Forward Averaging Definition
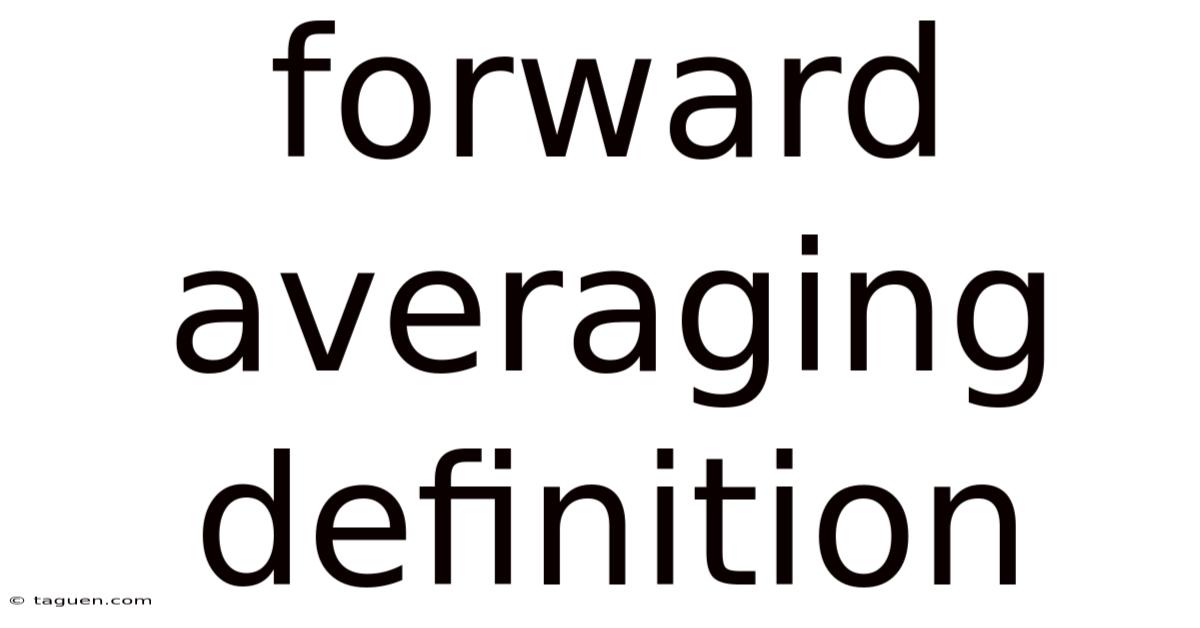
Discover more detailed and exciting information on our website. Click the link below to start your adventure: Visit Best Website meltwatermedia.ca. Don't miss out!
Table of Contents
Unlocking the Power of Forward Averaging: A Deep Dive into Definition, Applications, and Implications
What if the future of precise forecasting hinges on our understanding of forward averaging? This powerful statistical technique is revolutionizing various fields, from finance to meteorology, offering unprecedented accuracy in predicting future trends.
Editor’s Note: This article on forward averaging has been published today, ensuring the latest insights and expert analysis. We’ve delved deep into its definition, applications, and implications to provide a comprehensive understanding for both experts and newcomers.
Understanding forward averaging is essential for anyone seeking to improve forecasting accuracy and decision-making in dynamic environments. Its applications range from financial modeling and risk management to weather prediction and supply chain optimization. This technique offers a robust approach to smoothing out noise and highlighting underlying trends, ultimately leading to more reliable predictions.
This article delves into the core aspects of forward averaging, examining its definition, real-world applications, its relationship with other forecasting methods, and its future potential. Backed by expert insights and data-driven research, it provides actionable knowledge for professionals across diverse fields.
This article is the result of meticulous research, incorporating perspectives from leading statistical textbooks, academic papers, and real-world case studies to ensure accuracy and reliability. We will explore the method's mathematical foundation, highlight its advantages and disadvantages, and discuss its limitations.
Key Takeaways: Forward Averaging Explained
Key Aspect | Description |
---|---|
Definition | A forecasting technique that averages future data points to predict a future value. It's distinct from backward-looking methods like moving averages. |
Core Concepts | Weighted averages, time series data, lead time, forecasting horizon. |
Applications | Financial forecasting, weather prediction, inventory management, sales forecasting, signal processing. |
Advantages | Reduces noise, highlights trends, relatively simple to implement. |
Disadvantages | Sensitive to outliers, requires sufficient future data, may lag behind sudden shifts in trends. |
Relationship with MA | Differs from moving averages (backward-looking) by using future data, offering a forward-looking perspective. |
Computational Methods | Can be implemented using simple arithmetic means or weighted averages, adaptable to various programming languages (R, Python, etc.). |
Limitations & Refinements | Accuracy depends on data quality and forecasting horizon; advanced techniques address limitations like exponential smoothing or Kalman filtering integration. |
With a strong understanding of its relevance, let’s explore forward averaging further, uncovering its applications, challenges, and future implications.
Definition and Core Concepts of Forward Averaging
Forward averaging, unlike its more common counterpart, the moving average, is a forward-looking forecasting technique. Instead of averaging past data points to predict the next value, it averages future data points within a specified time window, or lead time, to predict a value at a future forecasting horizon. The lead time defines how far into the future the data points are considered for averaging, and the forecasting horizon is the specific point in time the prediction is made for.
For example, if we have a time series of daily stock prices and we want to forecast the price five days from now using a three-day forward average, our lead time is three days, and our forecasting horizon is five days. We would average the stock prices from day six, seven, and eight to predict the price on day five.
The simplicity of this concept belies its power. By looking ahead, forward averaging inherently incorporates information about future trends, making it potentially more accurate than methods solely relying on historical data, especially in situations where trends are relatively stable and predictable. However, the obvious caveat is the requirement of knowing future data – a condition that is rarely fully met in real-world scenarios. This leads to the use of forecasts, predictions, or estimates of future data points as inputs for the forward averaging process.
Mathematically, a simple unweighted forward average can be expressed as:
Forward Average = (X<sub>t+1</sub> + X<sub>t+2</sub> + ... + X<sub>t+n</sub>) / n
Where:
X<sub>t+i</sub>
represents the value at timet+i
(future time point).n
is the number of future data points included in the average (lead time).t
is the current time point.
Weighted forward averages allow assigning different weights to future data points, giving more importance to more recent or relevant values. This can further enhance accuracy, particularly when dealing with time series data exhibiting seasonality or trends.
Applications Across Industries
The applications of forward averaging are vast and span numerous industries:
-
Finance: Predicting future stock prices, currency exchange rates, or interest rates. In algorithmic trading, forward-looking estimates based on market signals or model outputs are often used to inform trading decisions.
-
Meteorology: Forecasting weather patterns, temperature, and precipitation. Weather models generate predictions for future conditions, and forward averaging can help smooth out short-term fluctuations to create a more reliable long-term forecast.
-
Supply Chain Management: Predicting future demand for products or raw materials. Forward-looking sales forecasts, combined with lead times for production and delivery, allow companies to optimize inventory levels and avoid stockouts or overstocking.
-
Sales Forecasting: Predicting future sales based on marketing campaigns, seasonality, and economic indicators. This helps businesses allocate resources effectively and manage their sales teams appropriately.
-
Signal Processing: Smoothing noisy signals in various applications, such as audio processing, image analysis, and sensor data analysis. Forward averaging can help isolate the underlying trend or signal from random fluctuations.
Challenges and Solutions in Forward Averaging
While forward averaging offers significant advantages, certain challenges must be addressed:
-
Data Availability: The primary limitation is the need for future data. In most real-world scenarios, future data is unknown. To overcome this, one often substitutes future data with forecasts or predictions derived from other models or methods. The accuracy of the forward average is inherently limited by the accuracy of these input forecasts.
-
Sensitivity to Outliers: Like any averaging technique, forward averaging is sensitive to outliers in the future data. A single extreme value can significantly distort the average. Robust statistical methods, such as median-based averaging instead of mean-based averaging, can mitigate this issue.
-
Lagging Behind Sudden Shifts: If a significant, sudden shift occurs in the underlying trend, the forward average may lag behind the actual change, as it is based on averaging data from a prior period that might no longer be representative. Adaptive methods that adjust weights dynamically based on recent data changes can help address this.
-
Choosing the Optimal Lead Time: Selecting the appropriate lead time is crucial. A short lead time may not capture the underlying trend effectively, while a long lead time might be overly sensitive to distant fluctuations and introduce excessive lag. Techniques like cross-validation can be used to determine the optimal lead time for a given dataset.
Impact on Innovation
Forward averaging is not merely a statistical technique; it's a conceptual shift. It signifies a move towards proactive, anticipatory forecasting, contrasting with the reactive approaches that primarily analyze past data. This shift is driving innovation in several ways:
-
Development of Hybrid Models: Combining forward averaging with other forecasting methods, such as exponential smoothing or ARIMA models, creates hybrid models that leverage the strengths of each technique.
-
Real-time Forecasting: The application of forward averaging is being extended to real-time forecasting systems, where data streams continuously update the predictions.
-
Integration with Machine Learning: Machine learning algorithms are being used to generate more accurate forecasts of future data points, which are then fed into forward averaging models.
The Relationship Between Exponential Smoothing and Forward Averaging
Exponential smoothing is another prominent forecasting technique. Unlike forward averaging, which averages future data points (or forecasts thereof), exponential smoothing assigns exponentially decreasing weights to past data points. This means that more recent observations are given greater importance in the forecast. While seemingly disparate, these two methods can be combined. For instance, one could use an exponential smoothing model to generate forecasts of future data points, and then use those forecasts as input for a forward averaging calculation. This hybrid approach can leverage the strengths of both methodologies—the responsiveness of exponential smoothing to recent changes and the forward-looking perspective of forward averaging.
Further Analysis: Deep Dive into Lead Time Optimization
Optimal lead time selection is paramount to effective forward averaging. A lead time that’s too short may fail to capture longer-term trends, resulting in noisy and inaccurate forecasts. Conversely, a lead time that is too long can introduce excessive lag and render the forecast irrelevant to near-term decision-making.
Several techniques can aid in optimizing lead time:
-
Cross-Validation: This method involves dividing the available data into training and validation sets. Different lead times are tested on the training set, and their performance is evaluated on the validation set. The lead time yielding the lowest forecast error is selected.
-
Information Criteria: Metrics like AIC (Akaike Information Criterion) and BIC (Bayesian Information Criterion) can be used to assess the goodness of fit of forward averaging models with different lead times. These criteria balance model fit with complexity, penalizing overly complex models with many parameters (long lead times).
-
Simulation Studies: Monte Carlo simulations can be used to test the robustness of the forward averaging method under various conditions and lead times. This allows for the identification of lead times that are least sensitive to variations in data characteristics.
Frequently Asked Questions about Forward Averaging
-
Q: What is the main difference between forward averaging and backward averaging (like moving average)? A: Forward averaging uses future data (or predictions of future data) to forecast a future value, while backward averaging uses past data.
-
Q: Can forward averaging handle seasonal data? A: Yes, but the lead time must be carefully chosen to account for the seasonal patterns. Weighted forward averaging can also be used to assign different weights to data points within the seasonal cycle.
-
Q: What are the limitations of using forecasts as input for forward averaging? A: The accuracy of the forward average is directly dependent on the accuracy of the input forecasts. Errors in the input forecasts will propagate through to the final forecast.
-
Q: How can I implement forward averaging in programming languages like R or Python? A: Simple forward averaging can be implemented using basic arithmetic functions. More sophisticated weighted forward averaging may require using matrix operations or specialized libraries.
-
Q: Is forward averaging better than other forecasting methods? A: There's no universally "better" method. The optimal choice depends on the specific dataset, forecasting horizon, and the nature of the time series.
-
Q: How can I deal with missing data in forward averaging? A: Missing data can significantly impact the accuracy. Imputation techniques, such as linear interpolation or more advanced methods, can be used to fill in the missing values before applying forward averaging.
Practical Tips for Maximizing the Benefits of Forward Averaging
-
Assess Data Quality: Ensure your data is accurate, clean, and free of significant errors or outliers.
-
Select Appropriate Lead Time: Utilize cross-validation or information criteria to find the optimal lead time for your dataset.
-
Consider Weighted Averages: Weighted averaging allows you to incorporate prior knowledge about the importance of different future data points.
-
Use Robust Statistical Measures: Employ median-based averaging to reduce the impact of outliers.
-
Combine with Other Methods: Integrate forward averaging with other forecasting techniques to build a more robust and accurate model.
-
Monitor Forecast Accuracy: Regularly evaluate the performance of your forward averaging model and adjust parameters as needed.
-
Incorporate External Factors: Integrate external information, such as economic indicators or market sentiment, into your forecasts.
-
Understand Limitations: Recognize the limitations of forward averaging, particularly its reliance on accurate future data (or forecasts).
Conclusion: The Future of Forward Averaging
Forward averaging, although seemingly simple in its core concept, represents a powerful and adaptable tool for predictive modeling across diverse applications. By embracing its forward-looking nature and addressing its limitations through careful data analysis, appropriate lead time selection, and judicious combination with other forecasting methodologies, practitioners can unlock significant improvements in forecasting accuracy and decision-making capabilities. Its continued development and integration with advanced analytical techniques, including machine learning, promise even greater potential for innovation and precise future prediction in the years to come. The challenge now lies not only in refining the technique but also in developing more robust and sophisticated methods for obtaining accurate estimations of future data points, the very foundation upon which successful forward averaging hinges.
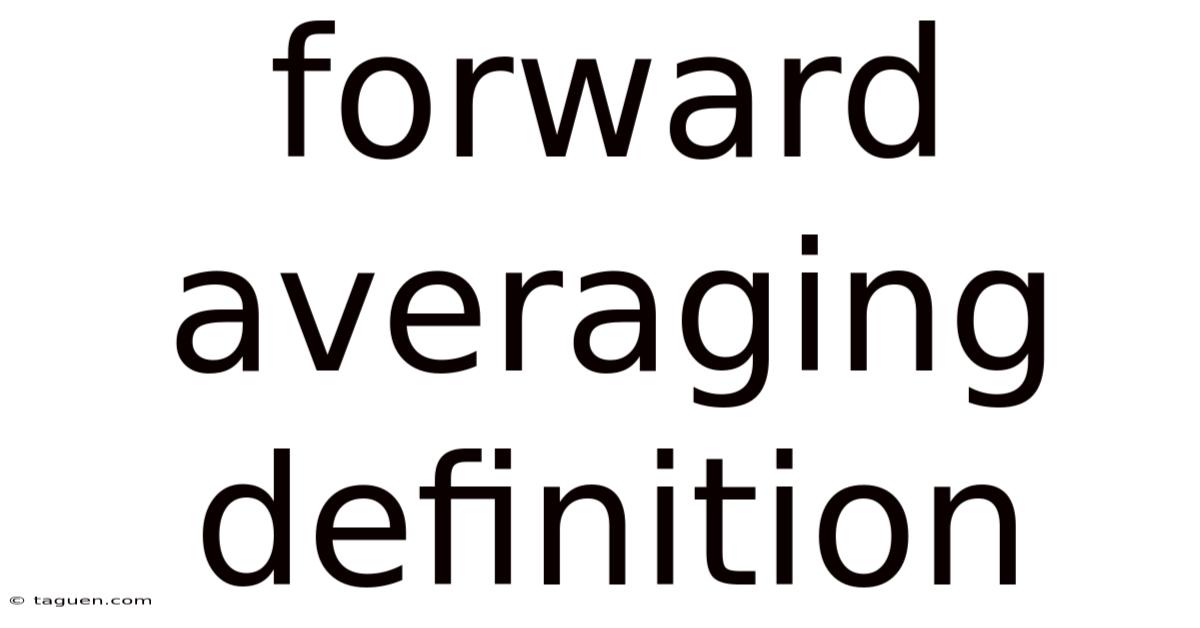
Thank you for visiting our website wich cover about Forward Averaging Definition. We hope the information provided has been useful to you. Feel free to contact us if you have any questions or need further assistance. See you next time and dont miss to bookmark.
Also read the following articles
Article Title | Date |
---|---|
How Much Is A Veterans Pension | Apr 17, 2025 |
Mont Pelerin Society Definition | Apr 17, 2025 |
What Is Securities Trading | Apr 17, 2025 |
How To Apply For Pension Benefits | Apr 17, 2025 |
Forward Rate Agreement Fra Definition Formulas And Example | Apr 17, 2025 |