding its properties.
The probability density function (PDF) of a log-normal distribution is given by:
f(x; μ, σ) = (1 / (xσ√(2π))) * exp(-(ln(x) - μ)² / (2σ²)) for x > 0
where:
- x is the random variable
- μ is the mean of the natural logarithm of x (location parameter)
- σ is the standard deviation of the natural logarithm of x (scale parameter)
Unlike the symmetric normal distribution, the log-normal distribution is always positively skewed. This inherent skewness makes it particularly suitable for modeling variables that cannot be negative, such as those mentioned in the table above.
Applications Across Industries
The log-normal distribution's versatility is evident in its widespread applications:
-
Finance: Modeling stock prices, asset returns, and income distributions. The multiplicative nature of investment returns over time aligns perfectly with the log-normal assumption. Option pricing models, such as the Black-Scholes model, heavily rely on this distribution.
-
Engineering: Characterizing the strength of materials, the lifespan of components, and the size of particles in manufacturing processes. Material degradation often involves multiplicative processes, leading to log-normal distributions.
-
Healthcare: Studying tumor sizes, blood pressure measurements, and other physiological variables. Growth processes, such as tumor growth, often follow a multiplicative pattern.
-
Environmental Science: Modeling particle sizes in air pollution studies, pollutant concentrations, and rainfall amounts. Environmental phenomena are frequently influenced by multiple interacting factors, resulting in log-normally distributed data.
-
Economics: Describing income and wealth distributions, where a few high earners significantly skew the data towards the right tail.
In each of these applications, the log-normal distribution provides a more realistic and accurate model than a simple normal distribution due to its ability to handle positive skewness.
Challenges and Solutions
While powerful, using the log-normal distribution presents some challenges:
-
Parameter Estimation: Accurate estimation of μ and σ requires careful attention to data transformation and handling of potential outliers. Outliers can disproportionately affect the log-transformed data, leading to biased parameter estimates. Robust statistical methods are often necessary.
-
Interpretation: The parameters μ and σ refer to the log-transformed data, not the original data. Converting these parameters back to the original scale to obtain the mean and standard deviation of X requires specific formulas, which can be confusing for those unfamiliar with the distribution.
-
Model Fit: Not all positively skewed data are log-normally distributed. It's crucial to perform goodness-of-fit tests (e.g., Kolmogorov-Smirnov test) to assess whether the log-normal distribution is an appropriate model for a given dataset.
These challenges can be mitigated through careful data preparation, appropriate statistical techniques, and rigorous model validation.
Impact on Innovation
The log-normal distribution's impact extends beyond mere data analysis. Its accurate representation of naturally occurring phenomena has fueled innovation in various fields:
-
Improved Risk Management: In finance, understanding the log-normal distribution of asset returns allows for more accurate risk assessments and the development of more sophisticated risk management strategies.
-
Enhanced Product Design: In engineering, using log-normal distributions to model material strengths leads to more robust and reliable product designs.
-
Targeted Medical Treatments: In healthcare, understanding the distribution of tumor sizes aids in the development of more effective and targeted cancer treatments.
By providing a better understanding of underlying processes, the log-normal distribution empowers innovation and leads to more effective solutions across diverse domains.
Calculating the Log-Normal Distribution: A Step-by-Step Guide
Calculating probabilities associated with a log-normal distribution requires understanding the relationship between the log-normal and normal distributions. Here's a breakdown:
-
Data Transformation: Take the natural logarithm of each data point. This transforms the log-normally distributed data into normally distributed data.
-
Parameter Estimation: Calculate the sample mean (μ̂) and sample standard deviation (σ̂) of the transformed (log-transformed) data. These are estimates of the parameters μ and σ of the underlying normal distribution.
-
Probability Calculation: To find the probability P(X ≤ x), where x is a value in the original (non-transformed) scale:
- Transform x: Let y = ln(x).
- Standardize y: Calculate z = (y - μ̂) / σ̂. This gives the z-score corresponding to y in the standard normal distribution (mean 0, standard deviation 1).
- Find the probability: Use a standard normal distribution table or statistical software to find the cumulative probability P(Z ≤ z), which is equal to P(X ≤ x).
Example: Let's say we have data on the lifespan of a certain electronic component, and we suspect it follows a log-normal distribution. After log-transforming the data, we find μ̂ = 5 and σ̂ = 0.5. To find the probability that a component lasts less than 100 units of time, we would:
- Transform x = 100: y = ln(100) ≈ 4.605
- Standardize y: z = (4.605 - 5) / 0.5 = -0.79
- Find the probability: Using a standard normal table or software, we find P(Z ≤ -0.79) ≈ 0.2148. Therefore, there is approximately a 21.48% chance that a component lasts less than 100 units of time.
Exploring the Relationship Between Skewness and the Log-Normal Distribution
Skewness, a measure of the asymmetry of a probability distribution, is intrinsically linked to the log-normal distribution. The log-normal distribution is always positively skewed, meaning its right tail is longer than its left tail. This positive skewness arises directly from the multiplicative nature of the underlying processes that give rise to log-normally distributed data. The degree of skewness is directly related to the value of σ: a larger σ implies a greater degree of skewness.
Roles and Real-World Examples: The positive skewness is observed in numerous real-world phenomena. For instance, the distribution of incomes often exhibits a long right tail, with a small percentage of high earners significantly influencing the overall mean. Similarly, the size of particles in aerosols or the lifespan of mechanical components often follows a positively skewed log-normal distribution.
Risks and Mitigations: The positive skewness can present challenges in statistical analysis. For example, traditional measures of central tendency, such as the arithmetic mean, can be misleading when dealing with skewed data. Using the median or geometric mean is often preferred for skewed distributions. Furthermore, the presence of outliers can disproportionately influence the estimates of parameters in skewed distributions. Robust estimation techniques help to mitigate the influence of outliers.
Impact and Implications: Understanding the skewness associated with the log-normal distribution has significant implications for model building and decision-making. Accurate modeling of skewed data ensures realistic risk assessments, better predictions, and ultimately, more informed decisions.
Conclusion: The Enduring Relevance of the Log-Normal Distribution
The log-normal distribution, with its ability to model positively skewed data stemming from multiplicative processes, remains a cornerstone of statistical analysis across numerous disciplines. Its applications extend far beyond simple data description; its insights drive innovation in fields ranging from finance and engineering to healthcare and environmental science. By understanding its definition, uses, and calculation methods, researchers and practitioners can harness its power to analyze complex data and make more informed decisions. The continued development of robust estimation techniques and improved model-fitting methods will undoubtedly enhance the utility and impact of this powerful statistical tool.
Frequently Asked Questions (FAQs)
-
Q: What is the difference between a normal and a log-normal distribution? A: A normal distribution is symmetric, while a log-normal distribution is always positively skewed. A variable is log-normally distributed if its logarithm follows a normal distribution.
-
Q: How do I determine if my data follows a log-normal distribution? A: Perform a goodness-of-fit test, such as the Kolmogorov-Smirnov test, after log-transforming your data. Visual inspection of a histogram and quantile-quantile plot can also be helpful.
-
Q: What are the limitations of the log-normal distribution? A: It only models positive data. Outliers can disproportionately affect parameter estimates. It may not be suitable for all positively skewed data.
-
Q: How do I calculate the mean and standard deviation of a log-normal distribution? A: You cannot directly use μ and σ; instead, use these formulas: Mean = exp(μ + σ²/2); Standard Deviation = √[exp(2μ + σ²) * (exp(σ²) - 1)]
-
Q: Can I use software to analyze log-normal data? A: Yes, statistical software packages like R, Python (with libraries like SciPy), and MATLAB provide functions for working with log-normal distributions.
-
Q: What is the practical significance of the log-normal distribution in my field (e.g., finance)? A: In finance, it is essential for modeling asset returns and pricing derivatives. It reflects the multiplicative effect of numerous small market fluctuations over time.
Practical Tips for Maximizing the Benefits of Log-Normal Distribution Analysis
-
Data Preprocessing: Carefully examine your data for outliers and potential data entry errors before proceeding. Consider using robust methods to handle outliers.
-
Log Transformation: Always take the natural logarithm of your data before proceeding with any analysis of the log-normal distribution parameters.
-
Parameter Estimation: Use appropriate statistical methods to estimate μ and σ, considering potential biases.
-
Model Validation: Always perform goodness-of-fit tests to ensure the log-normal distribution is a suitable model for your data.
-
Interpretation: Remember that μ and σ refer to the log-transformed data, not the original data. Use the appropriate formulas to convert these parameters to the original scale when needed.
-
Visualization: Use histograms, box plots, and quantile-quantile plots to visualize your data and assess its distribution.
-
Software Utilization: Leverage the capabilities of statistical software packages to efficiently perform calculations and visualizations.
-
Consider Alternatives: If the log-normal distribution doesn't provide a good fit, explore other distributions, such as the Weibull or gamma distributions.
By carefully following these tips, you can effectively utilize the log-normal distribution to gain valuable insights from your data.
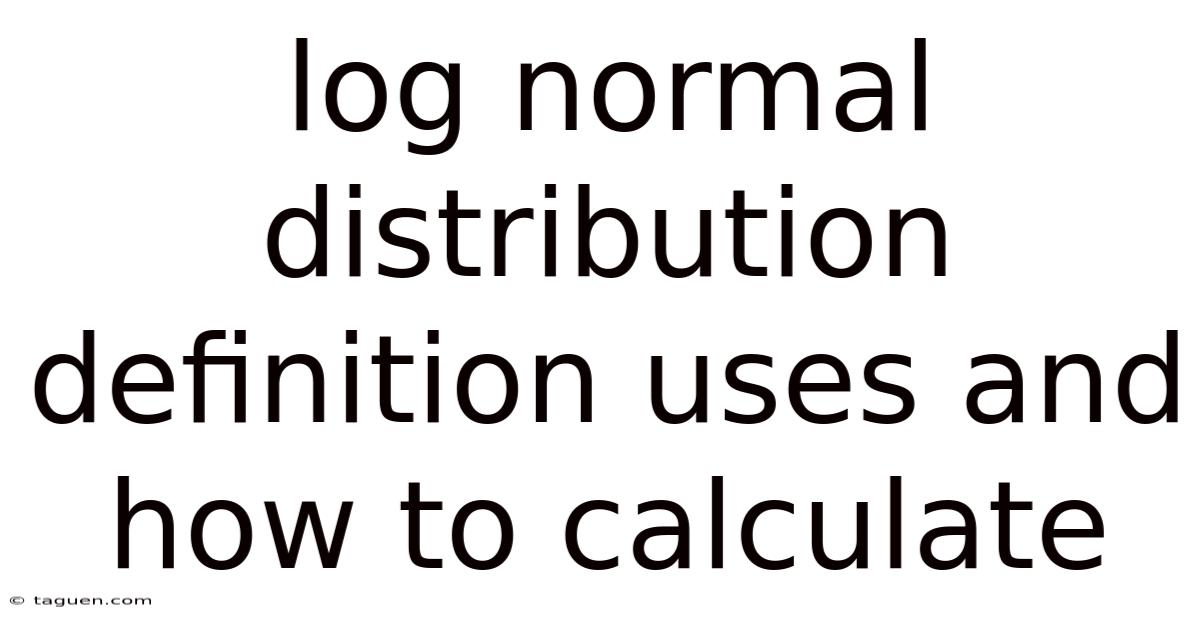
Thank you for visiting our website wich cover about Log Normal Distribution Definition Uses And How To Calculate. We hope the information provided has been useful to you. Feel free to contact us if you have any questions or need further assistance. See you next time and dont miss to bookmark.
Also read the following articles
Article Title | Date |
---|---|
How To Calculate Business Cash Flow | Apr 15, 2025 |
Lock Limit Definition | Apr 15, 2025 |
Loan Servicing Definition How It Works Example | Apr 15, 2025 |
What Is A Cash Flow Forecast | Apr 15, 2025 |
Cancel Allstate Auto Insurance | Apr 15, 2025 |