Econometrics Definition Models And Methods
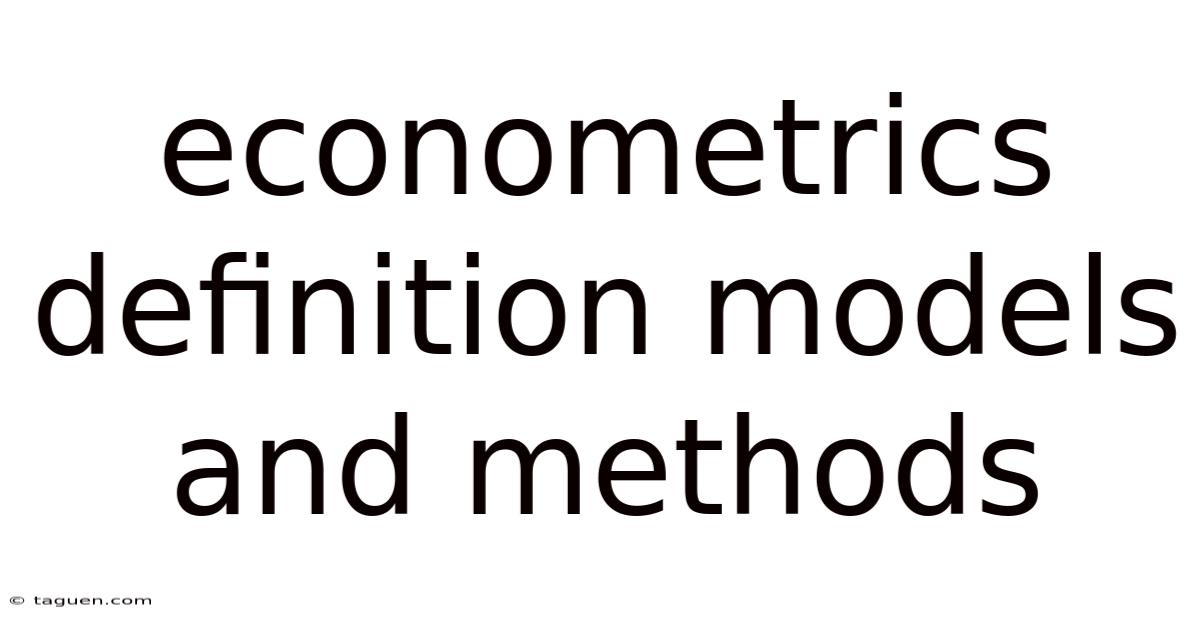
Discover more detailed and exciting information on our website. Click the link below to start your adventure: Visit Best Website meltwatermedia.ca. Don't miss out!
Table of Contents
Unlocking Economic Mysteries: Econometrics, Models, and Methods
What if understanding the complexities of the economy could be simplified through the power of data analysis? Econometrics, a powerful blend of economic theory, mathematical modeling, and statistical inference, offers precisely that – providing a robust framework for uncovering economic relationships and making data-driven predictions.
Editor’s Note: This article on econometrics, its models, and methods provides a comprehensive overview of this crucial field, incorporating the latest advancements and real-world applications. Updated [Date of Publication].
Econometrics plays a vital role in modern economics, offering a rigorous approach to testing economic theories and informing policy decisions. It allows economists to move beyond theoretical speculation and delve into the empirical evidence, quantifying relationships between economic variables and forecasting future trends. Understanding econometrics is essential for anyone seeking a deeper understanding of how economies function and how to analyze economic data effectively. Its applications span various fields, including forecasting economic growth, evaluating the effectiveness of government policies, analyzing market behavior, and understanding the impact of technological advancements.
This article delves into the core aspects of econometrics, examining its definition, various models employed, and the statistical methods used for analysis. We will explore its real-world applications, the challenges involved, and its potential for future advancements. Backed by expert insights and data-driven research, it provides a comprehensive resource for students, researchers, and professionals interested in economics and data analysis.
Key Takeaways:
Key Aspect | Description |
---|---|
Definition of Econometrics | The application of statistical methods to economic data to test economic theories and make predictions. |
Econometric Models | Various models (linear regression, time series, panel data) used to represent economic relationships. |
Estimation Methods | Techniques (OLS, GLS, Maximum Likelihood) used to estimate the parameters of econometric models. |
Model Diagnostics | Methods to assess the validity and reliability of the estimated econometric model (e.g., R-squared, t-tests). |
Real-World Applications | Forecasting, policy evaluation, market analysis, risk management. |
Challenges and Limitations | Data limitations, model misspecification, endogeneity. |
With a strong understanding of its relevance, let's explore econometrics further, uncovering its applications, challenges, and future implications.
Definition and Core Concepts
Econometrics is fundamentally the application of statistical and mathematical methods to economic data. It bridges the gap between theoretical economic models and real-world observations, allowing economists to test hypotheses, estimate relationships between variables, and make predictions. This involves:
-
Formulating economic models: These models translate economic theories into mathematical equations, specifying relationships between variables. For example, a simple model might posit a relationship between consumer spending (dependent variable) and disposable income (independent variable).
-
Collecting and preparing data: Econometric analysis relies on relevant and reliable data. This might involve using existing datasets from government agencies, international organizations, or private firms, or collecting original data through surveys or experiments. Data cleaning and preparation are crucial steps, addressing issues like missing values and outliers.
-
Estimating model parameters: Statistical techniques are employed to estimate the unknown parameters in the economic models. This involves using sample data to find the best fit for the model, representing the strength and direction of the relationships between variables.
-
Testing hypotheses and making inferences: Econometric analysis goes beyond simply estimating parameters; it involves rigorously testing hypotheses about the relationships between variables. This is done through hypothesis testing and confidence intervals, allowing economists to make statistically sound inferences about the economy.
-
Forecasting and prediction: Once a model is estimated and validated, it can be used to forecast future values of economic variables based on predicted values of the independent variables.
Applications Across Industries
The applications of econometrics are incredibly diverse and span various industries and fields:
-
Macroeconomics: Econometric models are used to analyze macroeconomic phenomena like inflation, unemployment, economic growth, and interest rates. These models can inform policy decisions by governments and central banks.
-
Microeconomics: At the micro level, econometrics helps to understand consumer behavior, firm decision-making, and market structures. This can be applied to areas like pricing strategies, marketing analysis, and investment decisions.
-
Finance: Econometrics is widely used in finance to model asset prices, risk management, portfolio optimization, and forecasting financial markets. Time series analysis plays a crucial role here.
-
Labor Economics: Econometric techniques are used to analyze labor market dynamics, such as wage determination, unemployment, and the impact of labor market policies.
-
Environmental Economics: Econometrics is applied to evaluate the economic costs and benefits of environmental policies, such as carbon taxes and regulations.
-
Health Economics: It helps to evaluate the effectiveness of health interventions, the impact of health insurance, and the determinants of health outcomes.
Econometric Models: A Closer Look
Several econometric models are commonly used, each suited to different data structures and research questions:
-
Linear Regression Models: These are the most fundamental models, assuming a linear relationship between the dependent and independent variables. Ordinary Least Squares (OLS) is the most common estimation method. Variations include multiple linear regression (with multiple independent variables) and weighted least squares (accounting for heteroscedasticity).
-
Time Series Models: These models are used to analyze data collected over time. They account for the temporal dependence in the data, often using techniques like autoregressive (AR) models, moving average (MA) models, and autoregressive integrated moving average (ARIMA) models. These models are crucial for forecasting economic variables.
-
Panel Data Models: These models analyze data that combines both time series and cross-sectional dimensions (e.g., observations on multiple individuals or firms over time). They are particularly useful for controlling for unobserved individual effects and analyzing dynamic relationships. Fixed effects and random effects models are common approaches.
-
Simultaneous Equations Models: These models address situations where multiple dependent variables are simultaneously determined. They explicitly acknowledge the interdependence between variables, using techniques like two-stage least squares (2SLS) to handle endogeneity.
-
Nonlinear Models: When the relationship between variables is not linear, nonlinear models are used. These models can be more complex to estimate, often requiring numerical optimization techniques. Examples include logit and probit models for binary dependent variables.
Estimation Methods and Model Diagnostics
Once an econometric model is specified, estimation methods are used to determine the parameters of the model. The most common method is Ordinary Least Squares (OLS), which minimizes the sum of squared differences between the observed and predicted values. Other methods include:
-
Generalized Least Squares (GLS): Used when the error terms in the model are correlated or have unequal variances (heteroscedasticity).
-
Maximum Likelihood Estimation (MLE): A more general method applicable to a wider range of models, including nonlinear models.
After estimating a model, diagnostic checks are crucial to assess its validity and reliability. These include:
-
R-squared: Measures the goodness of fit, indicating the proportion of variation in the dependent variable explained by the model.
-
t-tests and F-tests: Used to test the statistical significance of individual coefficients and the overall model.
-
Residual analysis: Examining the residuals (the differences between observed and predicted values) to check for patterns or violations of model assumptions, such as heteroscedasticity, autocorrelation, and normality.
Challenges and Limitations
Despite its power, econometrics faces several challenges:
-
Data limitations: The quality and availability of economic data can significantly impact the reliability of econometric results. Data may be incomplete, inaccurate, or subject to measurement error.
-
Model misspecification: Choosing the wrong model can lead to biased and inconsistent estimates. The selection of variables, functional form, and error structure are crucial considerations.
-
Endogeneity: This arises when an independent variable is correlated with the error term, leading to biased estimates. Instrumental variables (IV) techniques are often used to address endogeneity.
-
Causality vs. Correlation: Econometrics primarily deals with correlations between variables. Establishing causality requires careful consideration of confounding factors and experimental designs.
The Relationship Between Causality and Econometric Models
Establishing causality is a central challenge in econometrics. While econometric models can reveal correlations between variables, demonstrating that one variable causes a change in another requires careful consideration. Spurious correlations can easily arise, where two variables appear related but have no actual causal link.
Roles and Real-World Examples: Consider the relationship between ice cream sales and crime rates. Both tend to increase during summer, but this doesn't imply that ice cream sales cause crime. The underlying cause is the warmer weather, which affects both variables.
Risks and Mitigations: Failing to account for confounding variables (like weather in the ice cream/crime example) leads to misleading conclusions. Techniques like instrumental variables, difference-in-differences, and randomized controlled trials help mitigate this risk, allowing for more robust causal inferences.
Impact and Implications: Incorrectly inferring causality can have significant consequences, leading to ineffective policies or misguided business decisions. Rigorous econometric analysis is essential to avoid such pitfalls.
Conclusion
Econometrics is an indispensable tool for understanding and analyzing economic phenomena. By combining economic theory with statistical methods, it allows researchers and policymakers to test hypotheses, make predictions, and inform decision-making. While challenges remain, ongoing advancements in econometric methods and data availability continue to enhance its power and applicability across a wide range of economic questions. The ability to rigorously analyze economic data is crucial for navigating the complexities of modern economies and shaping sound economic policies.
Further Analysis: Deep Dive into Endogeneity
Endogeneity, a significant challenge in econometrics, arises when an independent variable is correlated with the error term in a regression model. This correlation violates the fundamental assumption of OLS regression, leading to biased and inconsistent estimates. The correlation can stem from several sources:
-
Omitted variable bias: If a relevant variable is omitted from the model, it might be captured in the error term, leading to correlation with an included independent variable.
-
Simultaneity bias: This occurs in simultaneous equations models where the dependent and independent variables influence each other reciprocally.
-
Measurement error: Errors in measuring independent variables can lead to correlation with the error term.
Cause-and-Effect Relationships: Endogeneity distorts the estimated relationship between the independent and dependent variables, making it difficult to infer causal effects. The bias can be positive or negative, depending on the direction of the correlation between the independent variable and the error term.
Significance and Real-World Applications: Ignoring endogeneity can lead to inaccurate conclusions about the effects of policies or economic interventions. For example, in evaluating the effect of education on wages, endogeneity can arise if individuals with higher inherent ability are more likely to pursue higher education, leading to an overestimation of the causal effect of education on wages.
Mitigation Strategies: Several techniques are used to address endogeneity:
-
Instrumental variables (IV): This involves finding an instrument—a variable correlated with the endogenous variable but not directly with the error term—to obtain consistent estimates.
-
Two-stage least squares (2SLS): A common method for estimating models with endogenous variables, using IV techniques.
-
Panel data methods: Fixed effects models can help control for unobserved individual effects that might be correlated with independent variables.
Frequently Asked Questions (FAQs)
Q1: What is the difference between econometrics and statistics?
A1: Statistics is a broader field encompassing the collection, analysis, interpretation, presentation, and organization of data. Econometrics specifically applies statistical methods to economic data to test economic theories and make predictions.
Q2: What software is commonly used for econometric analysis?
A2: Popular software packages include Stata, R, EViews, and SAS. These offer a wide range of econometric tools and functionalities.
Q3: How can I improve the accuracy of my econometric models?
A3: Use appropriate model specifications, address potential endogeneity issues, carefully consider data quality and limitations, and perform thorough diagnostic checks.
Q4: What are the limitations of econometric models?
A4: Models are simplifications of reality and might not capture all relevant factors. Data limitations, model misspecification, and endogeneity can affect accuracy.
Q5: Can econometrics be used to predict the future with certainty?
A5: No, econometric models provide probabilistic forecasts, not certainties. Uncertainty arises from data limitations, model assumptions, and inherent randomness in economic systems.
Q6: How can I learn more about econometrics?
A6: Start with introductory textbooks and online resources. Consider taking econometrics courses to develop a strong foundation in the field.
Practical Tips for Maximizing the Benefits of Econometrics
-
Clearly define your research question: Ensure you have a specific and well-defined question to guide your econometric analysis.
-
Gather high-quality data: The accuracy of your results depends heavily on data quality. Clean and prepare your data meticulously.
-
Choose the appropriate econometric model: Select a model that aligns with your data structure, research question, and theoretical framework.
-
Assess model assumptions: Check for violations of model assumptions (e.g., heteroscedasticity, autocorrelation) and address them appropriately.
-
Perform robustness checks: Test your results' sensitivity to different model specifications and estimation techniques.
-
Interpret results cautiously: Avoid over-interpreting your findings. Acknowledge limitations and potential biases.
-
Communicate your results clearly: Present your findings concisely and accurately, avoiding overly technical jargon.
-
Stay updated on advancements: Econometrics is a constantly evolving field. Keep abreast of the latest methods and techniques.
End Note
With its transformative potential, econometrics is shaping the future of economic analysis across various sectors. By embracing its principles and addressing its challenges, researchers, policymakers, and businesses can unlock new opportunities for informed decision-making and enhanced understanding of the economic world. The continued development and application of econometric methods promise to further refine our ability to unravel the complexities of economic systems and make more accurate and reliable predictions.
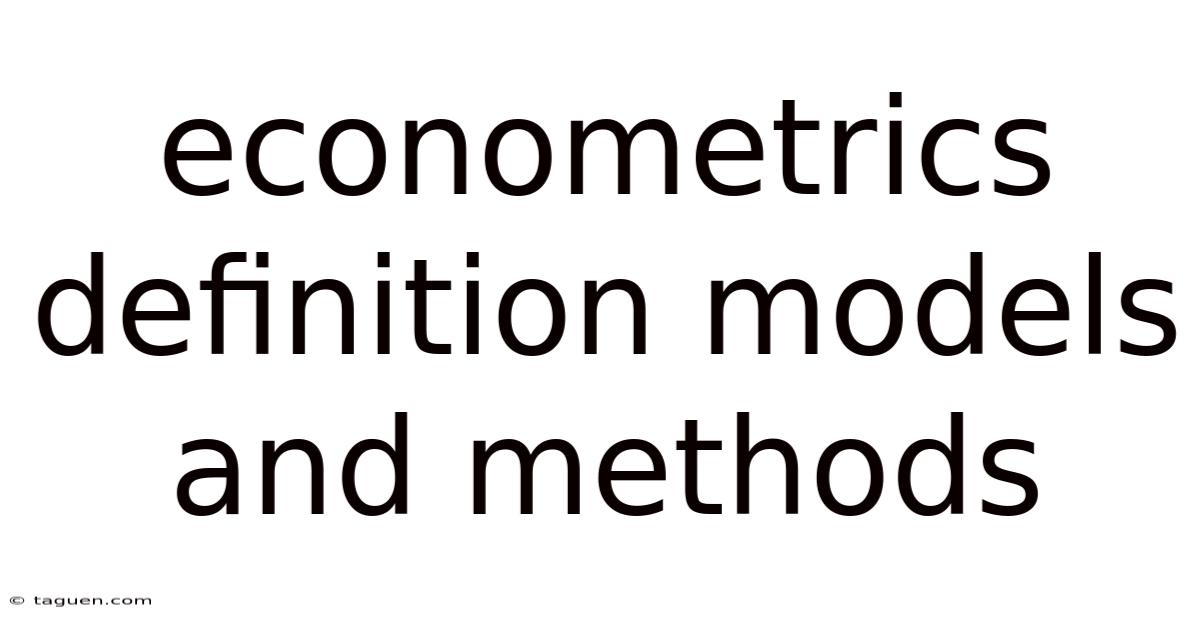
Thank you for visiting our website wich cover about Econometrics Definition Models And Methods. We hope the information provided has been useful to you. Feel free to contact us if you have any questions or need further assistance. See you next time and dont miss to bookmark.
Also read the following articles
Article Title | Date |
---|---|
What Insurance Covers Weight Loss Surgery | Mar 08, 2025 |
Why Should You Purchase Insurance | Mar 08, 2025 |
Effective Duration Definition Formula Example | Mar 08, 2025 |
How Much Is Clindamycin At Walmart Without Insurance | Mar 08, 2025 |
What Happens To An Insurance Policy When The Owner Dies | Mar 08, 2025 |