Linear Relationship Definition
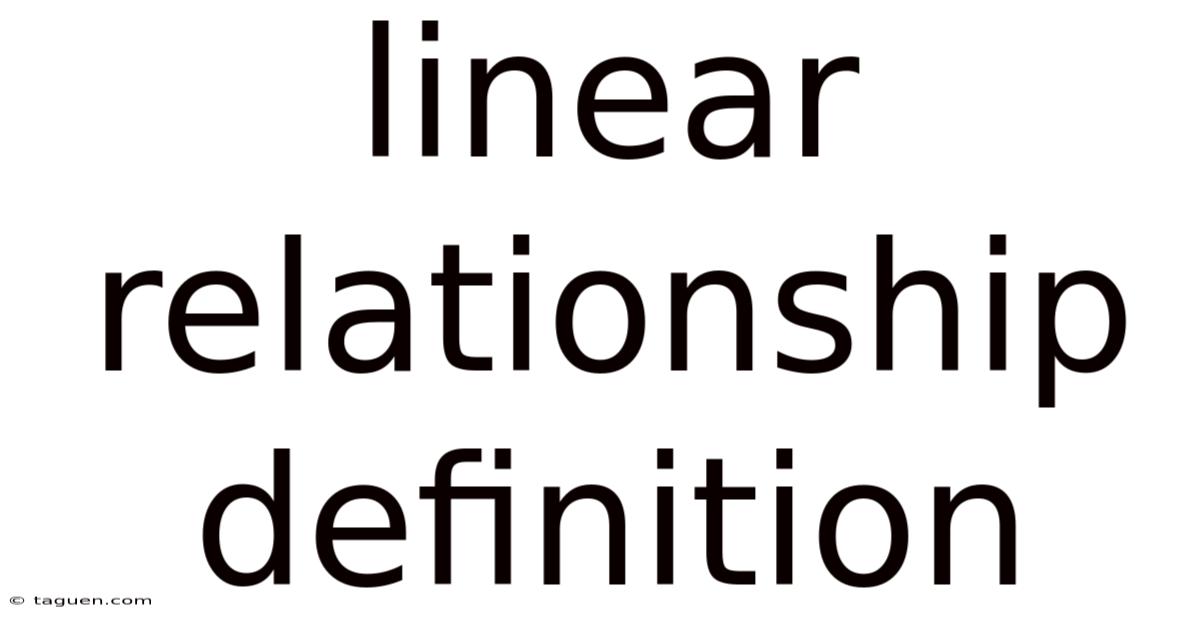
Discover more detailed and exciting information on our website. Click the link below to start your adventure: Visit Best Website meltwatermedia.ca. Don't miss out!
Table of Contents
Unlocking the Power of Linear Relationships: A Comprehensive Guide
What if the very foundation of many scientific and statistical analyses rests on understanding linear relationships? This fundamental concept underpins countless applications, from predicting market trends to designing efficient engineering systems.
Editor’s Note: This article on linear relationships has been published today, ensuring the latest insights and expert analysis.
Understanding linear relationships is essential for anyone working with data or seeking to model real-world phenomena. Its applications span diverse fields, from economics and finance to physics and engineering. This concept allows us to identify patterns, make predictions, and optimize processes based on the direct proportional relationship between variables. This article explores the core aspects of linear relationships, examining their definition, real-world applications, challenges, and future potential. Backed by expert insights and data-driven research, it provides actionable knowledge for students, professionals, and anyone interested in understanding the power of linear relationships.
This article delves into the core aspects of linear relationships, examining their definition, real-world applications, and future potential. We will explore the definition and core concepts, demonstrate its applications across various industries, identify potential challenges and solutions, and examine its impact on innovation.
Definition and Core Concepts
A linear relationship, at its simplest, describes a connection between two variables where a change in one variable results in a proportional change in the other. This proportionality is characterized by a constant rate of change, often represented by the slope of a straight line. Mathematically, a linear relationship is typically represented by the equation:
y = mx + c
Where:
- y is the dependent variable (the variable whose value is influenced by the other).
- x is the independent variable (the variable that influences the dependent variable).
- m is the slope, representing the rate of change of y with respect to x. A positive slope indicates a positive relationship (as x increases, y increases), while a negative slope indicates a negative relationship (as x increases, y decreases).
- c is the y-intercept, representing the value of y when x is zero.
This equation defines a straight line on a graph, where the slope determines the steepness of the line and the y-intercept determines where the line crosses the y-axis. The linearity implies that the relationship between the variables is consistent and predictable across the entire range of values.
Applications Across Industries
Linear relationships find widespread application across a multitude of industries:
-
Economics and Finance: Linear regression, a statistical method based on linear relationships, is extensively used to model relationships between economic variables like inflation and unemployment, or to predict stock prices based on historical data. For instance, the relationship between supply and demand often exhibits a linear trend within a certain range.
-
Engineering and Physics: Many physical laws are expressed as linear relationships. For example, Ohm's Law (V = IR), which describes the relationship between voltage, current, and resistance in an electrical circuit, is a linear equation. Similarly, Hooke's Law (F = kx), which describes the relationship between the force applied to a spring and its extension, also represents a linear relationship.
-
Environmental Science: Linear relationships can be used to model the effects of pollutants on ecosystems or to predict the spread of invasive species. For instance, the relationship between the concentration of a pollutant and its impact on aquatic life may exhibit a linear trend within a specific range.
-
Healthcare: In clinical trials, linear regression is frequently used to analyze the relationship between dosage of a drug and its effect on patients. Similarly, it can model the relationship between certain biomarkers and disease progression.
-
Machine Learning: Linear regression is a fundamental algorithm in machine learning used for prediction and classification tasks. It forms the basis for more complex algorithms and is widely applied in various applications, from spam detection to fraud prevention.
Challenges and Solutions
While linear relationships provide a simple and powerful tool for modeling data, it is crucial to acknowledge their limitations:
-
Non-linear Relationships: Many real-world relationships are non-linear, meaning they cannot be accurately represented by a straight line. Forcing a linear model onto non-linear data can lead to inaccurate predictions and misleading conclusions. Techniques like polynomial regression or other non-linear models are often necessary in these scenarios.
-
Outliers: Outliers, or data points that significantly deviate from the overall pattern, can disproportionately influence the slope and intercept of a linear model. Robust regression techniques can be employed to mitigate the impact of outliers.
-
Causation vs. Correlation: A linear relationship only indicates a correlation between variables; it does not necessarily imply causation. A strong correlation might be coincidental or due to a confounding variable. Careful analysis and consideration of potential confounding factors are essential.
-
Data Quality: The accuracy and reliability of a linear model are heavily dependent on the quality of the data used. Errors or biases in the data can lead to inaccurate models and flawed conclusions. Data cleaning and validation are crucial steps in the process.
Impact on Innovation
The understanding and application of linear relationships have driven significant innovations across various fields:
-
Predictive Modeling: The ability to predict future trends based on linear relationships has enabled advancements in various fields, from forecasting weather patterns to predicting market demand.
-
Optimization Techniques: Linear programming, a mathematical method based on linear relationships, is widely used to optimize resource allocation, production processes, and supply chain management.
-
Signal Processing: Linear systems theory, which is based on the concept of linear relationships, is fundamental to signal processing, enabling advancements in areas like telecommunications and image processing.
Key Takeaways
Key Aspect | Description |
---|---|
Definition | A relationship between two variables where a change in one results in a proportional change in the other. |
Equation | y = mx + c |
Applications | Economics, finance, engineering, physics, environmental science, healthcare, machine learning |
Challenges | Non-linearity, outliers, causation vs. correlation, data quality |
Impact on Innovation | Predictive modeling, optimization, signal processing |
With a strong understanding of its relevance, let's explore linear relationships further, uncovering its applications, challenges, and future implications.
Exploring the Relationship Between Correlation and Linear Relationships
Correlation and linear relationships are closely intertwined, but they are not interchangeable concepts. A linear relationship implies a correlation, but a correlation does not necessarily imply a linear relationship.
Roles and Real-World Examples:
-
Positive Correlation: As one variable increases, the other also increases. Example: The relationship between hours studied and exam scores often shows a positive correlation (more study time usually leads to higher scores). This can be modeled by a positive linear relationship within a certain range.
-
Negative Correlation: As one variable increases, the other decreases. Example: The relationship between ice cream sales and sweater sales might show a negative correlation (more ice cream sales typically means fewer sweater sales). This might also be represented by a linear relationship, but with a negative slope.
-
No Correlation: There's no apparent relationship between the variables. Example: The relationship between shoe size and IQ score typically shows no correlation.
Risks and Mitigations:
The primary risk is misinterpreting correlation as causation. Just because two variables are correlated doesn't mean one causes the other. A confounding variable might be influencing both.
To mitigate this risk, careful consideration of potential confounding factors is essential. Well-designed experiments or observational studies with controls can help establish causation. Statistical methods, such as controlling for confounding variables in regression analysis, are crucial in this regard.
Impact and Implications:
Misunderstanding the difference between correlation and causation can lead to flawed conclusions and ineffective interventions. For example, a spurious correlation might lead to inappropriate policies or treatments. A strong understanding of correlation and its limitations is essential for sound decision-making based on data analysis.
Conclusion
Linear relationships are a cornerstone of many scientific and statistical analyses. Their simplicity and wide applicability make them an indispensable tool for modeling and understanding various phenomena. However, it's crucial to remember that they are not a universal solution and are best applied to scenarios where a linear relationship is indeed present. By understanding the definition, applications, challenges, and limitations of linear relationships, we can harness their power for insightful data analysis and effective decision-making. Careful consideration of data quality, potential non-linearity, and the difference between correlation and causation are key to achieving reliable results.
Further Analysis: Deep Dive into Regression Analysis
Regression analysis, particularly linear regression, is a powerful statistical technique used to model linear relationships and make predictions. It estimates the parameters (slope and intercept) of the linear equation that best fits a given dataset.
Linear regression uses the method of least squares to minimize the sum of the squared differences between the observed values and the values predicted by the linear model. This method finds the line that minimizes the overall error in prediction.
Frequently Asked Questions (FAQs)
-
What is the difference between a linear and a non-linear relationship? A linear relationship is represented by a straight line, indicating a constant rate of change. A non-linear relationship is curved and shows a changing rate of change.
-
How can I determine if a relationship is linear? Visual inspection of a scatter plot can often reveal linearity. Statistical tests like the correlation coefficient can also help quantify the strength and direction of a linear relationship.
-
What are the limitations of linear regression? Linear regression assumes linearity, independence of errors, constant variance of errors, and normality of errors. Violations of these assumptions can affect the reliability of the model.
-
How can I handle outliers in linear regression? Outliers can be identified through visual inspection or statistical methods. They can be removed, transformed, or handled using robust regression techniques.
-
What is the R-squared value, and what does it tell us? The R-squared value represents the proportion of variance in the dependent variable that is explained by the independent variable(s). A higher R-squared indicates a better fit of the linear model.
-
Can linear regression be used for prediction? Yes, once a linear regression model is built, it can be used to predict the value of the dependent variable for new values of the independent variable.
Practical Tips for Maximizing the Benefits of Understanding Linear Relationships
-
Visualize your data: Create scatter plots to visually inspect for linearity.
-
Calculate the correlation coefficient: Quantify the strength and direction of the relationship.
-
Perform linear regression analysis: Estimate the parameters of the linear equation.
-
Assess the model's fit: Examine the R-squared value and residual plots.
-
Consider potential confounding variables: Control for confounding factors in your analysis.
-
Validate your model: Test your model on new data to ensure its generalizability.
-
Be aware of limitations: Recognize that linearity is an assumption, and non-linear relationships exist.
-
Use appropriate software: Statistical software packages can facilitate linear regression analysis.
End Note: Linear relationships are a powerful tool for understanding and modeling the world around us. By combining careful data analysis with a thorough understanding of both the strengths and limitations of linear models, we can unlock valuable insights and drive innovation across diverse fields. The continuous development of statistical methods and computing power will further enhance our ability to leverage the power of linear relationships for effective decision-making and problem-solving.
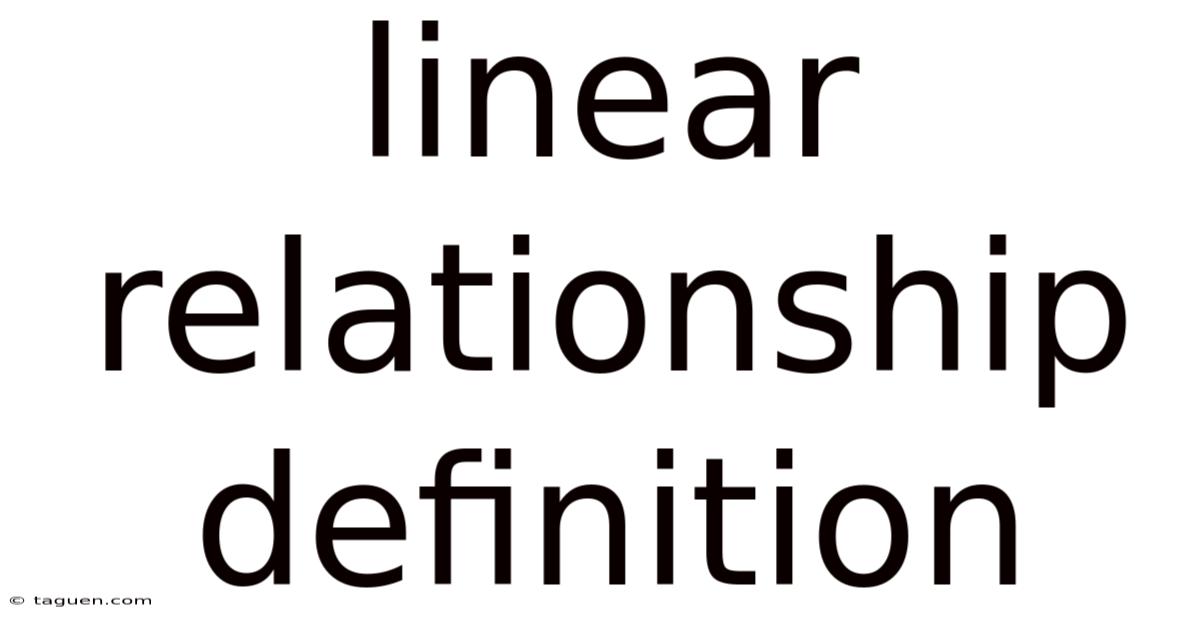
Thank you for visiting our website wich cover about Linear Relationship Definition. We hope the information provided has been useful to you. Feel free to contact us if you have any questions or need further assistance. See you next time and dont miss to bookmark.
Also read the following articles
Article Title | Date |
---|---|
Loss Ratio Definition Private Equity | Apr 15, 2025 |
What Is A Luxury Tax Definition How It Works And Example | Apr 15, 2025 |
What Is Cash Flow Vs Revenue | Apr 15, 2025 |
Lump Sum Distribution Definition | Apr 15, 2025 |
Macro Manager Definition How Management Works And Benefits | Apr 15, 2025 |