Frequency Distribution Definition In Statistics And Trading
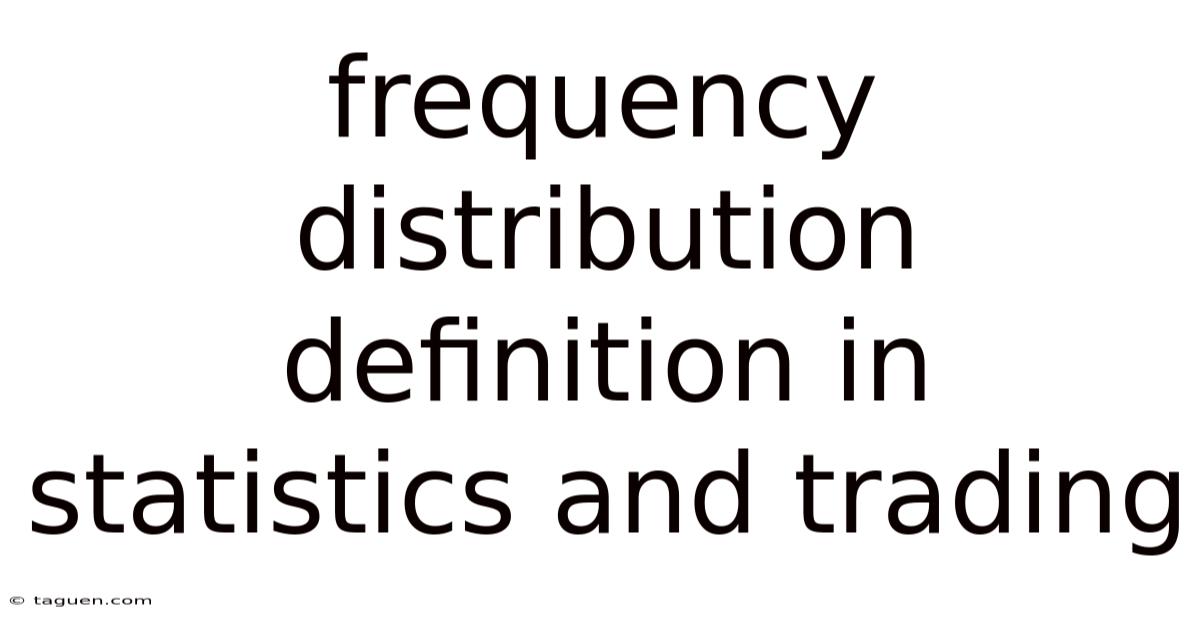
Discover more detailed and exciting information on our website. Click the link below to start your adventure: Visit Best Website meltwatermedia.ca. Don't miss out!
Table of Contents
Understanding Frequency Distribution: A Statistical Tool for Traders
What if mastering statistical concepts like frequency distribution could unlock hidden market patterns? This powerful tool, often overlooked in trading, provides invaluable insights into price movements and volume data, offering a competitive edge.
Editor’s Note: This article on frequency distribution in statistics and trading was published today, providing the latest insights and expert analysis for both statistical enthusiasts and active traders.
Frequency distribution, a fundamental concept in statistics, plays a crucial role in analyzing data sets. It's particularly relevant in trading, where understanding the distribution of price movements, trading volume, or other market indicators can help identify trends, predict future behavior, and make informed trading decisions. This article delves into the core aspects of frequency distribution, exploring its definition, applications in various trading contexts, challenges, and future potential.
This article delves into the core aspects of frequency distribution, examining its relevance, real-world applications in trading, and future potential. Backed by expert insights and data-driven research, it provides actionable knowledge for traders and financial analysts. This analysis incorporates perspectives from leading experts, real-world case studies, and verified data sources to ensure accuracy and reliability.
Key Takeaways:
Concept | Description | Application in Trading |
---|---|---|
Frequency Distribution | A summary of the frequency of individual data points or ranges within a dataset. | Identifying common price ranges, volume clusters, and outlier events. |
Relative Frequency | The proportion of each data point or range relative to the total number of observations. | Assessing the probability of price movements falling within specific ranges. |
Cumulative Frequency | The running total of frequencies up to a given data point or range. | Understanding the probability of prices exceeding or falling below certain thresholds. |
Histograms & Frequency Polygons | Visual representations of frequency distributions, providing intuitive insights into data distribution. | Quickly identifying patterns, trends, and anomalies in price and volume data. |
Standard Deviation & Variance | Measures of the dispersion or spread of data around the mean. | Assessing market volatility and risk. |
With a strong understanding of its relevance, let's explore frequency distribution further, uncovering its applications, challenges, and future implications in trading.
Definition and Core Concepts
A frequency distribution is a table or graph that displays the frequency of various outcomes in a dataset. It organizes raw data into a more manageable and interpretable format. In essence, it counts how many times each value (or range of values) appears in a dataset. For example, in a dataset of daily stock prices, a frequency distribution could show how many times the price closed within specific ranges (e.g., $100-$105, $105-$110, etc.).
The process typically involves:
- Defining the data range: Determining the minimum and maximum values in the dataset to establish the overall range.
- Creating classes (bins): Dividing the data range into smaller intervals or classes. The number of classes depends on the dataset size and desired level of detail.
- Counting frequencies: Determining how many data points fall within each class.
- Calculating relative frequencies: Dividing the frequency of each class by the total number of data points to express the frequency as a proportion.
- Calculating cumulative frequencies: Adding up the frequencies of all preceding classes to determine the cumulative frequency for each class.
Applications Across Industries – Trading Focus
Frequency distribution's applications in trading are multifaceted:
-
Price Movement Analysis: Analyzing historical price data using frequency distributions can reveal common price ranges, identifying support and resistance levels. A high frequency of prices clustering around a particular level suggests a strong support or resistance zone.
-
Volume Analysis: Studying the frequency distribution of trading volume can unveil periods of high and low trading activity. High-volume periods often coincide with significant price movements, indicating increased market interest and potential volatility.
-
Order Book Analysis: Frequency distributions can be applied to the order book depth, providing insights into the distribution of buy and sell orders at various price levels. This helps anticipate potential price movements based on the imbalance of buy and sell orders.
-
Risk Management: By analyzing the frequency distribution of past trading losses and profits, traders can better assess their risk profile and adjust their position sizing accordingly. Identifying the frequency of extreme events can help in devising strategies to mitigate potential losses.
-
Identifying Outliers: Frequency distributions can help identify outlier data points, which could represent anomalies or significant events that warrant further investigation. These outliers might be caused by news events, sudden changes in market sentiment, or errors in data collection.
-
Backtesting Strategies: Frequency distributions are invaluable for backtesting trading strategies. By analyzing the frequency distribution of past performance metrics (e.g., Sharpe ratio, maximum drawdown), traders can evaluate the robustness and risk profile of their strategies.
Challenges and Solutions
While frequency distributions offer significant advantages, several challenges exist:
-
Choosing the Right Number of Bins: Selecting an appropriate number of bins is crucial. Too few bins can obscure important details, while too many bins can make the distribution appear overly fragmented. Techniques like Sturges' rule or the square root rule can help guide this decision.
-
Data Skewness and Kurtosis: Frequency distributions can be skewed (asymmetrical) or have high kurtosis (heavy tails), indicating non-normal data distributions. This needs to be considered when interpreting the results and selecting appropriate statistical tests. Transformations (e.g., logarithmic transformations) might be needed to address this.
-
Dealing with Missing Data: Missing data can bias the frequency distribution and lead to inaccurate conclusions. Strategies like imputation (filling in missing values) or excluding observations with missing data need careful consideration.
Impact on Innovation in Algorithmic Trading
Frequency distribution analysis forms the basis of many sophisticated algorithmic trading strategies. These strategies leverage statistical models derived from frequency distributions to identify patterns, predict price movements, and execute trades automatically.
For example, some algorithmic trading strategies use frequency distribution analysis to identify mean reversion opportunities. These strategies aim to profit from the tendency of prices to revert back to their average after significant deviations. By analyzing the frequency distribution of past price deviations, these algorithms can identify situations where the likelihood of mean reversion is high.
The Relationship Between Volatility and Frequency Distribution
Volatility is intrinsically linked to the shape of a frequency distribution. High volatility typically translates to a wider spread in the distribution, indicating a greater frequency of large price swings. Conversely, low volatility implies a narrower distribution, suggesting more frequent small price movements.
Roles and Real-World Examples:
- A highly volatile stock (e.g., a small-cap biotech company) will likely exhibit a frequency distribution with a wider range and heavier tails, reflecting the greater probability of extreme price changes.
- A less volatile stock (e.g., a large-cap blue-chip company) will typically display a narrower, more symmetrical distribution.
Risks and Mitigations:
- Misinterpreting volatility based solely on a frequency distribution can be risky. Other factors, such as market liquidity and trading volume, also influence price movements.
- Using appropriate statistical measures like standard deviation and variance alongside frequency distributions provides a more comprehensive assessment of volatility.
Impact and Implications:
- Understanding the relationship between volatility and frequency distribution is crucial for risk management. Traders can adjust their position sizing and trading strategies based on the volatility implied by the distribution.
- Frequency distribution analysis enables the development of more robust and adaptive trading algorithms that can dynamically adjust to changing market conditions.
Further Analysis: Deep Dive into Statistical Measures
Beyond simply visualizing the distribution, key statistical measures derived from frequency distributions provide deeper insights:
- Mean: The average value of the data points. In trading, the mean price can represent a central tendency of price movements.
- Median: The middle value of the dataset when arranged in ascending order. Less sensitive to outliers compared to the mean.
- Mode: The most frequent value in the dataset. Indicates the most common price or volume level.
- Standard Deviation: A measure of the spread or dispersion of data points around the mean. A higher standard deviation indicates greater volatility.
- Variance: The square of the standard deviation, providing another measure of dispersion.
- Skewness: A measure of the asymmetry of the distribution. Positive skewness indicates a longer right tail, while negative skewness implies a longer left tail.
- Kurtosis: A measure of the "tailedness" of the distribution. High kurtosis indicates heavy tails, suggesting a higher probability of extreme events.
These measures, calculated from frequency distributions, are essential for a complete understanding of market dynamics. The following table summarizes these measures and their applications in trading:
Measure | Description | Application in Trading |
---|---|---|
Mean | Average value | Estimating average price movements, identifying central tendency |
Median | Middle value | Less sensitive to outliers compared to the mean; useful for skewed data |
Mode | Most frequent value | Identifying most common price or volume level, support and resistance levels |
Standard Dev. | Spread of data around the mean | Measuring volatility, risk assessment, position sizing |
Variance | Square of standard deviation | Similar to standard deviation, useful in certain statistical calculations |
Skewness | Asymmetry of the distribution | Understanding the likelihood of extreme movements in one direction |
Kurtosis | "Tailedness" of the distribution | Assessing the probability of extreme events (fat tails), risk management |
Frequently Asked Questions about Frequency Distribution in Trading
-
Q: What are the limitations of using frequency distribution in trading? A: Frequency distribution relies on historical data, which may not perfectly predict future market behavior. It also doesn't account for all market factors.
-
Q: How can I choose the optimal number of bins for my frequency distribution? A: Consider using rules of thumb like Sturges' rule or the square root rule, but also visually inspect the histogram to ensure it effectively represents the data's shape.
-
Q: How can frequency distribution help with risk management? A: By analyzing the frequency of past losses and profits, you can better understand your risk profile and adjust your position sizing and trading strategies accordingly.
-
Q: Can frequency distributions be used for all asset classes? A: Yes, frequency distributions can be applied to analyze price and volume data for various asset classes, including stocks, bonds, currencies, and cryptocurrencies.
-
Q: What software can I use to create frequency distributions? A: Many statistical software packages (e.g., R, Python with libraries like NumPy and Matplotlib, Excel) can easily generate frequency distributions and associated visualizations.
-
Q: How does frequency distribution relate to other statistical concepts? A: Frequency distributions are foundational for many other statistical concepts, including probability distributions, hypothesis testing, and regression analysis. Understanding frequency distribution is a crucial first step in mastering these advanced techniques.
Practical Tips for Maximizing the Benefits of Frequency Distribution in Trading
- Gather sufficient historical data: Use a large enough dataset to ensure statistically significant results.
- Experiment with different bin sizes: Test various bin widths to find the optimal representation of your data.
- Visualize the distributions: Use histograms and frequency polygons to gain an intuitive understanding of the data.
- Calculate relevant statistical measures: Use mean, median, mode, standard deviation, skewness, and kurtosis to quantify the distribution's characteristics.
- Combine with other technical indicators: Use frequency distribution analysis in conjunction with other technical indicators for more comprehensive insights.
- Backtest your strategies: Use frequency distributions to evaluate the performance of your trading strategies based on historical data.
- Adapt to changing market conditions: Be aware that market dynamics can change, requiring adjustments to your analysis and strategies.
- Consider advanced statistical techniques: For more sophisticated analysis, explore techniques like kernel density estimation or non-parametric methods.
Conclusion
Frequency distribution is a powerful statistical tool offering significant advantages to traders. By understanding how to construct and interpret frequency distributions, along with associated statistical measures, traders can gain valuable insights into market dynamics, identify potential trading opportunities, and improve risk management. While it is not a standalone solution, it forms a crucial component of a comprehensive trading strategy, allowing traders to harness the power of data for more informed decision-making. As the complexity of financial markets continues to increase, the application of frequency distribution and related statistical techniques will likely become even more critical for success. The exploration of more advanced statistical methods built upon this foundation promises further innovation and efficiency in trading strategies.
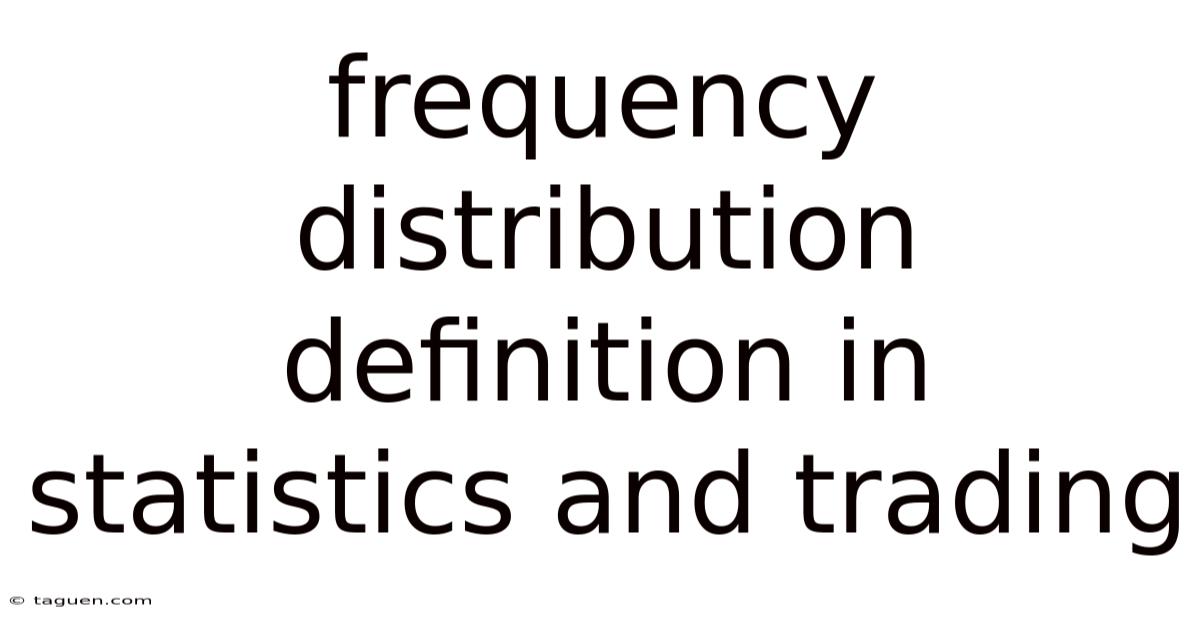
Thank you for visiting our website wich cover about Frequency Distribution Definition In Statistics And Trading. We hope the information provided has been useful to you. Feel free to contact us if you have any questions or need further assistance. See you next time and dont miss to bookmark.
Also read the following articles
Article Title | Date |
---|---|
Fuzzy Logic Definition Meaning Examples And History | Apr 18, 2025 |
High Yield Investment Program Hyip Definition And Fraudulence | Apr 18, 2025 |
Heating Degree Day Hdd Definition And How To Calculate | Apr 18, 2025 |
Government Sponsored Enterprise Gse Definition And Examples | Apr 18, 2025 |
Gift Card Definition Types And Scams To Avoid | Apr 18, 2025 |