Heteroscedasticity Definition Simple Meaning And Types Explained
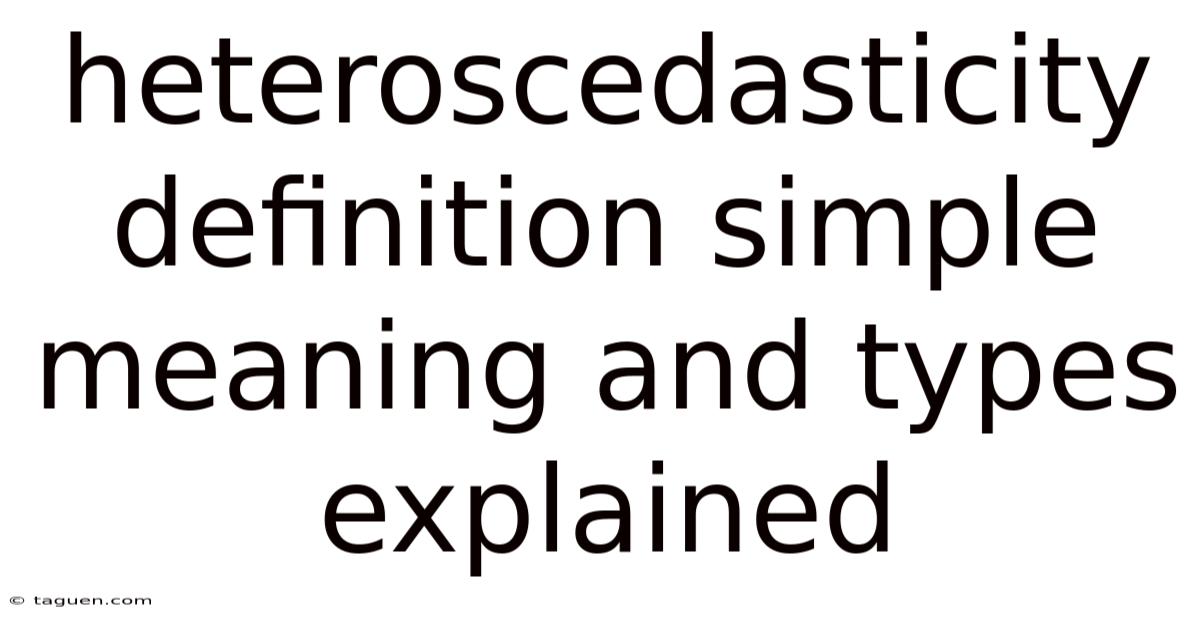
Discover more detailed and exciting information on our website. Click the link below to start your adventure: Visit Best Website meltwatermedia.ca. Don't miss out!
Table of Contents
Unveiling Heteroscedasticity: Simple Meaning, Types, and Impacts
What if the accuracy of your statistical models hinges on understanding a seemingly complex concept like heteroscedasticity? This often-overlooked statistical phenomenon can significantly impact the reliability of your analyses, leading to inaccurate conclusions and flawed predictions.
Editor’s Note: This article on heteroscedasticity was published today, providing the latest insights and expert analysis into this crucial statistical concept.
Heteroscedasticity, a term that might sound intimidating, is a fundamental concept in statistics, particularly in regression analysis. Simply put, it refers to the unequal spread or variability of a variable across different values of another variable. In regression, this translates to the variability of the error terms (residuals) not being constant across all levels of the independent variable(s). Understanding heteroscedasticity is crucial because its presence can invalidate the assumptions of many statistical tests, leading to unreliable results. This article will delve into the core aspects of heteroscedasticity, examining its definition, types, detection methods, consequences, and remediation techniques.
This article will explore the core aspects of heteroscedasticity, examining its definition, real-world applications, detection methods, consequences, and effective solutions. Backed by expert insights and data-driven research, it provides actionable knowledge for statisticians, researchers, and anyone working with statistical models.
Understanding Heteroscedasticity: Definition and Core Concepts
Heteroscedasticity (from Greek: hetero – different, skedasis – dispersion) essentially means "unequal scatter." In the context of regression analysis, it signifies that the variance of the error term (the difference between the observed value and the predicted value) is not constant across all levels of the independent variable(s). Imagine plotting the residuals against the predicted values or the independent variable. If heteroscedasticity is present, you'll see a pattern – the spread of the residuals will change systematically. For example, the spread might be small at lower values of the independent variable and become larger as the independent variable increases. This non-constant variance violates a key assumption of many statistical tests, specifically those based on ordinary least squares (OLS) estimation.
Types of Heteroscedasticity
Heteroscedasticity isn't a monolithic concept; it manifests in different forms. Understanding these variations is crucial for accurate diagnosis and effective treatment. We can broadly categorize heteroscedasticity as follows:
-
Linear Heteroscedasticity: This is the most common type, where the variance of the error term is linearly related to the independent variable(s). The spread of the residuals increases or decreases proportionally to the value of the independent variable. Graphically, this would appear as a cone-like shape, widening or narrowing as the independent variable changes.
-
Quadratic Heteroscedasticity: Here, the variance of the error term follows a quadratic relationship with the independent variable. This means the variance increases or decreases at an accelerating rate. Graphically, this would appear as a curve rather than a straight line.
-
Random Heteroscedasticity: In this case, the variance of the error term fluctuates randomly, without any discernible pattern or relationship with the independent variable. This type is more challenging to detect and address.
-
Clustering Heteroscedasticity: This type occurs when the variance differs significantly across distinct groups or clusters within the data. For example, you might find higher variance within one demographic group compared to another.
It is important to note that these categories are not mutually exclusive; complex datasets may exhibit combinations of these patterns.
Applications Across Industries
The implications of heteroscedasticity extend far beyond theoretical statistics. Its presence can significantly affect the reliability of results across diverse fields:
-
Finance: In financial modeling, heteroscedasticity can lead to inaccurate risk assessments and portfolio optimizations. The volatility of asset returns is often not constant over time, leading to heteroscedastic error terms in models predicting returns.
-
Economics: Economic models often encounter heteroscedasticity due to the inherent variability of economic indicators across different income levels, regions, or time periods. Incorrectly accounting for heteroscedasticity can lead to flawed policy recommendations.
-
Healthcare: In clinical trials or epidemiological studies, heteroscedasticity can arise due to variations in patient characteristics or treatment responses. Ignoring this can lead to biased estimates of treatment effects.
-
Environmental Science: Studies of environmental variables (e.g., pollution levels) often exhibit heteroscedasticity due to spatial or temporal variations in environmental conditions. This necessitates careful modeling to avoid spurious conclusions.
-
Marketing: Marketing research often deals with heteroscedastic data because customer responses to advertising campaigns vary based on demographics, geographic location, and other factors.
Detecting Heteroscedasticity
Several methods can be employed to detect the presence of heteroscedasticity:
-
Graphical Methods: Plotting the residuals against the predicted values or the independent variable is a simple yet effective visual check. Patterns in the spread of residuals (e.g., a cone shape, increasing spread) suggest heteroscedasticity.
-
Breusch-Pagan Test: This statistical test formally assesses the null hypothesis of homoscedasticity (constant variance). A low p-value indicates rejection of the null hypothesis, suggesting the presence of heteroscedasticity.
-
White Test: A more general test than the Breusch-Pagan test, the White test is less sensitive to specific assumptions about the form of heteroscedasticity.
-
Goldfeld-Quandt Test: This test divides the data into two groups based on the independent variable and compares the variances of the residuals in each group. A significant difference indicates heteroscedasticity.
-
Park Test: This test uses a regression model to assess the relationship between the squared residuals and the independent variable. A statistically significant relationship indicates heteroscedasticity.
Consequences of Ignoring Heteroscedasticity
Failing to address heteroscedasticity can have several serious consequences:
-
Inefficient Estimators: OLS estimators, while still unbiased, become inefficient in the presence of heteroscedasticity. This means the standard errors of the coefficients are underestimated, leading to inflated t-statistics and potentially inaccurate conclusions about statistical significance.
-
Inaccurate Confidence Intervals and p-values: Underestimated standard errors lead to narrower confidence intervals and smaller p-values than would be obtained with correctly modeled heteroscedasticity. This increases the risk of Type I errors (rejecting a true null hypothesis).
-
Flawed Hypothesis Testing: Incorrect p-values and confidence intervals lead to unreliable hypothesis testing, making it difficult to draw valid conclusions from statistical analyses.
-
Biased Predictions: Models with heteroscedasticity can generate unreliable predictions, particularly outside the range of observed data.
Addressing Heteroscedasticity
Several strategies can mitigate the effects of heteroscedasticity:
-
Weighted Least Squares (WLS): This method assigns weights to observations based on the estimated variance of the error term. Observations with higher variance receive lower weights, reducing their influence on the estimates.
-
Transformations: Transforming the dependent variable (e.g., using logarithmic or square root transformations) can sometimes stabilize the variance.
-
Robust Standard Errors: Employing robust standard error estimators (e.g., White's heteroscedasticity-consistent standard errors) corrects for the underestimated standard errors resulting from heteroscedasticity, without modifying the model itself.
-
Generalized Least Squares (GLS): GLS is a more general approach that takes into account the structure of the heteroscedasticity. It requires specifying a covariance matrix for the error terms.
The choice of method depends on the type and severity of heteroscedasticity, as well as the specific research question.
The Relationship Between Outliers and Heteroscedasticity
Outliers, data points that lie far from the rest of the data, can contribute to heteroscedasticity. The presence of outliers can inflate the variance of the error term, particularly in the regions of the independent variable where these outliers occur. This makes it crucial to identify and address outliers appropriately before dealing with heteroscedasticity. Techniques such as winsorizing or trimming outliers can help mitigate this issue. However, it’s essential to carefully consider the potential implications of removing outliers, as they may represent genuine, albeit extreme, data points.
Heteroscedasticity in Time Series Data
Heteroscedasticity is particularly relevant in time series analysis, where the variance of the error term can change over time. This is often due to changes in economic conditions, market volatility, or other external factors. ARCH (Autoregressive Conditional Heteroscedasticity) and GARCH (Generalized Autoregressive Conditional Heteroscedasticity) models are specifically designed to account for this type of heteroscedasticity. These models explicitly model the changing variance of the error term over time.
Frequently Asked Questions about Heteroscedasticity
Q1: Is heteroscedasticity always a problem?
Not always. In some cases, the impact of heteroscedasticity might be minimal, particularly if the sample size is large. However, it's always advisable to check for heteroscedasticity and address it if it significantly affects the results.
Q2: Can I ignore heteroscedasticity if my p-values are still significant?
No. Even if your p-values suggest statistical significance, the presence of heteroscedasticity can lead to inaccurate estimations of the standard errors and confidence intervals. This can lead to overconfidence in the results.
Q3: Which test is best for detecting heteroscedasticity?
There's no single "best" test. The choice depends on the specific data and the suspected form of heteroscedasticity. Graphical methods are a good starting point, followed by formal tests like the Breusch-Pagan, White, or Goldfeld-Quandt test.
Q4: How do I choose the right method to address heteroscedasticity?
The best method depends on the severity and nature of the heteroscedasticity. WLS is a common approach for linear heteroscedasticity, while robust standard errors are a simple solution if the heteroscedasticity is not severe. For complex cases, GLS or transformations might be necessary.
Q5: Can heteroscedasticity be present in non-linear regression models?
Yes, heteroscedasticity can occur in any type of regression model, including non-linear models. The same principles and detection/remediation methods generally apply.
Q6: What if my data has both heteroscedasticity and autocorrelation?
This scenario is common in time series analysis. You'll need to address both issues simultaneously, potentially using specialized models like GARCH models that can handle both heteroscedasticity and autocorrelation.
Practical Tips for Handling Heteroscedasticity
- Visual Inspection: Always start with graphical methods to visualize the spread of residuals.
- Formal Testing: Employ statistical tests like the Breusch-Pagan or White test to confirm the presence of heteroscedasticity.
- Data Transformation: Consider transforming your variables (e.g., logarithmic, square root) to stabilize variance.
- Weighted Least Squares: Use WLS if the heteroscedasticity is linear and you have an understanding of the variance structure.
- Robust Standard Errors: Employ robust standard errors as a simple and effective solution in many cases.
- Generalized Least Squares: Use GLS for complex heteroscedasticity patterns.
- Model Specification: Re-evaluate your model specification to ensure it's appropriately capturing the relationships between variables.
- Outlier Analysis: Carefully examine your data for outliers, and consider appropriate techniques to handle them.
Conclusion: Navigating the World of Unequal Variance
Heteroscedasticity, while a seemingly complex concept, is a crucial element in statistical modeling. Understanding its implications and employing appropriate detection and remediation techniques is vital for ensuring the accuracy and reliability of your analyses. By combining visual inspection with formal statistical tests, and by judiciously selecting appropriate remedial actions, researchers and analysts can navigate the complexities of unequal variance and obtain more robust and reliable insights from their data. The prevalence of heteroscedasticity across diverse fields underlines the need for continued vigilance and the application of appropriate statistical tools to ensure the validity of research findings and predictions. The ongoing development of new statistical methods to address heteroscedasticity highlights the enduring relevance of this crucial concept in the realm of statistical inference.
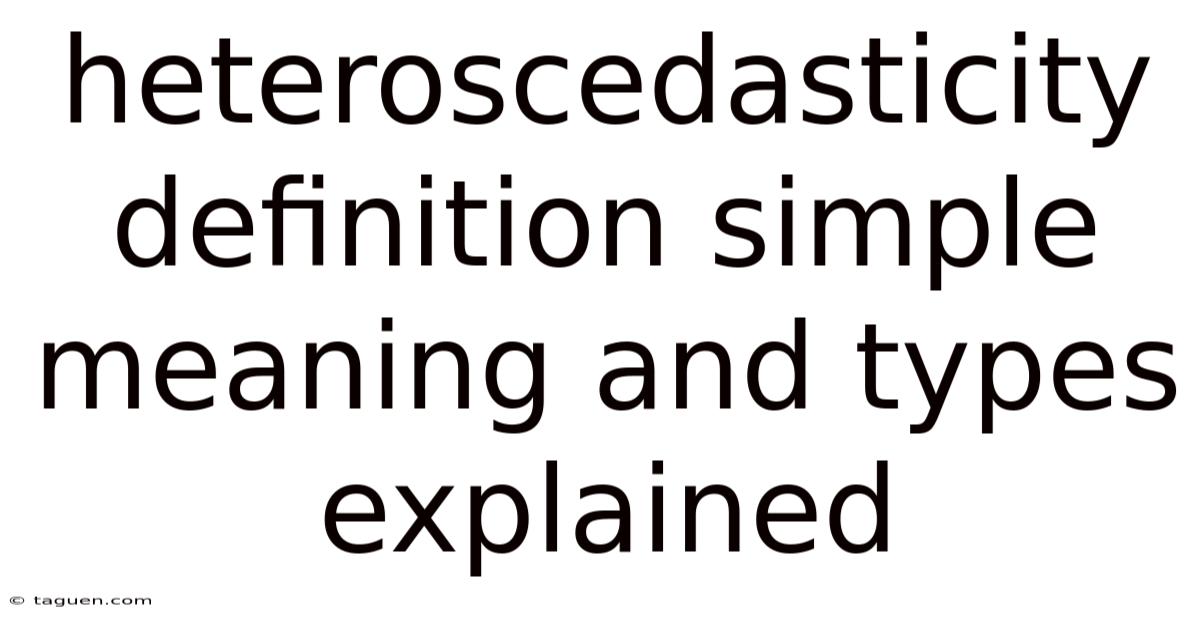
Thank you for visiting our website wich cover about Heteroscedasticity Definition Simple Meaning And Types Explained. We hope the information provided has been useful to you. Feel free to contact us if you have any questions or need further assistance. See you next time and dont miss to bookmark.
Also read the following articles
Article Title | Date |
---|---|
Grading Certificate Definition | Apr 18, 2025 |
Hospital Revenue Bond Definition | Apr 18, 2025 |
Hollowing Out Definition | Apr 18, 2025 |
High Low Index Definition Formula Example Chart | Apr 18, 2025 |
Harmonic Mean Definition Formula And Examples | Apr 18, 2025 |