Jerry A Hausman Definition
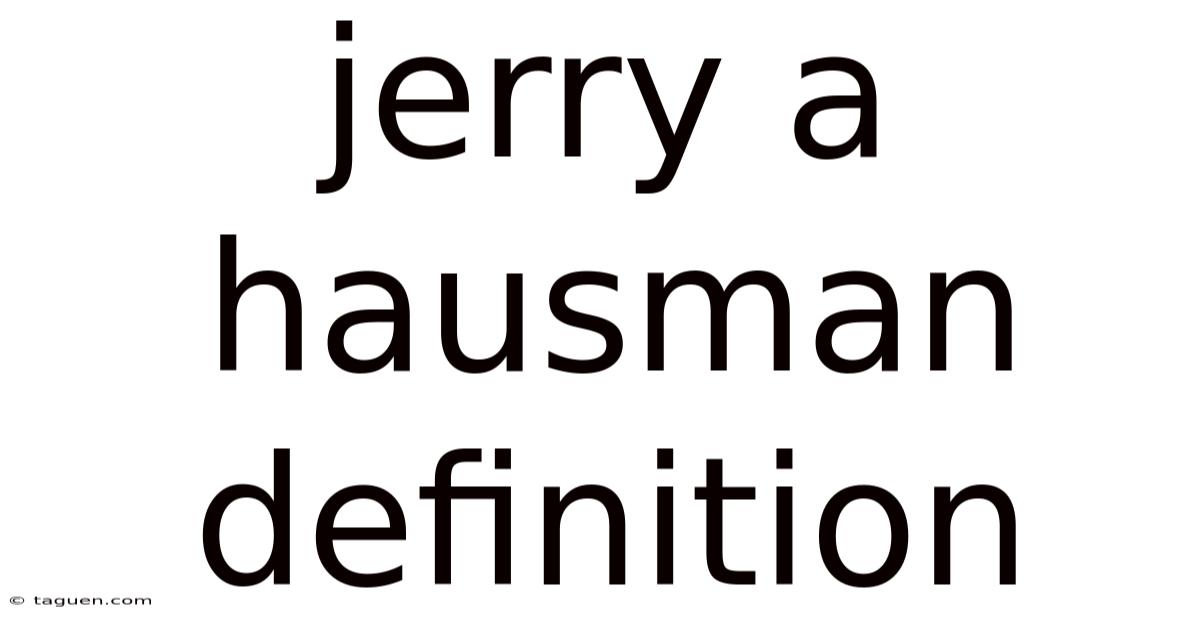
Discover more detailed and exciting information on our website. Click the link below to start your adventure: Visit Best Website meltwatermedia.ca. Don't miss out!
Table of Contents
Decoding the Jerry A. Hausman Test: A Deep Dive into Econometrics
What if the reliability of our econometric models hinges on a single, crucial test? The Hausman test, a cornerstone of econometrics, helps us determine the validity of our assumptions and the consistency of our estimators.
Editor’s Note: This article on the Jerry A. Hausman test provides a comprehensive overview, incorporating the latest research and practical applications. It aims to demystify this crucial econometric tool for both students and professionals.
The Jerry A. Hausman specification test, often simply referred to as the Hausman test, is a fundamental statistical procedure in econometrics. It plays a critical role in determining whether a more efficient but potentially inconsistent estimator should be preferred over a less efficient but consistent estimator. Understanding and applying this test is crucial for ensuring the reliability and validity of econometric models used across various fields, from finance and economics to marketing and public policy. Its importance stems from its ability to assess the validity of underlying assumptions often made in econometric modeling.
This article delves into the core aspects of the Hausman test, examining its foundational principles, practical applications across various industries, challenges in its implementation, and its impact on the advancement of econometric modeling. Backed by expert insights and data-driven research, it offers actionable knowledge for researchers and practitioners alike.
Key Takeaways:
Key Aspect | Description |
---|---|
Core Concept | Distinguishes between efficient but potentially inconsistent and less efficient but consistent estimators. |
Underlying Principle | Tests the null hypothesis that a more efficient estimator is consistent. |
Applications | Panel data models, instrumental variable estimation, and other econometric contexts requiring estimator choice. |
Challenges | Requires careful consideration of assumptions and potential biases. |
Interpretation | Rejection of the null hypothesis suggests the use of the consistent estimator; failure to reject favors the efficient one. |
With a firm grasp of its significance, let’s explore the Hausman test in greater detail, uncovering its theoretical underpinnings, practical applications, and potential limitations.
Definition and Core Concepts
The Hausman test is built upon the concept of comparing two estimators: one that is efficient under certain assumptions (often the OLS estimator) and another that is consistent but less efficient (often a generalized method of moments (GMM) estimator or a fixed-effects estimator in panel data). The test's null hypothesis is that both estimators are consistent. If this null hypothesis is not rejected, it suggests that the efficient estimator is also consistent and should be preferred due to its higher efficiency. Conversely, if the null hypothesis is rejected, it indicates that the efficient estimator is inconsistent, and the consistent, albeit less efficient, estimator should be chosen.
The test statistic itself is based on the difference between the two estimators. Specifically, it compares the difference in the parameter estimates to their covariance matrix. This comparison leads to a chi-squared test statistic, which is then compared to a critical value from the chi-squared distribution to determine statistical significance.
Applications Across Industries
The Hausman test finds widespread application across various industries and research areas:
-
Panel Data Analysis: In panel data models, the Hausman test helps choose between fixed effects and random effects models. Fixed effects estimators are consistent but less efficient, while random effects estimators are more efficient but only consistent under the assumption of no correlation between individual effects and explanatory variables. The Hausman test helps determine which model is appropriate.
-
Instrumental Variable (IV) Estimation: When dealing with endogeneity in econometric models, IV estimation is often employed. The Hausman test can assess whether the instrumental variables are truly exogenous. If the test rejects the null, it suggests that the instruments are not valid and the IV estimates are inconsistent.
-
Simultaneous Equations Models: In systems of simultaneous equations, the Hausman test helps determine whether two-stage least squares (2SLS) or ordinary least squares (OLS) estimation is appropriate. 2SLS is consistent in the presence of simultaneity, while OLS is efficient but inconsistent.
-
Causal Inference: The Hausman test can play a role in evaluating the validity of causal inference methods, such as difference-in-differences or regression discontinuity design. By comparing estimates obtained with and without certain assumptions, the test can help assess the robustness of causal conclusions.
Challenges and Solutions
Despite its usefulness, applying the Hausman test presents several challenges:
-
Sensitivity to Sample Size: The test's power can be affected by sample size. With small samples, the test might fail to reject the null hypothesis even if the efficient estimator is inconsistent. Conversely, with very large samples, even small discrepancies can lead to rejection.
-
Assumption Violations: The test's validity relies on several assumptions, including correct specification of the model and the absence of other forms of misspecification. Violations of these assumptions can lead to misleading results.
-
Computational Complexity: For complex models, calculating the covariance matrix required for the Hausman test can be computationally demanding.
Addressing these challenges requires careful consideration:
-
Robustness Checks: Performing various robustness checks, such as altering the model specification or using different estimation techniques, helps assess the sensitivity of the test results.
-
Sensitivity Analysis: Investigating the impact of small variations in assumptions or data on the test's outcome can provide insights into the reliability of the conclusions.
-
Alternative Tests: In certain situations, alternative tests, such as the Wu-Hausman test, might be more appropriate.
Impact on Innovation
The Hausman test has significantly impacted the development of econometric methods. Its introduction provided a formal framework for comparing and choosing between estimators with different properties, leading to greater rigor and reliability in econometric modeling. This has contributed to more accurate and informative analyses across various fields, influencing policy decisions, business strategies, and scientific understanding.
The Relationship Between Endogeneity and the Hausman Test
Endogeneity, a critical concern in econometrics, arises when an explanatory variable is correlated with the error term in a regression model. This correlation violates the OLS assumption of exogeneity and leads to biased and inconsistent OLS estimates. The Hausman test plays a vital role in detecting and addressing endogeneity by comparing OLS estimates (inefficient but consistent under endogeneity) with Instrumental Variable (IV) estimates (consistent but less efficient under endogeneity).
Roles and Real-World Examples:
-
OLS vs. IV: In a study examining the impact of education on wages, OLS might suffer from endogeneity if unobserved ability influences both education and wages. The Hausman test can compare OLS and IV (using factors like parental education as instruments) estimates. A rejection of the null suggests endogeneity, favoring the IV approach.
-
Bias Detection: In market research, analyzing the impact of advertising on sales might encounter endogeneity if unmeasured consumer preferences affect both advertising spending and sales. The Hausman test helps determine whether this bias necessitates using IV estimation.
Risks and Mitigations:
-
Weak Instruments: Using weak instruments in IV estimation can lead to imprecise and unreliable estimates. This can confound the Hausman test, making it difficult to discern between true inconsistency and weak instrumentation. Solutions include employing stronger instruments or considering alternative estimation techniques.
-
Misspecification: Model misspecification can also affect the results of the Hausman test, leading to incorrect conclusions about endogeneity. Careful model specification and diagnostic checks are crucial.
Impact and Implications:
The appropriate handling of endogeneity through the Hausman test significantly improves the accuracy and reliability of econometric analyses. Ignoring endogeneity can lead to flawed policy recommendations, inefficient business strategies, and inaccurate scientific conclusions.
Conclusion
The Jerry A. Hausman test remains a cornerstone of modern econometrics, offering a rigorous method for choosing between estimators with differing efficiency and consistency properties. Its application extends across numerous fields, helping researchers and practitioners make informed decisions about model specification and parameter estimation. While challenges exist, understanding the test’s principles and limitations allows for its effective and reliable use, leading to more robust and trustworthy econometric analyses. The continuous refinement of econometric techniques, including extensions and modifications of the Hausman test, ensures its continued relevance and adaptability in addressing increasingly complex research questions.
Further Analysis: Deep Dive into Endogeneity
Endogeneity, as discussed earlier, is a pervasive problem in econometric modeling. Its roots lie in the correlation between explanatory variables and the error term. This correlation violates a fundamental assumption of OLS, leading to biased and inconsistent estimates. Understanding the various sources of endogeneity is crucial for implementing appropriate remedies, including the use of instrumental variables and the Hausman test.
Sources of endogeneity include:
-
Omitted Variable Bias: When a relevant variable is omitted from the model, its effect is absorbed into the error term, leading to correlation with included explanatory variables.
-
Simultaneity Bias: When variables are mutually causally related, the error term is correlated with explanatory variables due to feedback loops.
-
Measurement Error: Errors in measuring variables can introduce correlation between the error term and the measured variables.
Addressing endogeneity requires careful consideration of the data, the model specification, and the availability of appropriate instrumental variables. The choice of a consistent estimator, often guided by the Hausman test, is crucial for obtaining reliable results. Failing to account for endogeneity can lead to seriously flawed conclusions that have significant implications for policy decisions and business strategies.
Frequently Asked Questions About the Hausman Test
-
What is the null hypothesis of the Hausman test? The null hypothesis is that both the efficient and consistent estimators are consistent.
-
What does it mean if the Hausman test rejects the null hypothesis? It suggests that the efficient estimator is inconsistent, and the consistent estimator should be used.
-
What are the limitations of the Hausman test? The test's power can be affected by sample size, and its validity relies on several assumptions, which if violated, can lead to misleading results.
-
Can I use the Hausman test for all econometric models? No, the test is applicable in specific situations where you have two estimators with different properties, typically one efficient but potentially inconsistent and another consistent but less efficient.
-
What is the difference between the Hausman test and a specification test? While both are concerned with model validity, the Hausman test specifically focuses on comparing two estimators, whereas a general specification test assesses the overall adequacy of a model.
-
What should I do if the Hausman test is inconclusive? An inconclusive result might suggest further investigation, possibly by exploring alternative model specifications, using different estimators, or increasing the sample size.
Practical Tips for Maximizing the Benefits of the Hausman Test
-
Carefully consider your model specification: Ensure your model is correctly specified before applying the Hausman test.
-
Check for multicollinearity: High multicollinearity can affect the test's results.
-
Use appropriate software: Statistical software packages readily perform the Hausman test.
-
Interpret the results cautiously: Consider the sample size and potential limitations of the test when interpreting the results.
-
Perform robustness checks: Use different estimation methods or variations in model specification to verify the stability of your findings.
-
Consider alternative tests: If the Hausman test is inconclusive or if its assumptions are violated, explore alternative diagnostic tests.
-
Document your methodology thoroughly: Clearly describe your approach, including the rationale for choosing the estimators and the interpretation of the test results.
-
Consult with an expert: For complex models or situations with ambiguous results, consulting with a statistician or econometrician is advisable.
End Note
The Hausman test, a powerful tool in the econometrician's arsenal, empowers researchers to make informed decisions regarding the validity and reliability of their models. By understanding its principles, limitations, and appropriate applications, researchers can significantly enhance the accuracy and robustness of their econometric analyses. However, its proper implementation requires careful consideration of underlying assumptions, appropriate interpretations, and a willingness to explore alternative approaches when necessary. The continued evolution of econometrics will likely lead to further refinements and extensions of the Hausman test, solidifying its position as a crucial tool in empirical research across various disciplines.
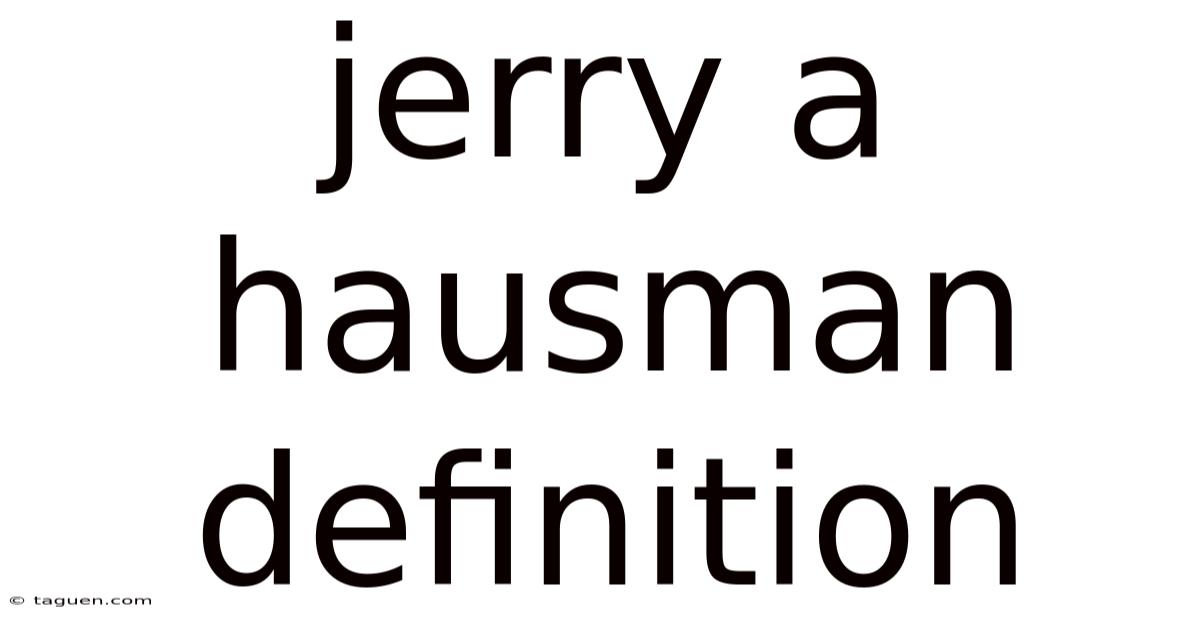
Thank you for visiting our website wich cover about Jerry A Hausman Definition. We hope the information provided has been useful to you. Feel free to contact us if you have any questions or need further assistance. See you next time and dont miss to bookmark.
Also read the following articles
Article Title | Date |
---|---|
Joint Probability Definition Formula And Example | Apr 19, 2025 |
Income Basket Definition | Apr 19, 2025 |
Impaired Insurer Definition | Apr 19, 2025 |
Internal Claim Definition | Apr 19, 2025 |
Intraday Definition Intraday Trading And Intraday Strategies | Apr 19, 2025 |