Hypothesis To Be Tested Definition And 4 Steps For Testing With Example
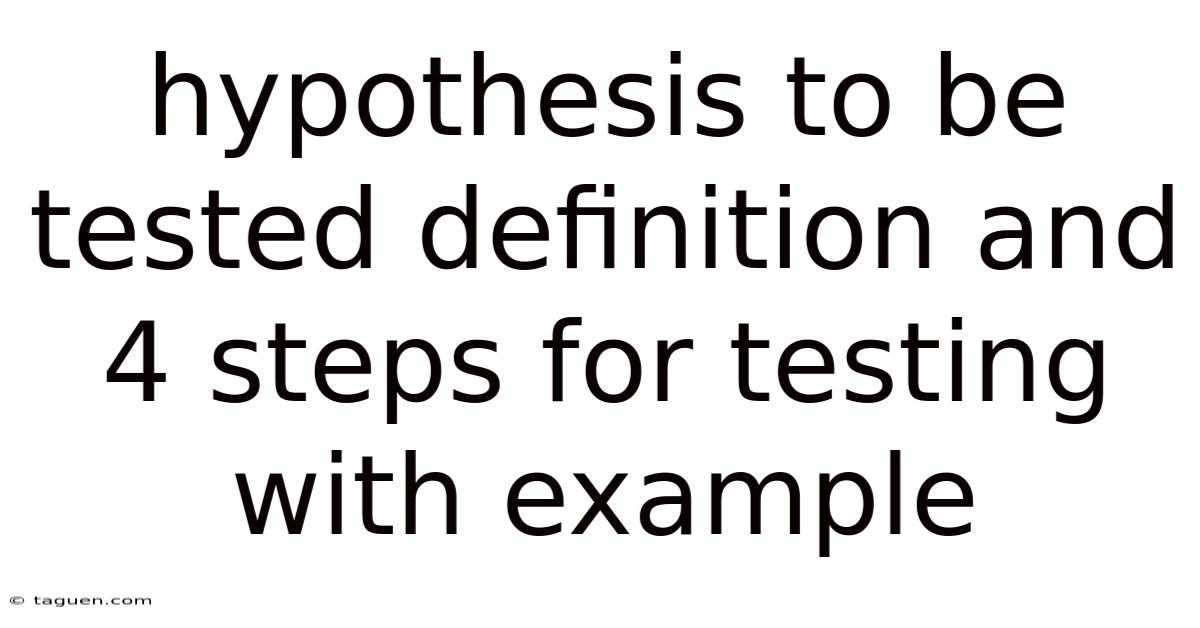
Discover more detailed and exciting information on our website. Click the link below to start your adventure: Visit Best Website meltwatermedia.ca. Don't miss out!
Table of Contents
Decoding the Hypothesis: Definition and 4 Steps to Successful Testing
What if the success of your research hinges on understanding how to formulate and test a robust hypothesis? A well-defined hypothesis, rigorously tested, is the cornerstone of scientific discovery and informed decision-making.
Editor’s Note: This article on hypothesis testing provides a comprehensive guide to understanding its definition and the four crucial steps involved in the process. We've included real-world examples to illustrate each stage, ensuring the latest insights and practical application.
Understanding the concept of a hypothesis and mastering the art of testing it is crucial across numerous fields. From scientific research and medical studies to business strategy and marketing campaigns, a well-defined hypothesis forms the bedrock of effective investigations and informed decision-making. Its importance lies in its ability to transform vague questions into testable propositions, guiding the research process and facilitating the extraction of meaningful conclusions. This article delves into the core aspects of hypothesis testing, exploring its definition, real-world applications, potential challenges, and the steps involved in rigorous testing. Backed by examples and a structured approach, it provides actionable knowledge for students, researchers, and professionals alike.
This article will explore the following:
- Definition and Core Concepts: We will define a hypothesis, differentiate between different types, and understand its role in the scientific method.
- Applications Across Industries: We will see how hypothesis testing is applied in diverse fields such as medicine, marketing, and engineering.
- Four Steps to Testing a Hypothesis: We'll break down the process into four clear and manageable steps, each illustrated with a detailed example.
- Challenges and Solutions: We will identify common obstacles encountered during hypothesis testing and suggest strategies for overcoming them.
- Impact on Innovation: We will explore how rigorous hypothesis testing drives innovation and progress across various sectors.
Key Takeaways:
Key Concept | Description | Example |
---|---|---|
Hypothesis Definition | A testable statement predicting a relationship between variables. | "Increased social media engagement leads to higher brand awareness." |
Null Hypothesis (H0) | A statement of no effect or no relationship between variables. | "There is no relationship between social media engagement and brand awareness." |
Alternative Hypothesis (H1) | A statement proposing an effect or relationship between variables. | "Increased social media engagement leads to higher brand awareness." |
Four Steps of Hypothesis Testing | 1. State Hypothesis, 2. Design Study, 3. Analyze Data, 4. Interpret Results | See detailed examples in the subsequent sections. |
Type I Error | Rejecting the null hypothesis when it's actually true (false positive). | Concluding social media engagement boosts brand awareness when it doesn't. |
Type II Error | Failing to reject the null hypothesis when it's false (false negative). | Failing to detect a real relationship between social media and brand awareness. |
With a strong understanding of its relevance, let's explore hypothesis testing further, uncovering its applications, challenges, and future implications.
Defining a Hypothesis: The Foundation of Inquiry
A hypothesis is a testable statement that proposes a relationship between two or more variables. It's more than just a guess; it's a specific, measurable prediction based on existing knowledge and observations. Crucially, a hypothesis must be falsifiable—meaning it's possible to design an experiment or study that could disprove it. This falsifiability is what separates scientific hypotheses from mere speculation.
There are two main types of hypotheses:
- Null Hypothesis (H0): This hypothesis states that there is no significant relationship between the variables being studied. It's often the default assumption until proven otherwise.
- Alternative Hypothesis (H1): This hypothesis proposes a specific relationship between the variables. It directly contradicts the null hypothesis.
For example, consider the hypothesis: "Regular exercise improves cardiovascular health."
- H0 (Null Hypothesis): Regular exercise does not improve cardiovascular health.
- H1 (Alternative Hypothesis): Regular exercise improves cardiovascular health.
This distinction is critical because statistical tests are designed to assess the evidence against the null hypothesis. If the evidence is strong enough, we reject the null hypothesis in favor of the alternative hypothesis.
Applications Across Industries: A Versatile Tool
Hypothesis testing is not confined to the laboratory. Its applications span a wide range of fields:
- Medicine: Testing the effectiveness of new drugs or treatments. For example, a hypothesis might be: "A new drug will reduce blood pressure more effectively than the current standard treatment."
- Marketing: Evaluating the impact of advertising campaigns on sales. A hypothesis could be: "An increase in online advertising spending will lead to a proportional increase in website traffic and sales."
- Engineering: Assessing the performance of new materials or designs. A hypothesis might be: "A new composite material will increase the tensile strength of a bridge by 20%."
- Social Sciences: Investigating the correlation between social factors and behaviors. For instance: "Increased access to education correlates with reduced crime rates."
Four Steps to Testing a Hypothesis: A Practical Guide
Testing a hypothesis involves a systematic process typically involving these four steps:
Step 1: State the Hypothesis: This involves clearly defining the null and alternative hypotheses. Ensure your hypotheses are specific, measurable, achievable, relevant, and time-bound (SMART).
Example: Let's say we want to test the hypothesis that listening to classical music improves concentration levels in students.
- H0: Listening to classical music has no effect on student concentration levels.
- H1: Listening to classical music improves student concentration levels.
Step 2: Design the Study: This involves planning the research methodology, including:
- Sampling: How will you select participants for your study? A random sample is generally preferred to minimize bias.
- Data Collection: What methods will you use to measure the variables (e.g., questionnaires, tests, observations)? Ensure your measurement tools are reliable and valid.
- Experimental Design: If conducting an experiment, how will you manipulate the independent variable (classical music exposure) and control for extraneous factors? A controlled experiment with a control group (no classical music) and an experimental group (listening to classical music) is ideal.
Example (continued): We might randomly assign students to two groups: a control group that studies in silence and an experimental group that studies while listening to classical music. We'd measure their concentration levels using a standardized test before and after the study period.
Step 3: Analyze the Data: This involves using statistical methods to analyze the collected data. The choice of statistical test depends on the type of data and the research design. Common tests include t-tests, ANOVA, and chi-square tests.
Example (continued): After the study, we'd compare the changes in concentration scores between the two groups using a paired t-test. This test would determine if the difference in concentration improvements between the two groups is statistically significant.
Step 4: Interpret the Results: Based on the statistical analysis, we either reject or fail to reject the null hypothesis. A statistically significant result (typically p < 0.05) provides evidence against the null hypothesis, suggesting support for the alternative hypothesis. However, it's crucial to remember that correlation doesn't equal causation. Even with a significant result, other factors might be influencing the relationship. It’s also important to consider effect size and practical significance beyond statistical significance.
Example (continued): If the t-test reveals a statistically significant difference in concentration improvements favoring the experimental group (p < 0.05), we would reject the null hypothesis and conclude that listening to classical music likely improves student concentration. However, we must also consider the magnitude of the improvement (effect size) to assess the practical significance of the findings.
Challenges and Solutions in Hypothesis Testing
Several challenges can arise during hypothesis testing:
- Bias: Researchers' preconceived notions or expectations can influence the design and interpretation of the study. Blind and double-blind studies help mitigate this.
- Sample Size: A small sample size can lead to inaccurate conclusions. Power analysis can help determine the appropriate sample size needed to detect a meaningful effect.
- Confounding Variables: Uncontrolled variables can affect the results, making it difficult to isolate the effect of the independent variable. Careful experimental design and statistical control techniques can help address this.
The Impact of Hypothesis Testing on Innovation
Rigorous hypothesis testing drives innovation by allowing researchers to systematically evaluate new ideas and approaches. By testing predictions and identifying what works and what doesn't, we can refine theories, develop better products and services, and make more informed decisions across various sectors.
Exploring the Relationship Between Sample Size and Hypothesis Testing Accuracy
The relationship between sample size and hypothesis testing accuracy is crucial. Larger sample sizes generally lead to more reliable results. Smaller samples are more susceptible to random variations and may not accurately reflect the population being studied. This can lead to Type I errors (false positives) or Type II errors (false negatives).
Roles and Real-World Examples: Consider a drug trial. A small sample size might fail to detect a genuine effect of the drug (Type II error), leading to its rejection despite potential benefits. Conversely, a small sample size might show a positive effect by chance (Type I error), leading to the approval of an ineffective drug.
Risks and Mitigations: The risk of inaccurate results increases with smaller sample sizes. Mitigation involves performing a power analysis to determine the minimum sample size needed to detect a meaningful effect with a desired level of confidence.
Impact and Implications: The choice of sample size directly influences the credibility and generalizability of the research findings. Insufficient sample sizes can lead to wasted resources and flawed policy decisions.
Frequently Asked Questions (FAQs) about Hypothesis Testing
-
What is a p-value? The p-value represents the probability of obtaining the observed results (or more extreme results) if the null hypothesis is true. A low p-value (typically below 0.05) suggests strong evidence against the null hypothesis.
-
What is statistical significance? Statistical significance means that the observed results are unlikely to have occurred by chance alone. It indicates that there's a real effect, but the magnitude of the effect (effect size) needs separate consideration.
-
What is a Type I error? A Type I error (false positive) occurs when you reject the null hypothesis when it's actually true.
-
What is a Type II error? A Type II error (false negative) occurs when you fail to reject the null hypothesis when it's actually false.
-
How do I choose the right statistical test? The choice of statistical test depends on the type of data (e.g., continuous, categorical), the research design (e.g., experimental, observational), and the hypotheses being tested.
-
What is effect size? Effect size measures the magnitude of the difference or relationship between variables. It provides a more comprehensive understanding of the practical significance of the findings beyond just statistical significance.
Practical Tips for Maximizing the Benefits of Hypothesis Testing:
- Clearly Define Your Research Question: Ensure your research question is specific and measurable.
- Formulate Testable Hypotheses: Ensure your hypotheses are specific, measurable, achievable, relevant, and time-bound (SMART).
- Choose the Appropriate Statistical Test: Select the test based on your data type, research design, and hypotheses.
- Control for Confounding Variables: Account for variables that might influence your results.
- Use a Sufficient Sample Size: Conduct a power analysis to determine the appropriate sample size.
- Interpret Results Carefully: Consider both statistical and practical significance.
- Replicate Your Study: Repeating the study can help validate your findings and increase confidence in your conclusions.
- Consider Limitations: Acknowledge any limitations of your study and how they might affect the interpretation of your findings.
Conclusion: The Power of Rigorous Inquiry
Hypothesis testing, with its rigorous methodology and reliance on empirical evidence, is an indispensable tool for advancing knowledge and driving innovation. By following a systematic approach and carefully interpreting the results, researchers and professionals can use hypothesis testing to make informed decisions and contribute to progress across a wide range of fields. The ongoing refinement of statistical methods and the increased accessibility of data analysis tools continue to enhance the power and applicability of hypothesis testing, solidifying its role as a cornerstone of evidence-based decision-making. The journey from a simple question to a substantiated conclusion, guided by the principles of hypothesis testing, highlights the power of methodical inquiry in unlocking new understanding and shaping a better future.
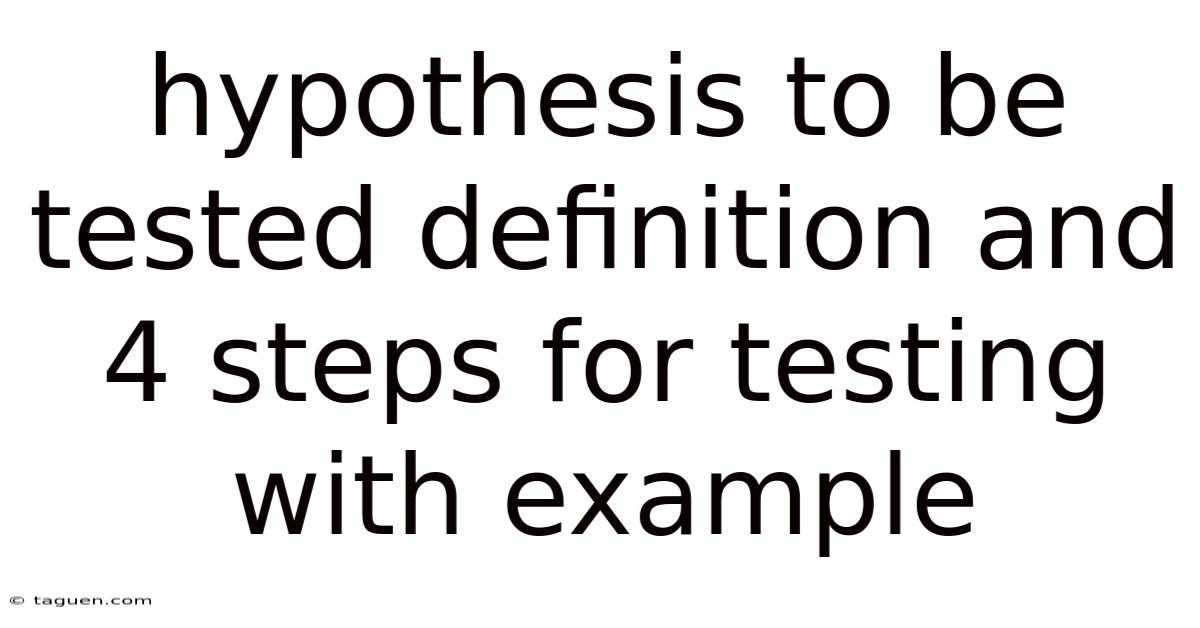
Thank you for visiting our website wich cover about Hypothesis To Be Tested Definition And 4 Steps For Testing With Example. We hope the information provided has been useful to you. Feel free to contact us if you have any questions or need further assistance. See you next time and dont miss to bookmark.
Also read the following articles
Article Title | Date |
---|---|
Immunization Definition Investing Strategies And Examples | Apr 19, 2025 |
What Is Identity Theft Definition Types And Examples | Apr 19, 2025 |
Implicit Rental Rate Definition | Apr 19, 2025 |
Indexing Definition And Uses In Economics And Investing | Apr 19, 2025 |
Installment Sale Definition And How Its Used In Accounting | Apr 19, 2025 |